Reinforcement Learning-Based Motion Planning in Partially Observable Environments under Ethical Constraints
AI and Ethics(2024)
关键词
Ethical constraints,Partially observable,Complex task,Motion planning
AI 理解论文
溯源树
样例
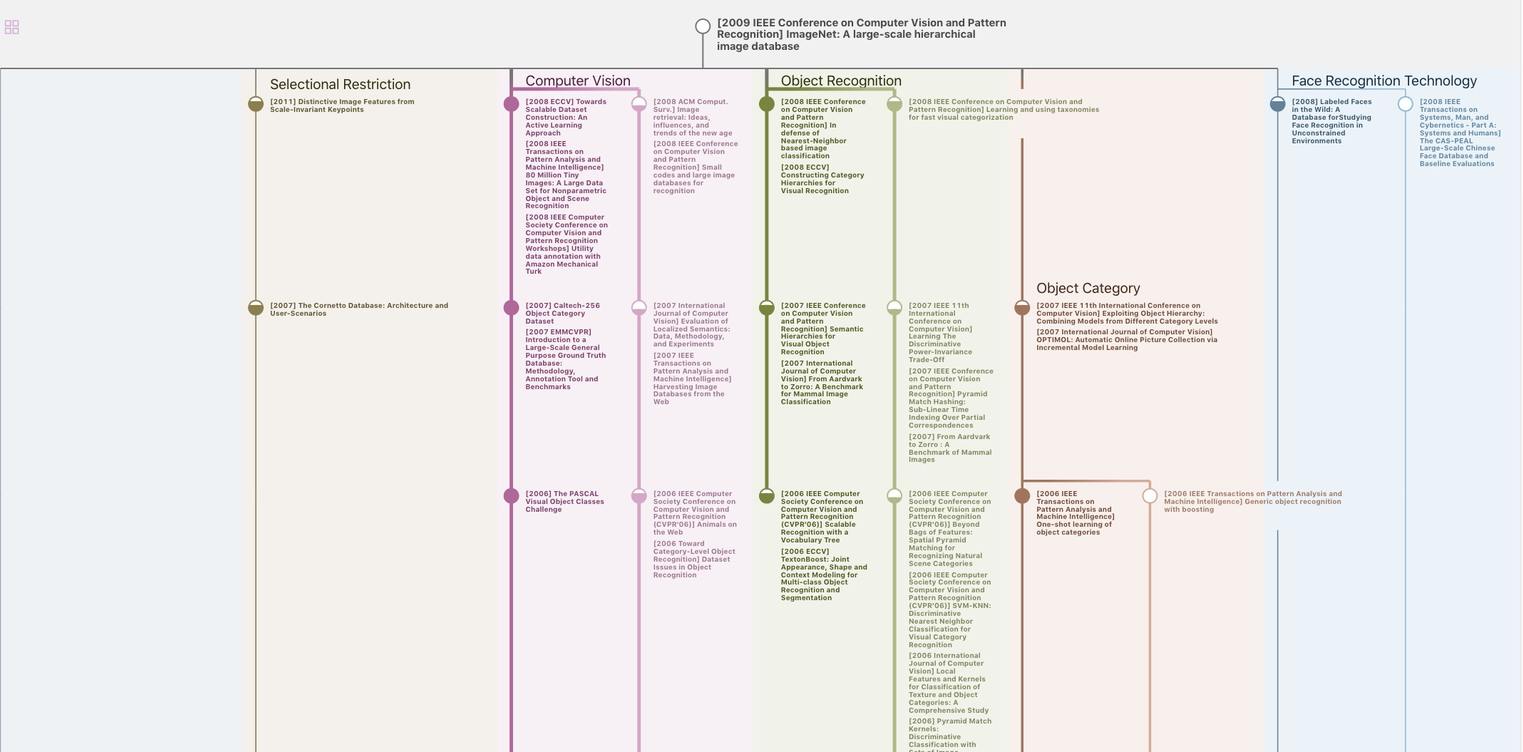
生成溯源树,研究论文发展脉络
Chat Paper
正在生成论文摘要