Two-Level Spatio-Temporal Feature Fused Two-Stream Network for Micro-Expression Recognition
SENSORS(2024)
摘要
Micro-expressions, which are spontaneous and difficult to suppress, reveal a person's true emotions. They are characterized by short duration and low intensity, making the task of micro-expression recognition challenging in the field of emotion computing. In recent years, deep learning-based feature extraction and fusion techniques have been widely used for micro-expression recognition, particularly methods based on Vision Transformer that have gained popularity. However, the Vision Transformer-based architecture used in micro-expression recognition involves a significant amount of invalid computation. Additionally, in the traditional two-stream architecture, although separate streams are combined through late fusion, only the output features from the deepest level of the network are utilized for classification, thus limiting the network's ability to capture subtle details due to the lack of fine-grained information. To address these issues, we propose a new two-level spatio-temporal feature fused with a two-stream architecture. This architecture includes a spatial encoder (modified ResNet) for learning texture features of the face, a temporal encoder (Swin Transformer) for learning facial muscle motor features, a feature fusion algorithm for integrating multi-level spatio-temporal features, a classification head, and a weighted average operator for temporal aggregation. The two-stream architecture has the advantage of extracting richer features compared to the single-stream architecture, leading to improved performance. The shifted window scheme of Swin Transformer restricts self-attention computation to non-overlapping local windows and allows cross-window connections, significantly improving the performance and reducing the computation compared to Vision Transformer. Moreover, the modified ResNet is computationally less intensive. Our proposed feature fusion algorithm leverages the similarity in output feature shapes at each stage of the two streams, enabling the effective fusion of multi-level spatio-temporal features. This algorithm results in an improvement of approximately 4% in both the F1 score and the UAR. Comprehensive evaluations conducted on three widely used spontaneous micro-expression datasets (SMIC-HS, CASME II, and SAMM) consistently demonstrate the superiority of our approach over comparative methods. Notably, our approach achieves a UAR exceeding 0.905 on CASME II, making it one of the few frameworks in the published micro-expression recognition literature to achieve such high performance.
更多查看译文
关键词
micro-expression recognition,two-stream architecture,feature fusion algorithm,shifted window-based self-attention
AI 理解论文
溯源树
样例
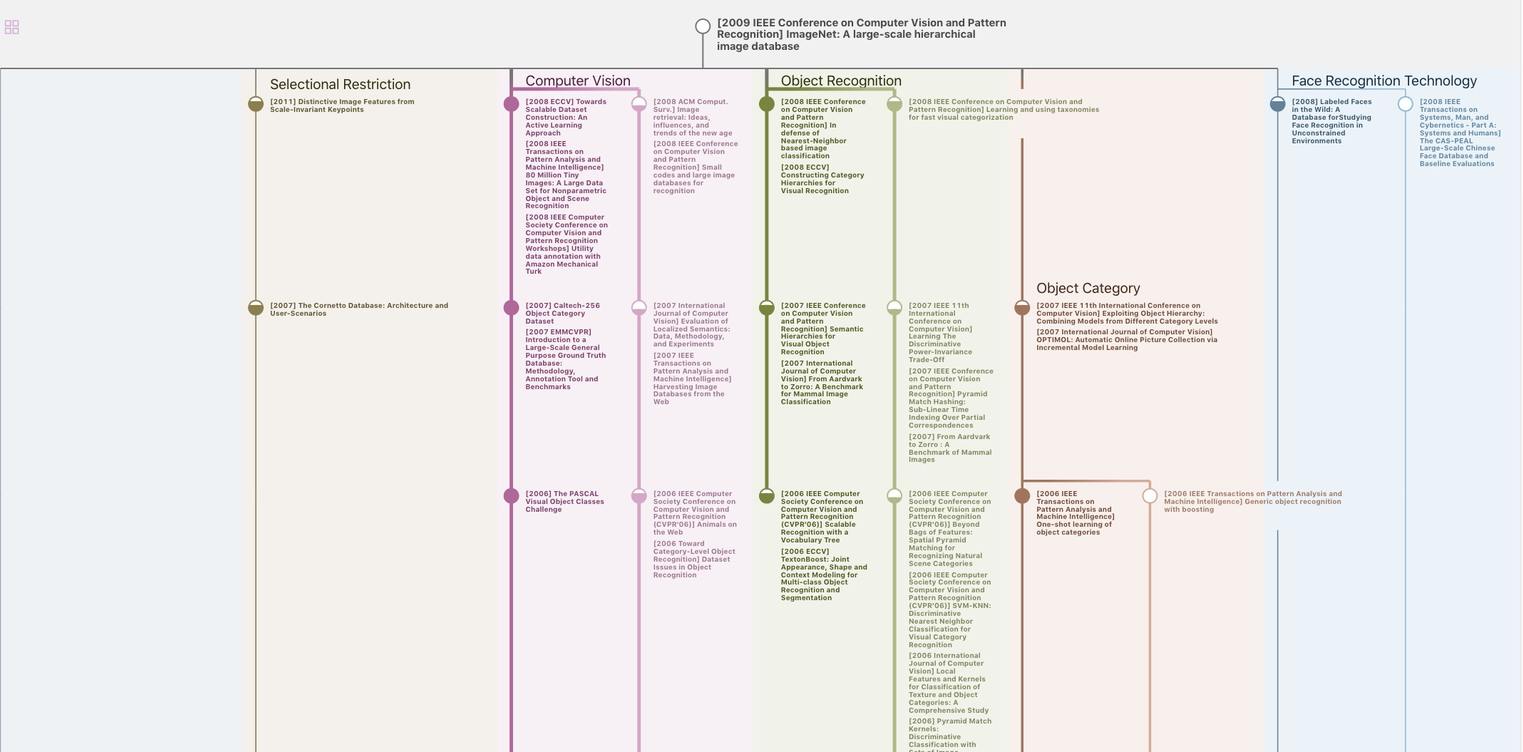
生成溯源树,研究论文发展脉络
Chat Paper
正在生成论文摘要