Evaluating Sentinel-1 volume scattering based snow depth retrievals over NASA SnowEx sites
crossref(2024)
摘要
Synthetic aperture radar will be at the forefront of future advancements in global remote sensing of snow depth and snow water equivalent. Recently, snow depth retrievals using an empirical volume scattering approach with C-band Sentinel-1 (S1) data have been demonstrated over the European Alps and Northern Hemisphere, with the most accurate results obtained in regions with dry, deep (>1.5 m) snowpacks and little vegetation influence. However, these S1-based snow depth retrievals have previously been compared only to point-based measurements or modeled snow depth products. In this study we develop an open-source version of the S1 snow depth retrieval technique and compare the results to spatially-distributed lidar snow depth measurements. The highly accurate and fine resolution lidar datasets were collected during the NASA Snow SnowEx 2020 and 2021 field campaigns at six study sites across the western United States. These regions represent different snow environments and characteristics than the datasets used for comparison in previous investigations. We compare the S1 and lidar snow depths at a range of spatial resolutions and interpret the results within the context of snowpack, vegetation, and terrain characteristics. At 90 m resolution, comparisons between lidar and S1 snow depth retrievals show low to moderate correlations (R = 0.38) and high RMSE (0.98 m) averaged across the study sites, with improved performance at 500 m resolution (R = 0.59, RMSE = 0.69 m). The distribution of S1 and lidar snow depths are more similar in regions of deeper snow, lower forest coverage, higher incidence angles, dry snow, and at coarser spatial resolutions. Our results highlight limitations of the current S1 snow depth algorithm and present opportunities to improve the technique for future snow depth retrievals across varied snow environments.
更多查看译文
AI 理解论文
溯源树
样例
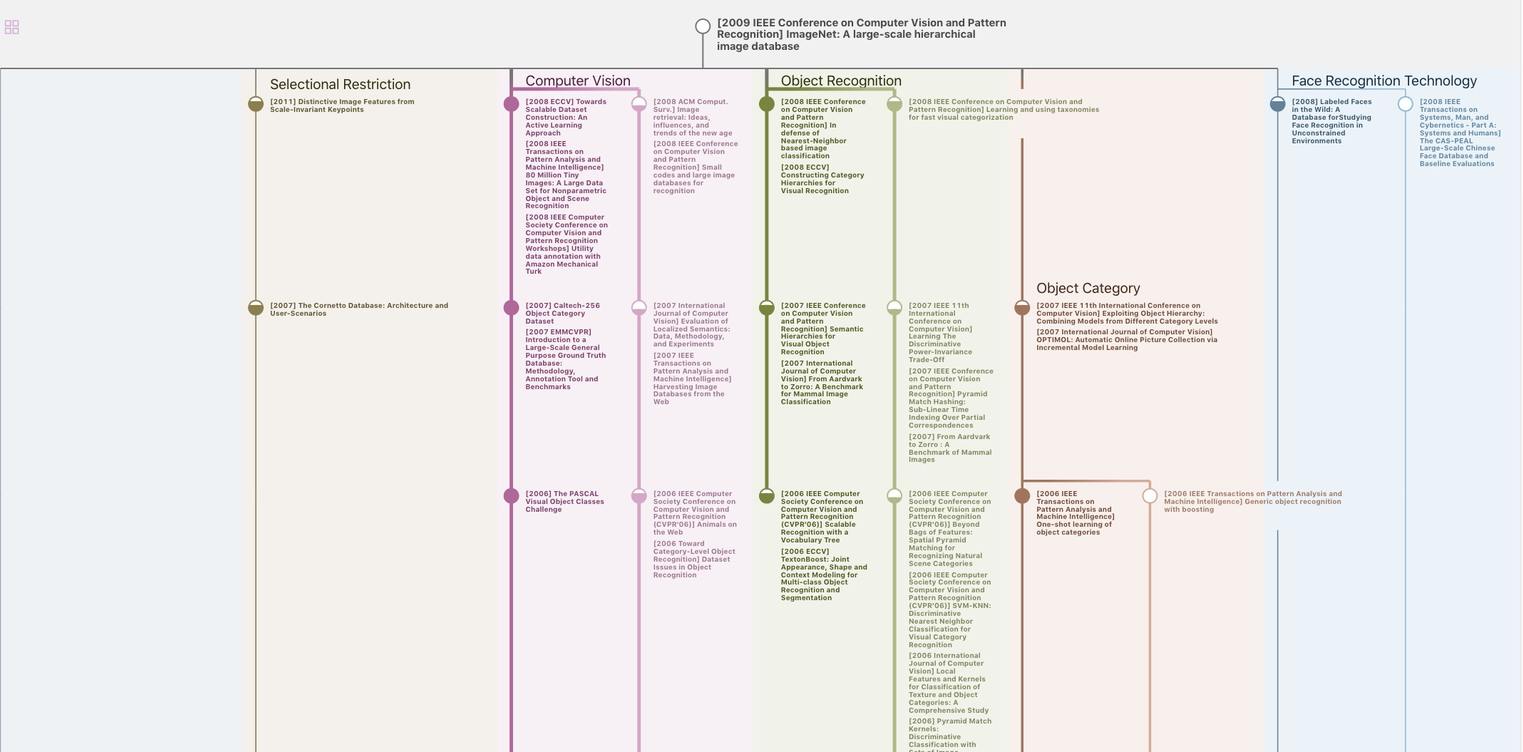
生成溯源树,研究论文发展脉络
Chat Paper
正在生成论文摘要