Accelerating factor Xa inhibitor discovery with a de novo drug design pipeline
Chinese Journal of Chemical Engineering(2024)
摘要
Small-molecule drugs are essential for maintaining human health. The objective of this study is to identify a molecule that can inhibit the Factor Xa protein and be easily procured. An optimization-based de novo drug design framework, DrugCAMD, that integrates a deep learning model with a mixed-integer nonlinear programming model is used for designing drug candidates. Within this framework, a virtual chemical library is specifically tailored to inhibit Factor Xa. To further filter and narrow down the lead compounds from the designed compounds, comprehensive approaches involving molecular docking, binding pose metadynamics (BPMD), binding free energy calculations, and enzyme activity inhibition analysis are utilized. To maximize efficiency in terms of time and resources, molecules for in vitro activity testing are initially selected from commercially available portions of virtual chemical libraries. In vitro studies assessing inhibitor activities have confirmed that the compound EN300-331859 shows potential Factor Xa inhibition, with an IC50 value of 34.57 μmol·L–1. Through in silico docking and BPMD, the most plausible binding pose for the EN300-331859-Factor Xa complex are identified. The estimated binding free energy values correlate well with the results obtained from biological assays. Consequently, EN300-331859 is identified as a novel and effective sub-micromolar inhibitor of Factor Xa.
更多查看译文
关键词
Chemical product design,Mathematical programming method,Deep learning,Binding affinity,Binding free energy
AI 理解论文
溯源树
样例
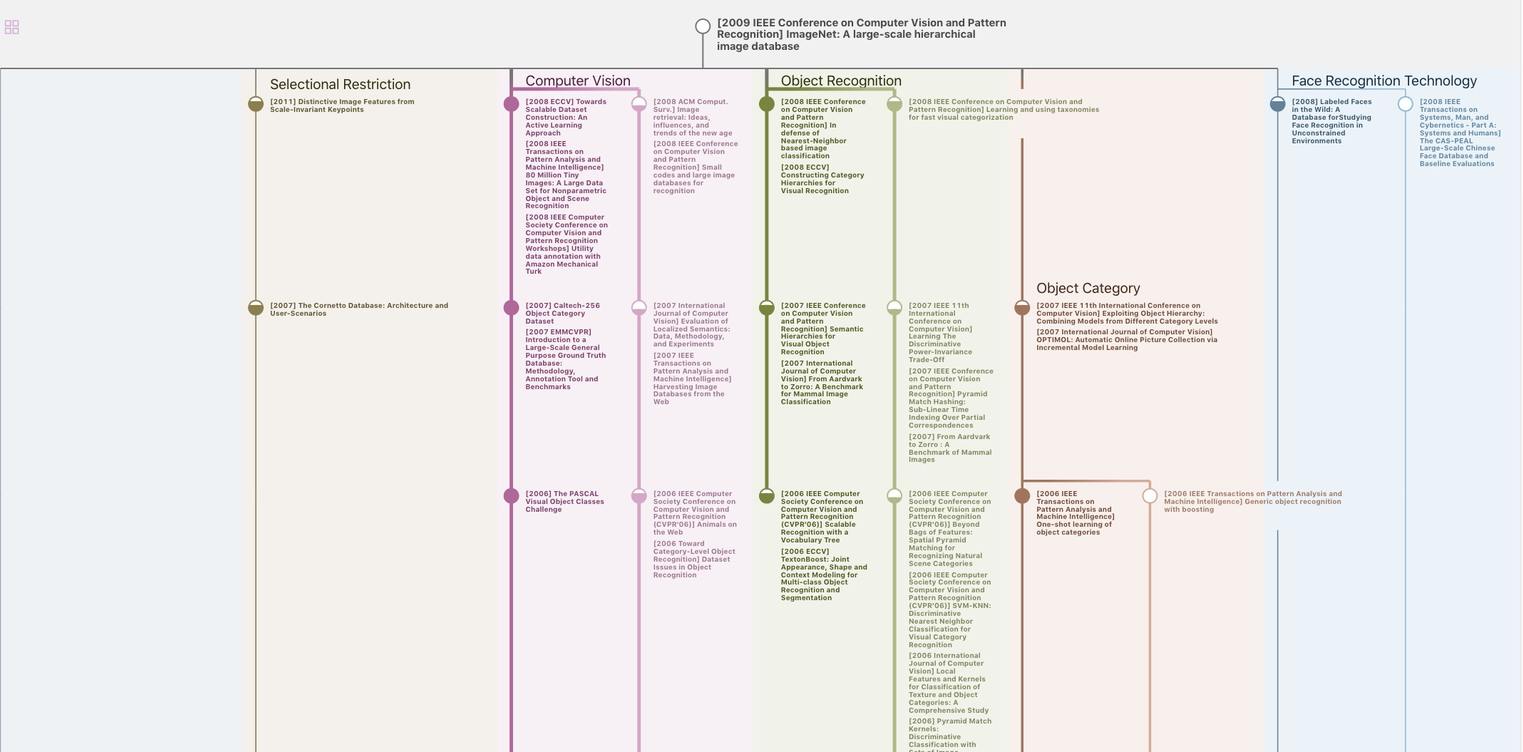
生成溯源树,研究论文发展脉络
Chat Paper
正在生成论文摘要