Improving decametric vegetation products by exploiting machine learning and routine ground reference measurements: the GROUNDED EO project
crossref(2024)
摘要
Estimates of vegetation biophysical variables, including leaf area index (LAI) and the fraction of absorbed photosynthetically active radiation (FAPAR), are essential for effective agricultural and forest monitoring/management. They also represent a crucial input into crop yield, carbon exchange, and weather and climate system models. Exploiting optical Earth Observation (EO), retrieval algorithms now provide routine decametric (10 m to 100 m) estimates of LAI and FAPAR, but are subject to known biases due to assumptions embedded within the radiative transfer models used in their training. To overcome these limitations, retrieval approaches might be based on real EO data and contemporaneous ground reference observations. Historically, however, such ground reference observations have been limited in quantity and suffered from inconsistencies and unquantified measurement uncertainties.Recently, advances in novel automated field instrumentation, standardised processing, and routine data collection by environmental monitoring networks (including the National Ecological Observatory Network (NEON), Integrated Carbon Observation System (ICOS), and Terrestrial Ecosystem Research Network (TERN), amongst others) have led to substantial improvements in ground reference data spatiotemporal coverage and consistency. In parallel, cutting-edge machine learning approaches such as Gaussian processes have dramatically reduced the number of required training samples (i.e. thousands as opposed to hundreds of thousands). These approaches also enable the explicit treatment of measurement uncertainties. Through work under the Fiducial Reference Measurements for Vegetation (FRM4VEG) programme and other similar initiatives, methods to quantify ground reference measurement uncertainties in a traceable manner have recently become available.As a result of these advances, for the first time, the development of operational retrieval approaches based on real observations is a realistic possibility. Funded by the European Space Agency’s Living Planet Fellowship programme, the Ground Reference Observations Underlying Novel Decametric Vegetation Data Products from Earth Observation (GROUNDED EO) project is adopting such a data-driven strategy. To date, work has primarily focused on developing a harmonised ground reference database. Raw digital hemispherical photography (DHP) and digital cover photography (DCP) has been obtained from 47 NEON sites in the United States, 20 ICOS sites in Europe, and 10 TERN sites in Australia. This have been processed to derive plant area index (PAI) and the fraction of intercepted photosynthetically active radiation (FIPAR), with associated uncertainties.To make use of the harmonised ground reference database for biophysical variable retrieval, a matchup database containing contemporaneous Sentinel-2 Level 2A surface reflectance observations is being assembled. Because the Collection 1 reprocessing is not yet complete, Level 2A products prior to December 2018 (or March 2018 in Europe) are not available, severely limiting the number of matchups. To address this, work is underway to process all associated Level 1C products acquired over GROUNDED EO sites to Level 2A using Sen2Cor. Once complete, work will commence on the development of a data-driven biophysical processor. Gaussian processes, which have already proven efficient for radiative transfer model based biophysical variable retrieval, will be utilised for this. Active learning will be adopted to select only the most informative training samples, reducing the size of the training dataset and increasing computational efficiency and accuracy.
更多查看译文
AI 理解论文
溯源树
样例
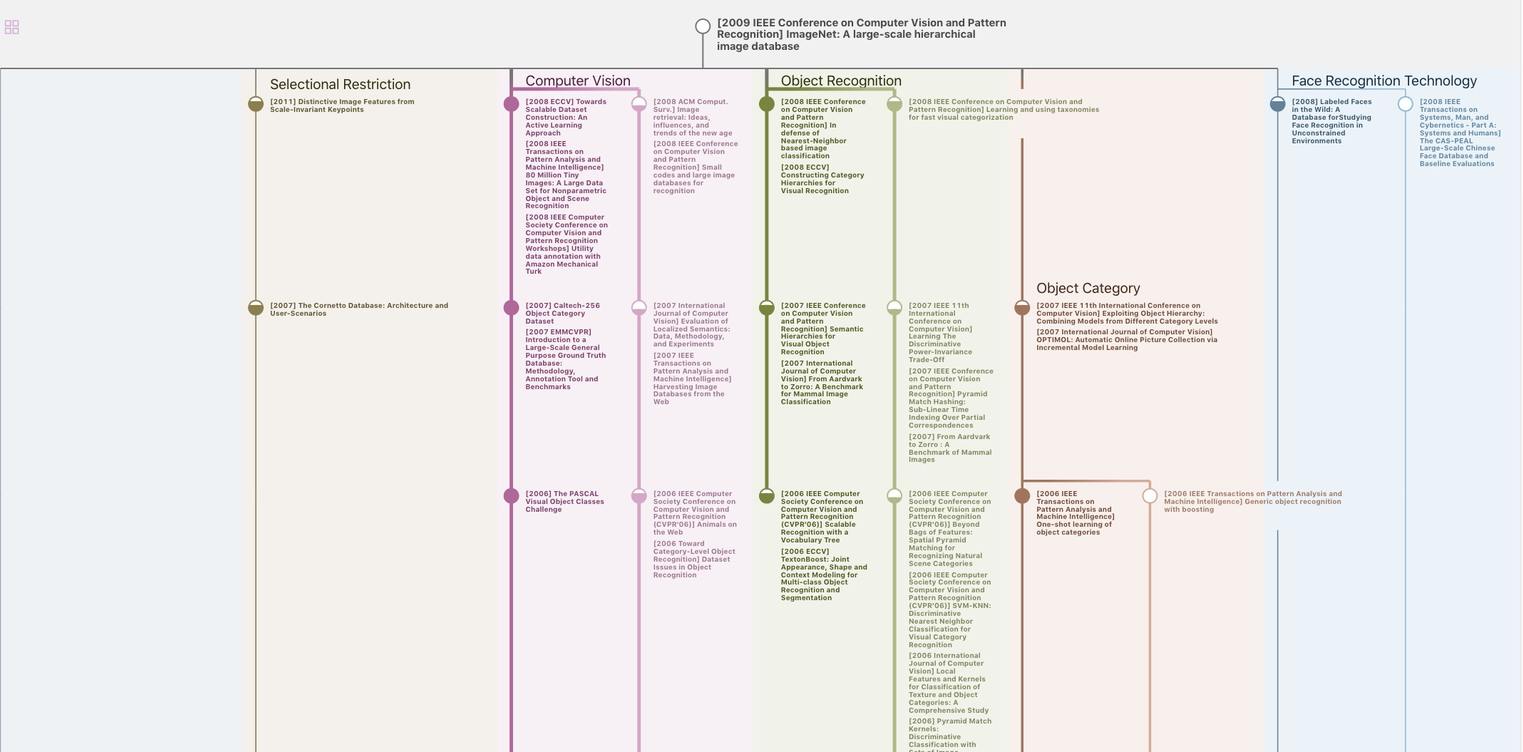
生成溯源树,研究论文发展脉络
Chat Paper
正在生成论文摘要