Lower Bounds on the Noiseless Worst-Case Complexity of Efficient Global Optimization
Journal of Optimization Theory and Applications(2024)
摘要
Efficient global optimization is a widely used method for optimizing expensive black-box functions. In this paper, we study the worst-case oracle complexity of the efficient global optimization problem. In contrast to existing kernel-specific results, we derive a unified lower bound for the oracle complexity of efficient global optimization in terms of the metric entropy of a ball in its corresponding reproducing kernel Hilbert space. Moreover, we show that this lower bound nearly matches the upper bound attained by non-adaptive search algorithms, for the commonly used squared exponential kernel and the Matérn kernel with a large smoothness parameter ν . This matching is up to a replacement of d/2 by d and a logarithmic term logR/ϵ , where d is the dimension of input space, R is the upper bound for the norm of the unknown black-box function, and ϵ is the desired accuracy. That is to say, our lower bound is nearly optimal for these kernels.
更多查看译文
关键词
Efficient global optimization,Worst-case complexity,Reproducing Kernel Hilbert space
AI 理解论文
溯源树
样例
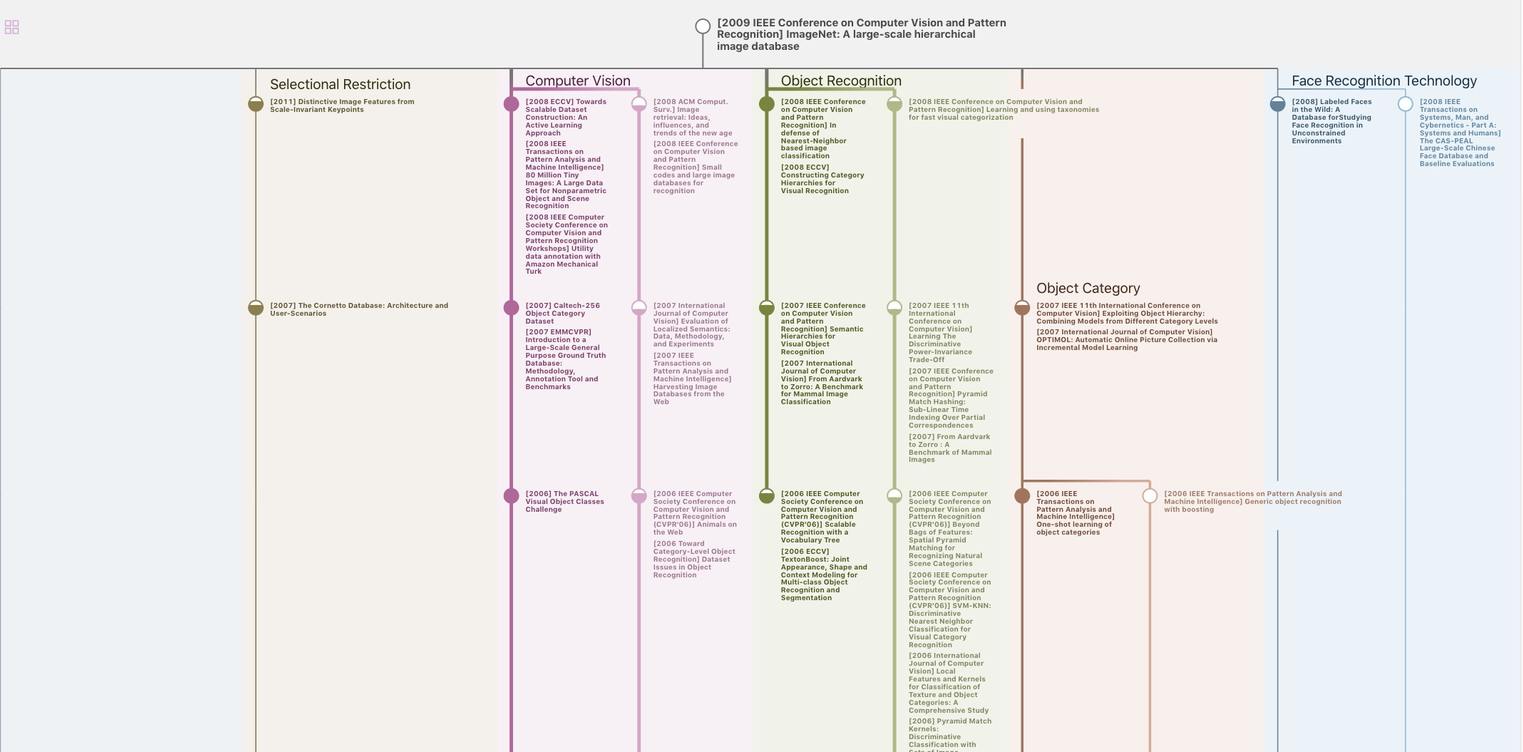
生成溯源树,研究论文发展脉络
Chat Paper
正在生成论文摘要