Optimizing Short-Term Photovoltaic Power Forecasting: A Novel Approach with Gaussian Process Regression and Bayesian Hyperparameter Tuning
PROCESSES(2024)
摘要
The inherent volatility of PV power introduces unpredictability to the power system, necessitating accurate forecasting of power generation. In this study, a machine learning (ML) model based on Gaussian process regression (GPR) for short-term PV power output forecasting is proposed. With its benefits in handling nonlinear relationships, estimating uncertainty, and generating probabilistic forecasts, GPR is an appropriate approach for addressing the problems caused by PV power generation's irregularity. Additionally, Bayesian optimization to identify optimal hyper-parameter combinations for the ML model is utilized. The research leverages solar radiation intensity data collected at 60-min and 30-min intervals over periods of 1 year and 6 months, respectively. Comparative analysis reveals that the data set with 60-min intervals performs slightly better than the 30-min intervals data set. The proposed GPR model, coupled with Bayesian optimization, demonstrates superior performance compared to contemporary ML models and traditional neural network models. This superiority is evident in 98% and 90% improvements in root mean square errors compared to feed-forward neural network and artificial neural network models, respectively. This research contributes to advancing accurate and efficient forecasting methods for PV power output, thereby enhancing the reliability and stability of power systems.
更多查看译文
关键词
Bayesian optimization,PV power forecasting,Gaussian process regression,machine learning,solar radiation intensity
AI 理解论文
溯源树
样例
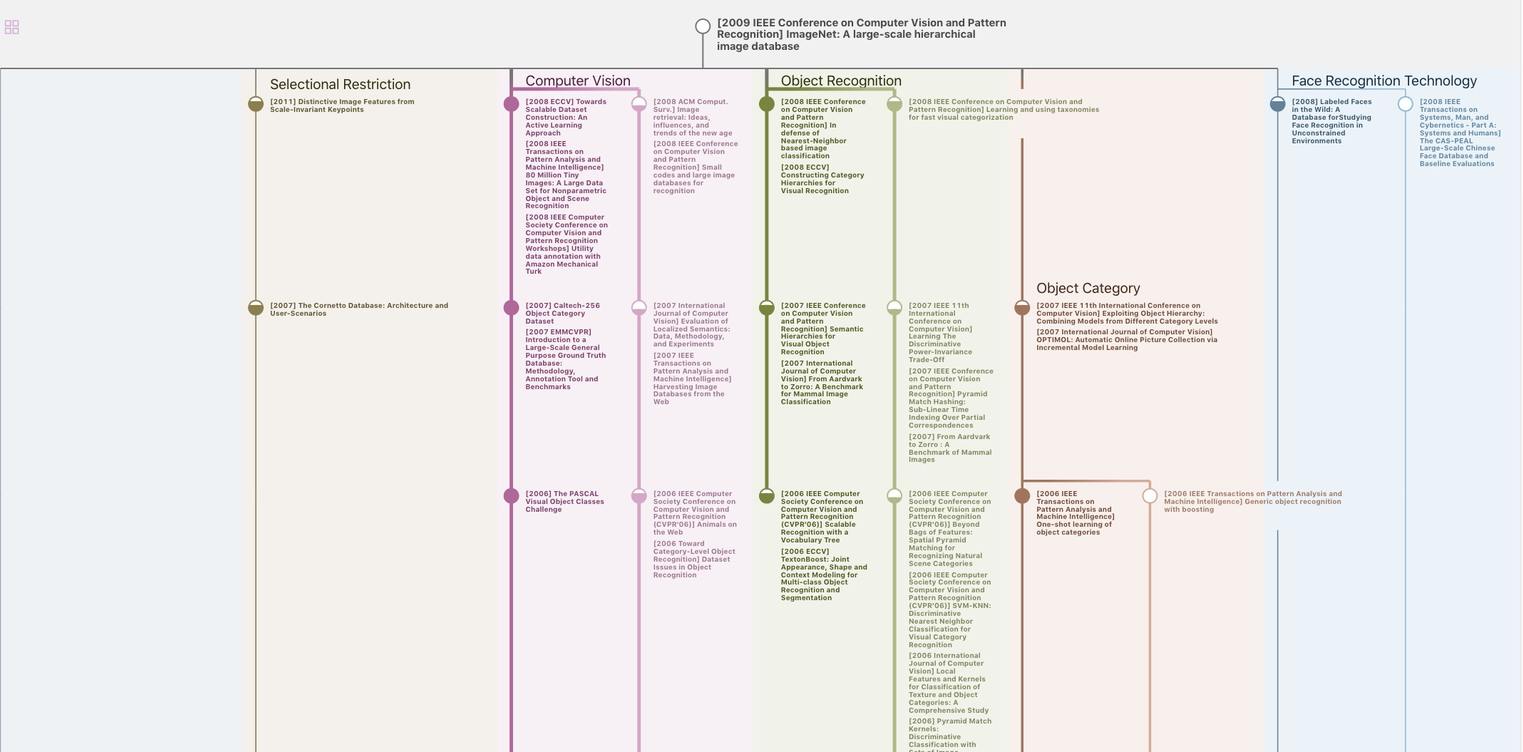
生成溯源树,研究论文发展脉络
Chat Paper
正在生成论文摘要