Towards Improved Water Quality Modelling Using Neural ODE Models
crossref(2024)
摘要
Neural Ordinary Differential Equations (ODEs) fuse neural networks with a mechanistic equation framework. This hybrid structure offers both traceability of model states and processes, as it is typical for physics-based models, and the ability of machine learning to encode new functional relations. Neural ODE models have demonstrated high potential in hydrologic predictions and scientific investigation of the related process in the hydrologic cycle, i.e. tasks of water quantity estimation (Höge et al., 2022). This explicit representation of state variables is key to water quality modelling. There, we typically have several interrelated state variables like nitrate, nitrite, phosphorous, organic matter,… Traditionally, these states are modelled based on mechanistic kinetic rate expressions that are often only rough approximations of the underlying dynamics. At the same time, this domain of water research suffers from data scarcity and therefore solely data-driven methods struggle to provide accurate predictions reliably. We show how to improve predictions of state dynamics and to foster knowledge gain about the processes in such interrelated systems with multiple states using Neural ODEs. Höge, M., Scheidegger, A., Baity-Jesi, M., Albert, C., & Fenicia, F.: Improving hydrologic models for predictions and process understanding using Neural ODEs. Hydrol. Earth Syst. Sci., 26, 5085-5102, https://hess.copernicus.org/articles/26/5085/2022/
更多查看译文
关键词
Water Quality Monitoring,Model Performance,Rainfall-Runoff Modeling,Groundwater Level Forecasting
AI 理解论文
溯源树
样例
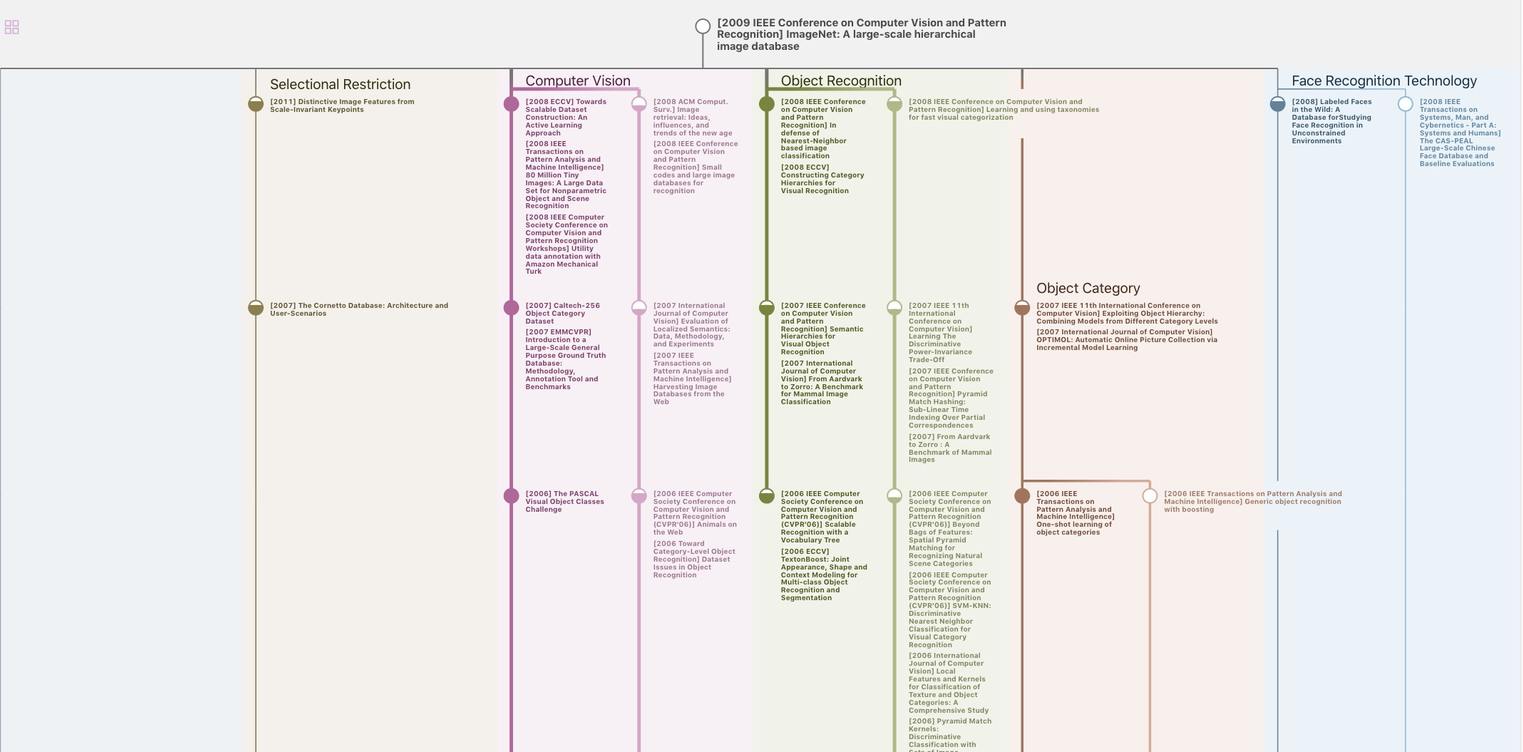
生成溯源树,研究论文发展脉络
Chat Paper
正在生成论文摘要