Efficient-gastro: optimized EfficientNet model for the detection of gastrointestinal disorders using transfer learning and wireless capsule endoscopy images
PEERJ COMPUTER SCIENCE(2024)
摘要
Gastrointestinal diseases cause around two million deaths globally. Wireless capsule endoscopy is a recent advancement in medical imaging, but manual diagnosis is challenging due to the large number of images generated. This has led to research into computer-assisted methodologies for diagnosing these images. Endoscopy produces thousands of frames for each patient, making manual examination difficult, laborious, and error-prone. An automated approach is essential to speed up the diagnosis process, reduce costs, and potentially save lives. This study proposes transfer learning-based efficient deep learning methods for detecting gastrointestinal disorders from multiple modalities, aiming to detect gastrointestinal diseases with superior accuracy and reduce the efforts and costs of medical experts. The Kvasir eight-class dataset was used for the experiment, where endoscopic images were preprocessed and enriched with augmentation techniques. An EfficientNet model was optimized via transfer learning and fine tuning, and the model was compared to the most widely used pre-trained deep learning models. The model's efficacy was tested on another independent endoscopic dataset to prove its robustness and reliability.
更多查看译文
关键词
Deep learning,Gastrointestinal,Digestive endoscopy,Multiclass classification,Augmentation,Capsule endoscopy,Transfer learning
AI 理解论文
溯源树
样例
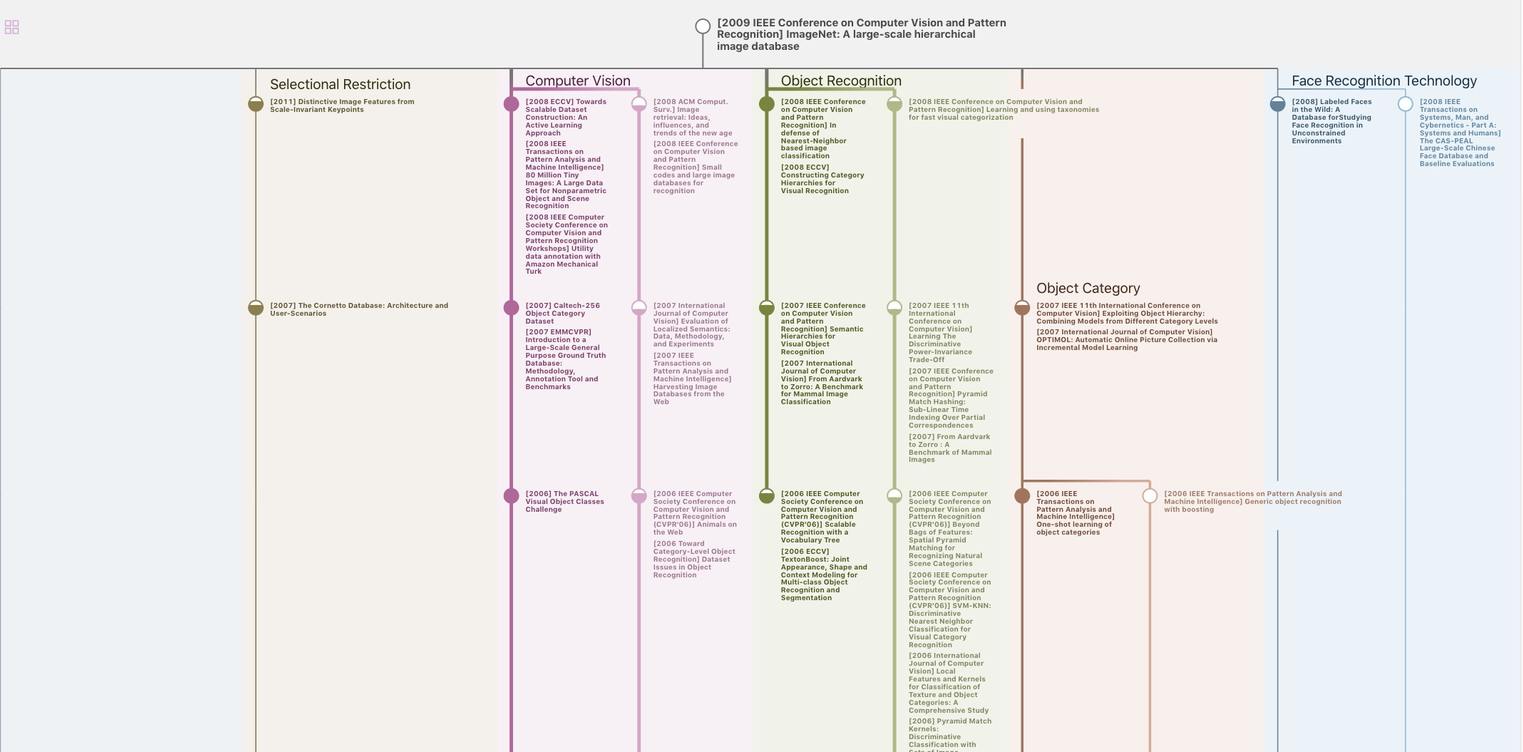
生成溯源树,研究论文发展脉络
Chat Paper
正在生成论文摘要