ARISGAN: Extreme Super-Resolution of Arctic Surface Imagery using Generative Adversarial Networks
crossref(2024)
摘要
This research explores the application of generative artificial intelligence, specifically the novel ARISGAN framework, for generating high-resolution synthetic satellite imagery in the challenging arctic environment. The study addresses the crucial need for realistic and high-resolution surface imagery in the Arctic, vital for applications ranging from satellite retrieval systems to the well-being and safety of Inuit populations relying on detailed surface observations. The ARISGAN framework combines dense block, multireceptive field, and Pix2Pix architecture, showcasing promising results that surpass existing state-of-the-art models across diverse tasks and metrics. Land-based imagery super-resolution exhibits superior metrics in comparison to sea-based imagery across multiple models. This research contributes to the advancement of Earth Observation in polar regions by introducing a framework that combines advanced image processing techniques and a well-designed architecture. The ARISGAN framework's effectiveness in outperforming existing models underscores its potential for generating perceptually valid high-resolution arctic surface imagery. The study concludes with a discussion on identified limitations and proposes avenues for future research, emphasizing the importance of addressing challenges in temporal synchronicity, multi-spectral image analysis, pre-processing, and quality metrics. The findings encourage further refinement of the ARISGAN framework, ultimately advancing the quality and availability of high-resolution satellite imagery in the Arctic.
更多查看译文
AI 理解论文
溯源树
样例
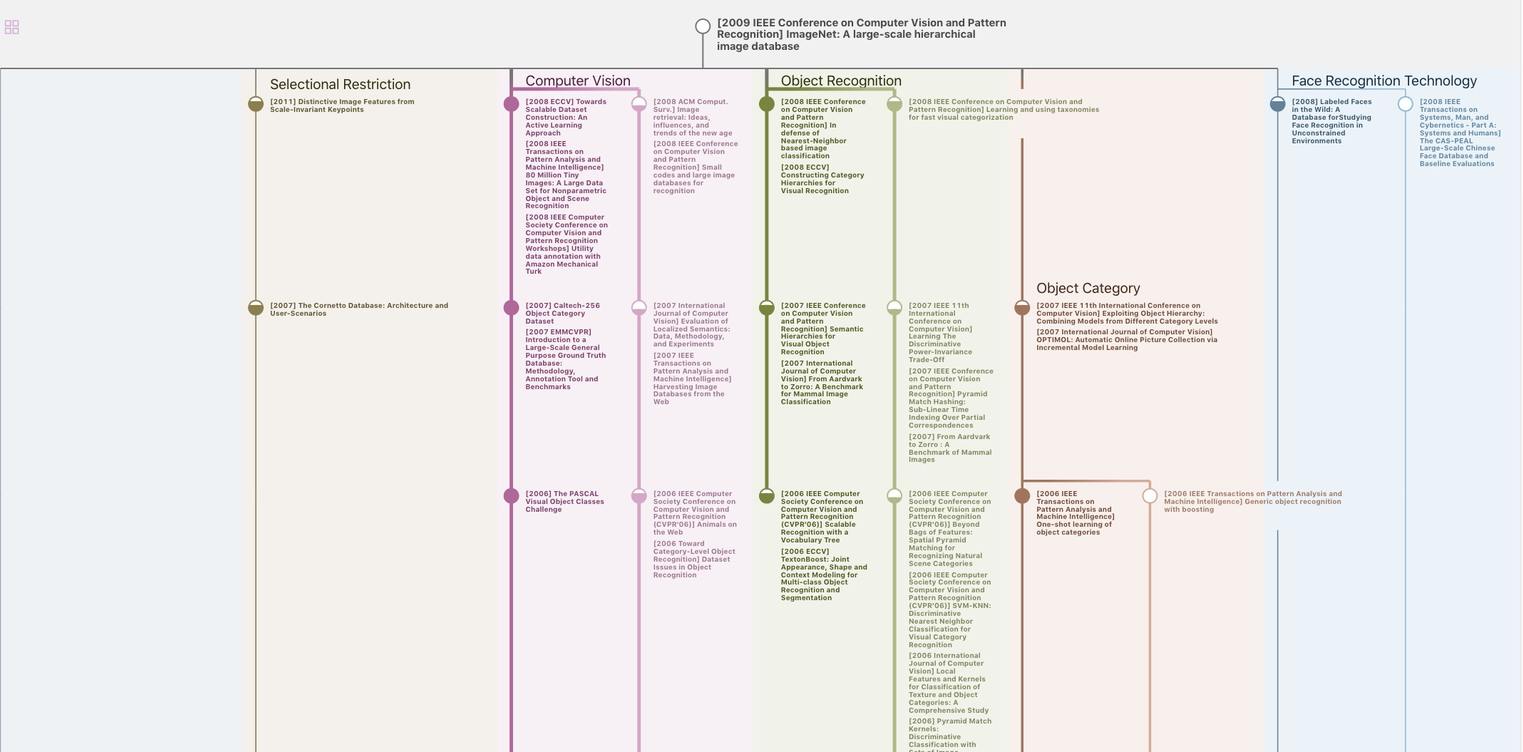
生成溯源树,研究论文发展脉络
Chat Paper
正在生成论文摘要