A new method for disaggregating path-averaged rain rates from commercial microwave links
crossref(2024)
摘要
Commercial microwave links (CMLs) serve as point-to-point radio connections in cellular backhaul and offer a promising way to measure rainfall opportunistically. Raindrops along the CML path attenuate electromagnetic waves, allowing the conversion of this attenuation into path-averaged rain rates. Wide coverage of CML networks, high density in urban areas, and cost-effective operation present clear advantages over traditional rain gauges and radar networks. However, the integrated nature of CML data poses a challenge. When transforming this data into spatially representative rainfall estimates, such as 2D maps, path-integrated rain rates need to be converted into point data and interpolated to a regular two-dimensional Cartesian grid. The most direct method involves reducing each CML observation to a single-point measurement at the path's center, followed by interpolation using techniques like kriging or inverse distance weighted (IDW) interpolation. Yet, past studies indicate that for longer CMLs (several kilometers) and intense localized rain showers, this approach can introduce significant biases and unrealistic rainfall distributions due to the substantial spatial and temporal variability of rainfall. In this contribution, we introduce a new disaggregation method employing random cascades. The method redistributes rainfall amounts along CML paths across progressively smaller scales using a discrete, conservative multiplicative random cascade. Inspired by the EVA (Equal-volume area) cascade developed by Schleiss (2020) for disaggregating spatially intermittent rainfall fields, our approach involves splitting each CML segment into two new segments with different path-lengths but identical path-integrated rainfall. We call this new method CLEAR (CML segments with equal amounts of rain). CLEAR is tested for CML network of 77 CMLs located in Prague, CZ. First, the disaggregation is evaluated using simulated CML observations and, second, CML rain rates derived from real attenuation data. Our findings demonstrate that CLEAR surpasses reconstruction algorithms that reduce CML observations into a single point. It accurately replicates the highly diverse rainfall distributions observed along CMLs, including their intermittency. Moreover, the stochastic nature of the cascade enables the quantification of uncertainty associated with the spatial redistribution of rainfall rates along CMLs. References Schleiss, Marc. “A New Discrete Multiplicative Random Cascade Model for Downscaling Intermittent Rainfall Fields.” Hydrology and Earth System Sciences 24, no. 7 (July 23, 2020): 3699–3723. https://doi.org/10.5194/hess-24-3699-2020.
更多查看译文
AI 理解论文
溯源树
样例
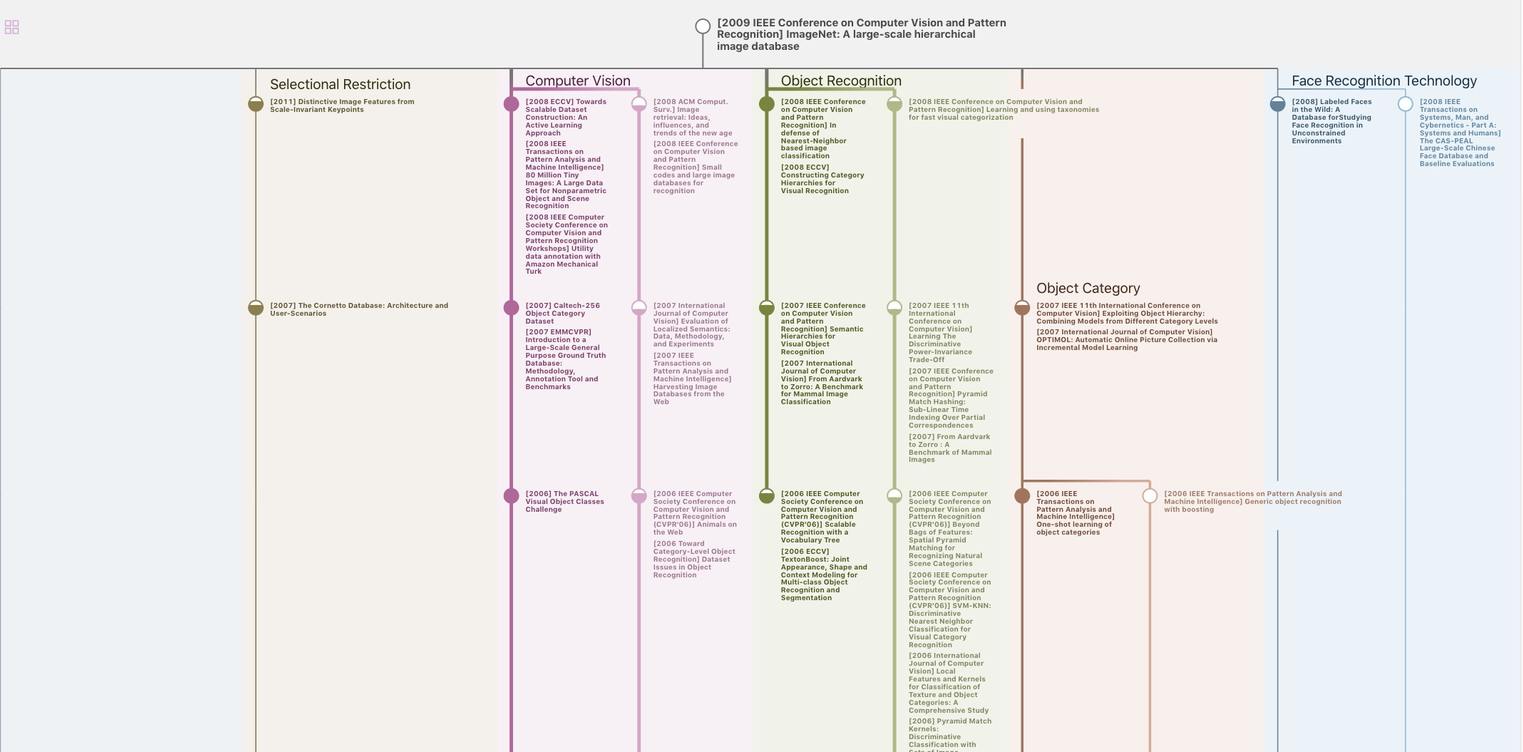
生成溯源树,研究论文发展脉络
Chat Paper
正在生成论文摘要