A Coarse-to-Fine Transformer-Based Network for 3D Reconstruction from Non-Overlapping Multi-View Images
REMOTE SENSING(2024)
摘要
Reconstructing 3D structures from non-overlapping multi-view images is a crucial task in the field of 3D computer vision, since it is difficult to establish feature correspondences and infer depth from overlapping parts of views. Previous methods, whether generating the surface mesh or volume of an object, face challenges in simultaneously ensuring the accuracy of detailed topology and the integrity of the overall structure. In this paper, we introduce a novel coarse-to-fine Transformer-based reconstruction network to generate precise point clouds from multiple input images at sparse and non-overlapping viewpoints. Specifically, we firstly employ a general point cloud generation architecture enhanced by the concept of adaptive centroid constraint for the coarse point cloud corresponding to the object. Subsequently, a Transformer-based refinement module applies deformation to each point. We design an attention-based encoder to encode both image projection features and point cloud geometric features, along with a decoder to calculate deformation residuals. Experiments on ShapeNet demonstrate that our proposed method outperforms other competing methods.
更多查看译文
关键词
point cloud reconstruction,Transformer,non-overlapping,multi-view
AI 理解论文
溯源树
样例
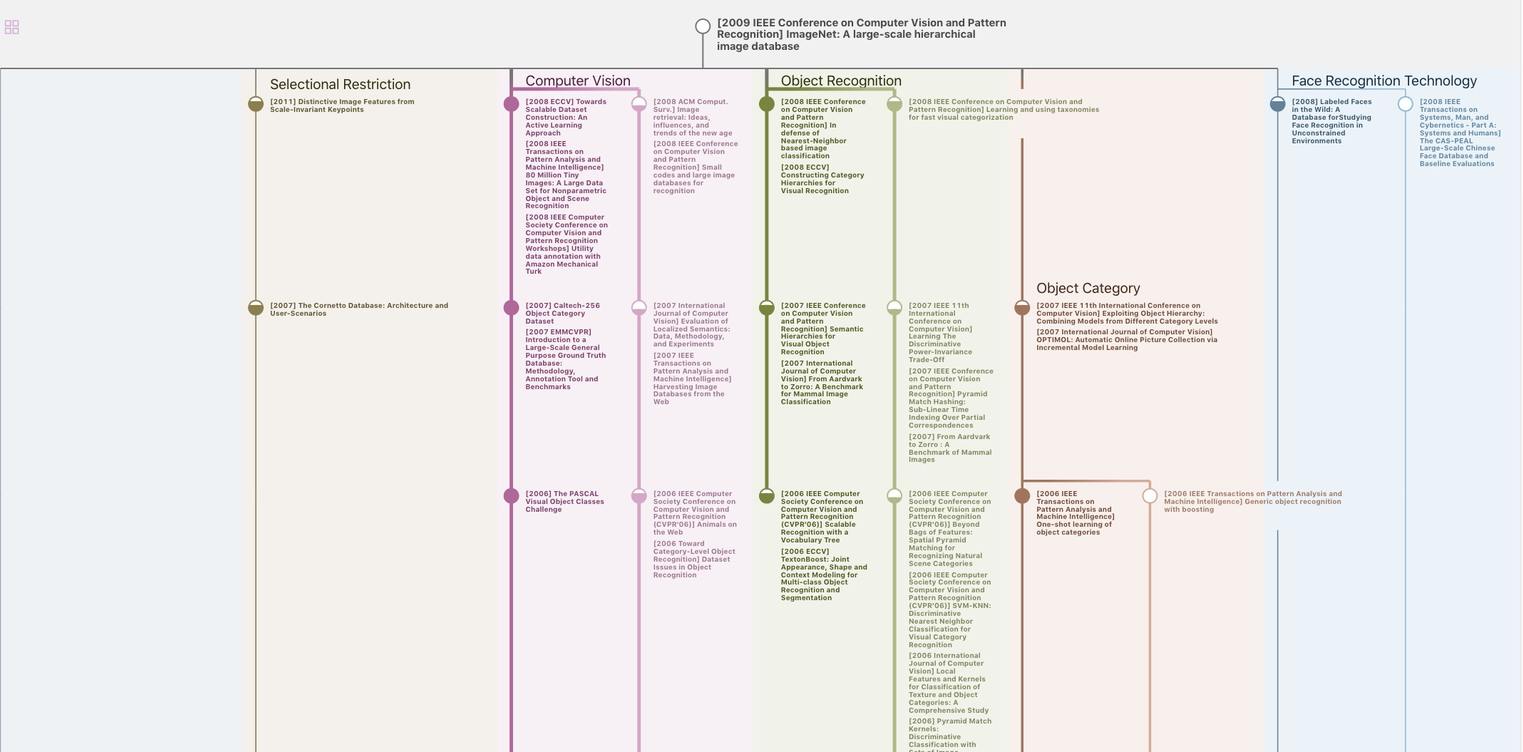
生成溯源树,研究论文发展脉络
Chat Paper
正在生成论文摘要