Toward Data-Driven Urban Canopy Models
crossref(2024)
摘要
Understanding the air flows in urban environments is crucial to mitigating urban heat islands, modeling pollutant dispersion, and other health and safety issues. Cities represent intricate environments, showcasing large-scale disparities from the order of the meter, up to hundreds of meters. Numerical methods are extensively employed to investigate urban areas, encompassing microscale building-resolved simulations (1-10m) and Numerical Weather Predictions (NWP) at larger scales (100m-1km). Microscale simulations are used for high-fidelity analysis. NWP, operating on broader scales, necessitates the parameterization of microscale features like sub-grid turbulence and building drag. Two parametrization methods are utilized: unmeshed, situating the model's first level above the urban canopy, and partially resolved, immersing buildings into the atmosphere. Common unmeshed models use a rough logarithmic law of the wall, characterized by roughness parameters (z0 and d), posing a parameterization challenge for accurate predictions. In the partially resolved approach, layers interact and exchange horizontally averaged quantities. Buildings' effects are parametrized through a drag coefficient and an urban-specific mixing length to model building drag and sub-grid turbulence, respectively. Current models for roughness parameters rely on idealized arrays and morphological metrics. However, challenges emerge as most models encounter difficulties in capturing the intricacies of heterogeneous arrays. Similarly, drag and sub-grid turbulence models are derived from idealized building-resolved simulations, leading to oversimplified parametrizations that assume constant values for the drag coefficient and mixing length. Dispersive momentum fluxes (DMF) arising from spatial averaging are also neglected. Building-resolved simulations across complex, heterogeneous arrays have demonstrated the variability of both drag coefficient and mixing length and that DMF could not be neglected [1]. The intricate interactions between buildings’ layouts and these parameters remain incompletely understood. Data-driven methodologies show promise for urban parametrizations, but the challenge lies in acquiring an extensive dataset for learning tasks. Traditional high-fidelity simulations of urban neighborhoods are computationally expensive. Recent advancements in Lattice Boltzmann solvers, specifically on GPUs, enable cost-effective simulations [2]. The Lattice Boltzmann GPU solver validated on DNS [3], LBMpy, allows the generation of a large dataset of idealized urban arrays with randomized street patterns and heights. Simple learning algorithms are trained with a statistical representation of buildings, incorporating height fluctuation statistics up to the fourth moment, following Jouybari’s methodology [4]. We conduct a comparative analysis with a neural network approach that autonomously processes raw building representations. This study evaluates the effectiveness of Artificial Neural Networks in accurately predicting urban canopy parametrization quantities. The assessment is grounded in two representations of morphologies: the traditional statistical treatment of buildings and an innovative approach where a neural network directly interacts with the raw layout geometry. [1] Sützl, Drag Distribution in Idealized Heterogeneous Urban Environments, BLM, 2021. [2] Lenz, Towards real-time simulation of turbulent air flow over a resolved urban canopy using the cumulant lattice Boltzmann method on a GPGPU. Journal of Wind Engineering, 2019. [3] Coceal. Spatially-averaged flow statistics within a canopy of large bluff bodies: Results from direct numerical simulations. Geophysica, 2008. [4] Jouybari. Data-driven prediction of the equivalent sand-grain height in rough-wall turbulent flows. JFM, 2021.
更多查看译文
AI 理解论文
溯源树
样例
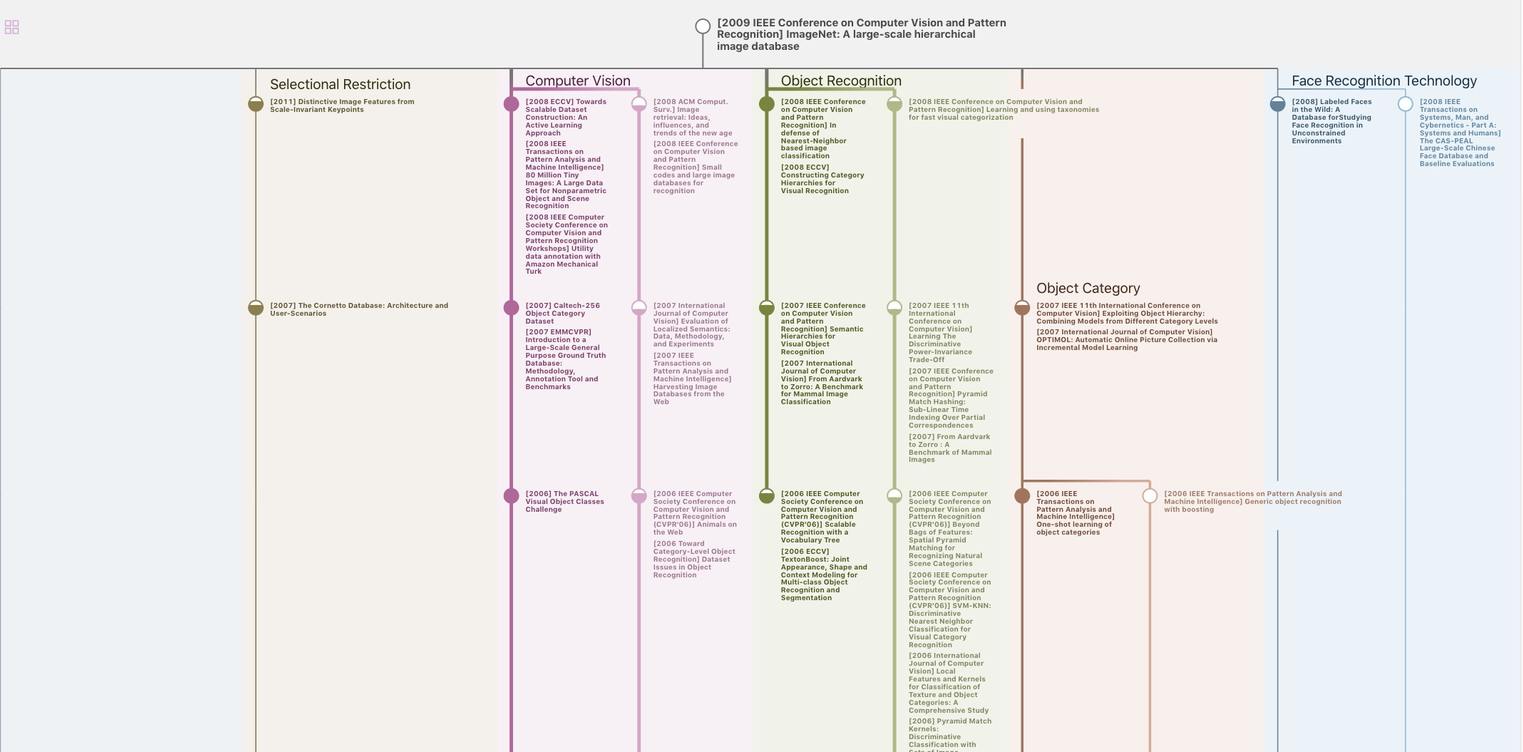
生成溯源树,研究论文发展脉络
Chat Paper
正在生成论文摘要