A Dual-mode Framework for Robust Long-term Tracking in Video SAR
IEEE Sensors Journal(2024)
摘要
Moving target shadows are being increasingly used in video synthetic aperture radar (video SAR) for moving target tracking. However, moving target shadows frequently disappear and appear caused by factors such as background occlusion and motion cessation. Most existing shadow-based tracking methods are inadequate in handling the aforementioned situation, particularly when confronted with the prolonged disappearance of moving target shadows, which leads to a significant deterioration in tracking performance. To solve the problem, we proposed a dual-mode framework for robust long-term tracking in video SAR. Specifically, the dual working mode, i.e., the local mode and the global mode, are constructed to deal with the appearance and the disappearance of the moving target shadows, respectively. In the local mode, we first design the modified discrimitant correlation filters network (m-DCFnet) by integrating the multilayer fusion strategy and the channel attention mechanism, as well as generate multiple candidate tracking results. After that, the Siamese-based network (SVnet) is proposed to screen out the optimal tracking result, which improves the tracking accuracy and the tracking precision. In the global mode, the window generation network (WGN) is first proposed to continually and rapidly search for the moving target shadows until they reappear. Then m-DCFnet and SVnet are successively utilized to re-track the reappearing target shadows. Experimental results on multiple real data demonstrate the effectiveness of the proposed tracking framework.
更多查看译文
关键词
Convolutional neural network (CNN),discriminant correlation filters (DCF),ground moving target (GMT),shadow tracking,video synthetic aperture radar (video SAR)
AI 理解论文
溯源树
样例
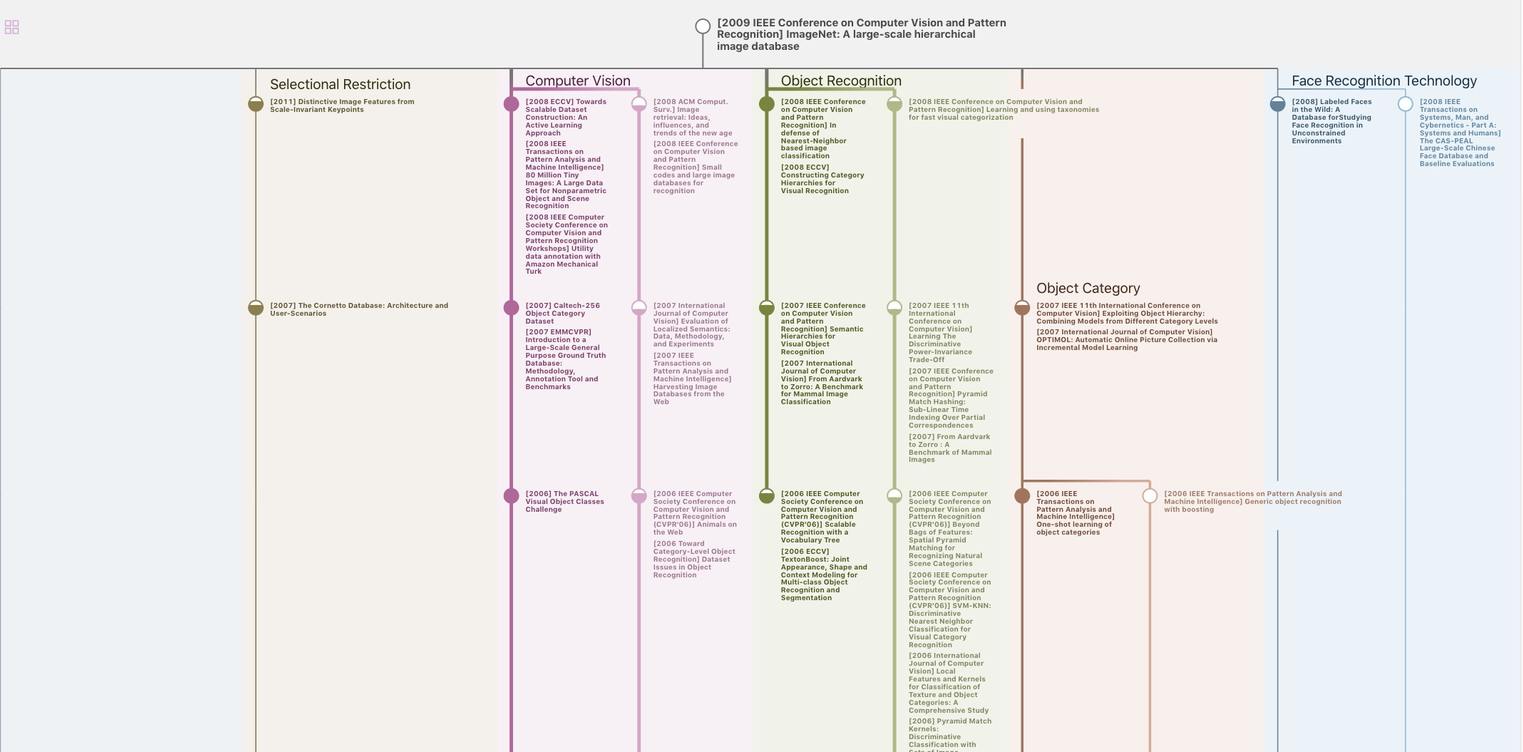
生成溯源树,研究论文发展脉络
Chat Paper
正在生成论文摘要