An Explainable Prediction for Dietary-Related Diseases via Language Models
NUTRIENTS(2024)
摘要
Our study harnesses the power of natural language processing (NLP) to explore the relationship between dietary patterns and metabolic health outcomes among Korean adults using data from the Seventh Korea National Health and Nutrition Examination Survey (KNHANES VII). Using Latent Dirichlet Allocation (LDA) analysis, we identified three distinct dietary patterns: "Traditional and Staple", "Communal and Festive", and "Westernized and Convenience-Oriented". These patterns reflect the diversity of dietary preferences in Korea and reveal the cultural and social dimensions influencing eating habits and their potential implications for public health, particularly concerning obesity and metabolic disorders. Integrating NLP-based indices, including sentiment scores and the identified dietary patterns, into our predictive models significantly enhanced the accuracy of obesity and dyslipidemia predictions. This improvement was consistent across various machine learning techniques-XGBoost, LightGBM, and CatBoost-demonstrating the efficacy of NLP methodologies in refining disease prediction models. Our findings underscore the critical role of dietary patterns as indicators of metabolic diseases. The successful application of NLP techniques offers a novel approach to public health and nutritional epidemiology, providing a deeper understanding of the diet-disease nexus. This study contributes to the evolving field of personalized nutrition and emphasizes the potential of leveraging advanced computational tools to inform targeted nutritional interventions and public health strategies aimed at mitigating the prevalence of metabolic disorders in the Korean population.
更多查看译文
关键词
dietary pattern,obesity,dyslipidemia,language model,natural language processing (NLP),explainable artificial intelligence (xAI)
AI 理解论文
溯源树
样例
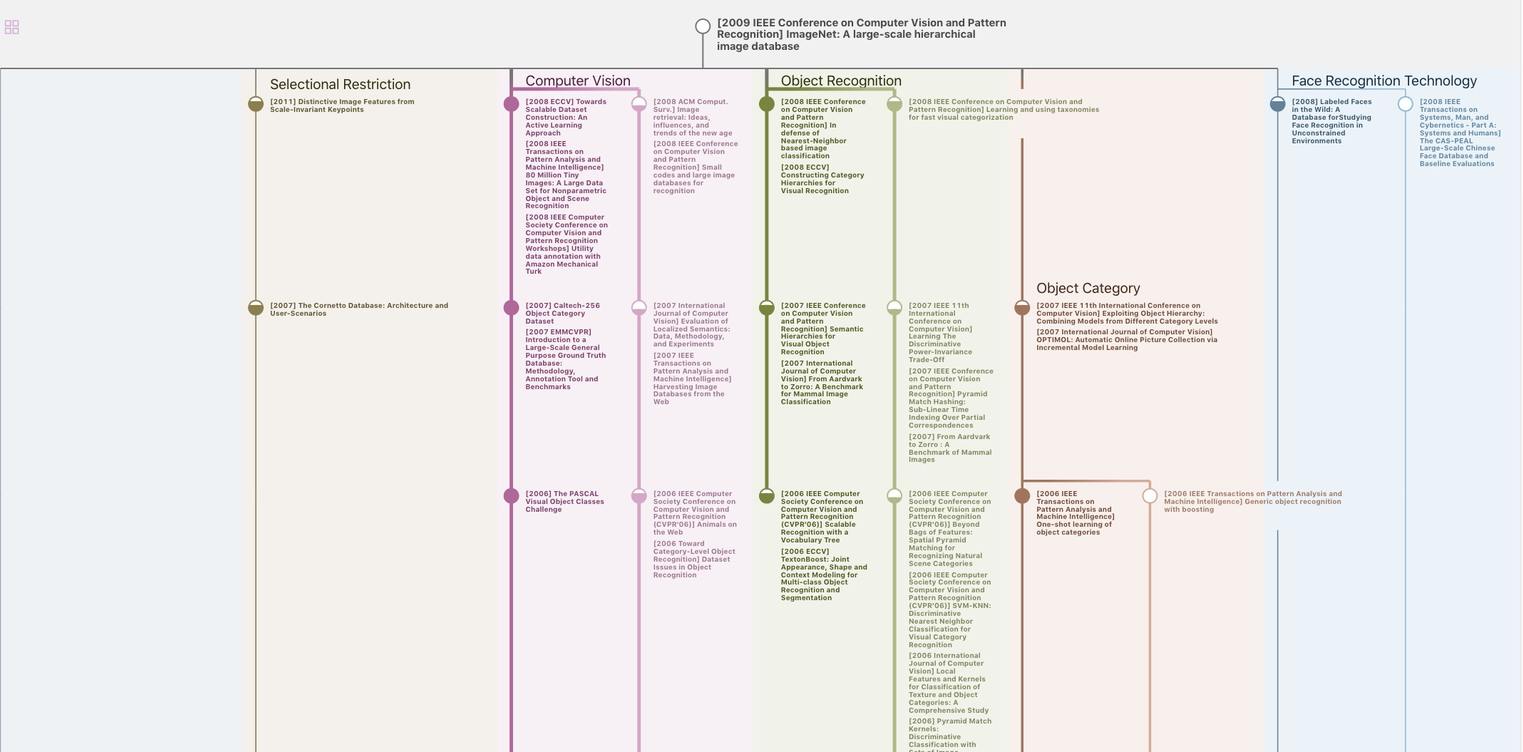
生成溯源树,研究论文发展脉络
Chat Paper
正在生成论文摘要