Evaluating the trade-offs between precision, prediction lead time, transferability, and generalisation in data-driven models for wheat prediction in Morocco
crossref(2024)
摘要
Achieving precision in predicting wheat yields is a critical aspect of agricultural planning, risk mitigation and food security. Here, we present a methodological approach to study the trade-offs between precision, prediction lead time, generalisability, and transferability in wheat yield prediction across arid and semi-arid climates. The methodology leveraged Sentinel-2 satellite imagery for phenology extraction, focusing on derivatives to capture essential model features indicative of wheat growth in distinct climatic zones in Morocco. Model evaluation highlighted varied performances across models and regions, with Support Vector Regression excelling in arid regions (RMSE of 0.23 t.ha-1), while globally, Random Forest consistently outperformed the other models (i.e., Random Forest and Multiple Linear regression). Precision, crucial for capturing evolving growth dynamics, necessitated an increasing number of selected features as the crop progressed and more satellite imagery were available. However, this precision came with trade-offs. Model transferability faced substantial challenges, resulting in notable prediction errors (up to 72.8% decrease in RMSE) when models are transferred between climates. Generalised models revealed lower performances as compared to baseline models in arid climates, emphasizing limitations in leveraging broader training domains.
更多查看译文
AI 理解论文
溯源树
样例
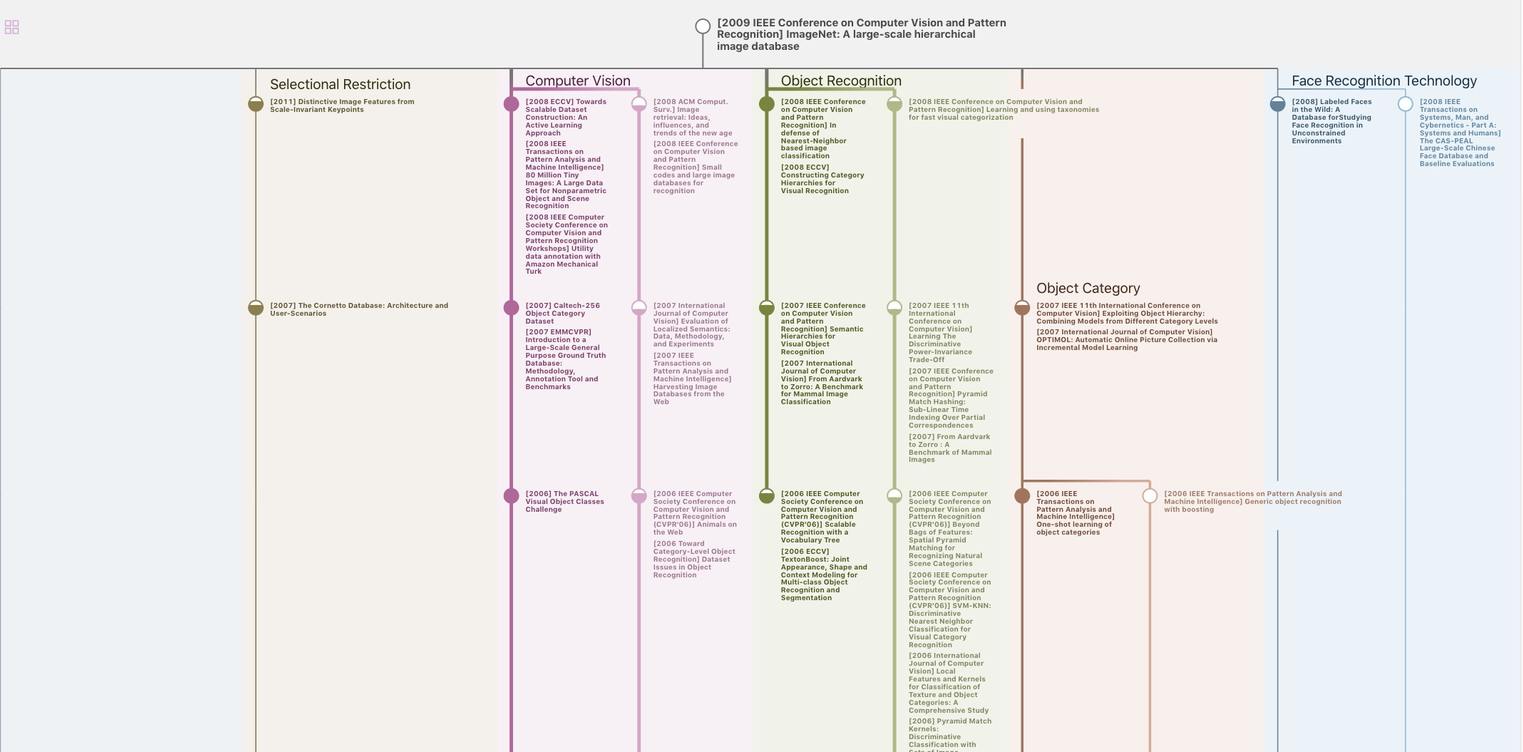
生成溯源树,研究论文发展脉络
Chat Paper
正在生成论文摘要