Singular Vector Decomposition (SVD) of satellite datasets: relation between cloud properties, radiative fluxes and climate indices.
crossref(2024)
摘要
We describe a technique, singular vector decomposition (SVD), that can identify the spatial patterns that best describe the temporal variability of a global satellite dataset. These patterns, and their temporal evolution are then correlated with established climate indices. We apply this technique to datasets of cloud properties and radiative fluxes over three decades ((A)ATSR/SLSTR, MODIS, IASI and CERES), but it can be more generically used to extract the pattern of variability of any regular gridded dataset such as different parameters from satellite retrieval and models.Leading singular vector for independent global data sets on both cloud properties (cloud fraction, cloud-top height) and TOA radiative fluxes, from polar orbiting satellites, covering different time periods is strongly correlated with ENSO index.SVD approach can provide incites into the underlying causes of observed changes in a particular dataset and provide a new tool in using global satellite observations in assessing global climate model (GCM) performance.
更多查看译文
AI 理解论文
溯源树
样例
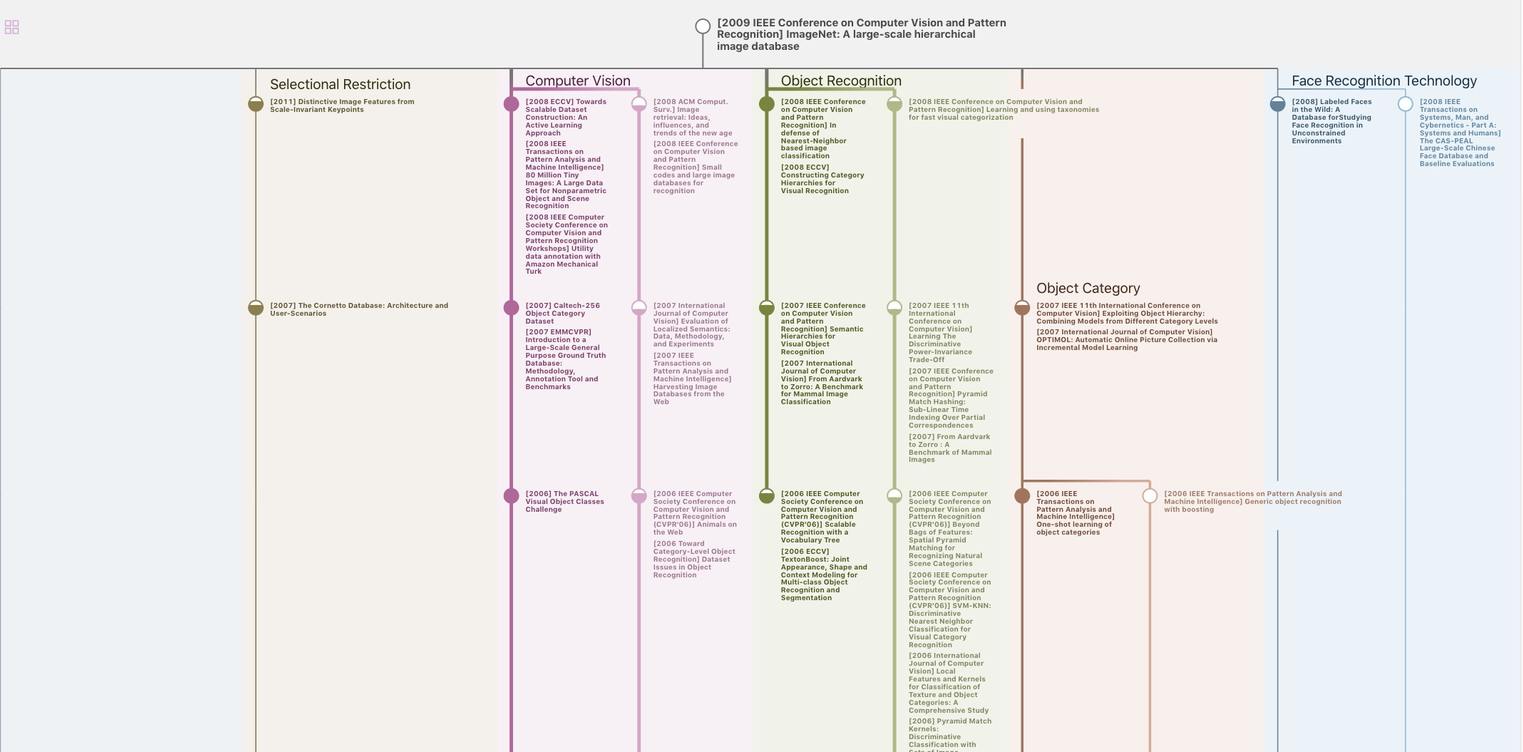
生成溯源树,研究论文发展脉络
Chat Paper
正在生成论文摘要