Stochastic Spatial Weather Generator SPAGETTA: Development and Applications
crossref(2024)
摘要
Stochastic weather generators are one of the most frequently used methodologies for producing input weather series for various process-based models (especially agricultural crop growth models and hydrological rainfall-runoff models) used e.g. in assessing impacts of climate change/variability on weather-dependent processes. SPAGETTA (Dubrovsky et al., 2020, Theor. Appl. Climatol.) is a parametric multi-variable spatial weather generator run commonly (but not only) with daily time step. It is based on applying the spatialisation approach developed by Wilks (1998, J. Hydrol.) to our older single-site weather generator M&Rfi. Similarly to M&Rfi, SPAGETTA is designed for agricultural and hydrological modeling. Until recently, the stress was put on finetuning and validating the generator. Now, when the generator performs reasonably well, it is being used in various experiments. In the first part of the presentation, the main results of the validation tests will be shown, focusing on the ability of the generator to reproduce spatial-temporal variability of multi-site temperature and precipitation series. Concerning the temporal variability, both high-frequency (interdiurnal) and low-frequency (intermonthly and interannual) variability was considered. The performance of the generator was compared with the performance of 19 RCMs taken from the CORDEX database. In the second-part, to demonstrate different applications of the generators, we show results obtained in four experiments: (1) Assessment of separate effects of changes in the WG parameters, which represent the means, variability and lag-0 & lag-1 spatial correlations of temperature and precipitation, on a set of temperature and precipitation indices. The generator parameters were calibrated using the observational E-OBS data from 8 European regions and then modified with the changes (2070-99 vs. 1971-2000) derived from 19 RCM climate simulations (this experiment was already presented in EGU 2023). (2) To show the generator's performance in hydrological modeling, we applied the rainfall–runoff model to the watershed of Dyje river. The model outputs obtained using the synthetic weather series were compared with outputs obtained with the observed weather series (we call this type of experiment “indirect validation of WG”. (3) The generator was used to develop a new test for examining the collective significance of local trends at multiple sites (Huth and Dubrovsky, 2021, J. Clim.). This was made by applying large ensembles of realizations of synthetic multi-site weather series for user-defined lag-0 and lag-1 spatial correlation matrices, (4) The generator was used to assess the statistical significance of climate change scenarios produced by Regional Climate Models. The significance of the RCM-based changes (future vs. baseline) in individual WG parameters is based on comparing their values with the spread of the changes of these parameters based on ensembles of synthetic weather series, i.e. the pairs of synthetic series representing future and present climate; the generator was calibrated by RCM simulations for the corresponding time slices.
更多查看译文
AI 理解论文
溯源树
样例
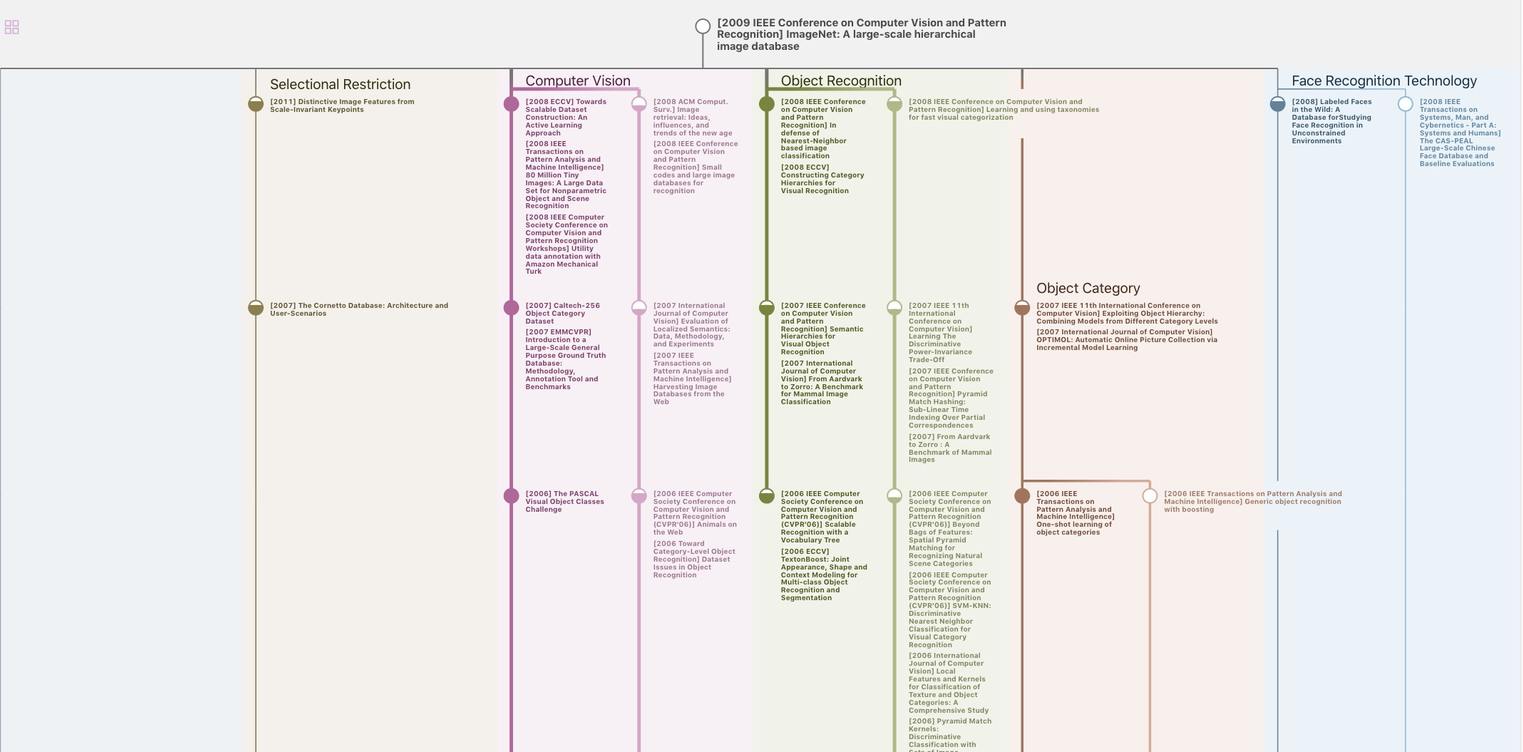
生成溯源树,研究论文发展脉络
Chat Paper
正在生成论文摘要