Gaussian Mixture Estimation from Lower-Dimensional Data with Application to PET Imaging
MATHEMATICS(2024)
摘要
In positron emission tomography (PET), the original points of emission are unknown, and the scanners record pairs of photons emitting from those origins and creating lines of response (LORs) in random directions. This presents a latent variable problem, since at least one dimension of relevant information is lost. This can be solved by a statistical approach to image reconstruction-modeling the image as a Gaussian mixture model (GMM). This allows us to obtain a high-quality continuous model that is not computationally demanding and does not require postprocessing. In this paper, we propose a novel method of GMM estimation in the PET setting, directly from lines of response. This approach utilizes some well-known and convenient properties of the Gaussian distribution and the fact that the random slopes of the lines are independent from the points of origin. The expectation-maximization (EM) algorithm that is most commonly used to estimate GMMs in the traditional setting here is adapted to lower-dimensional data. The proposed estimation method is unbiased, and simulations and experiments show that accurate reconstruction on synthetic data is possible from relatively small samples.
更多查看译文
关键词
Gaussian mixture models,EM algorithm,positron emission tomography,missing data
AI 理解论文
溯源树
样例
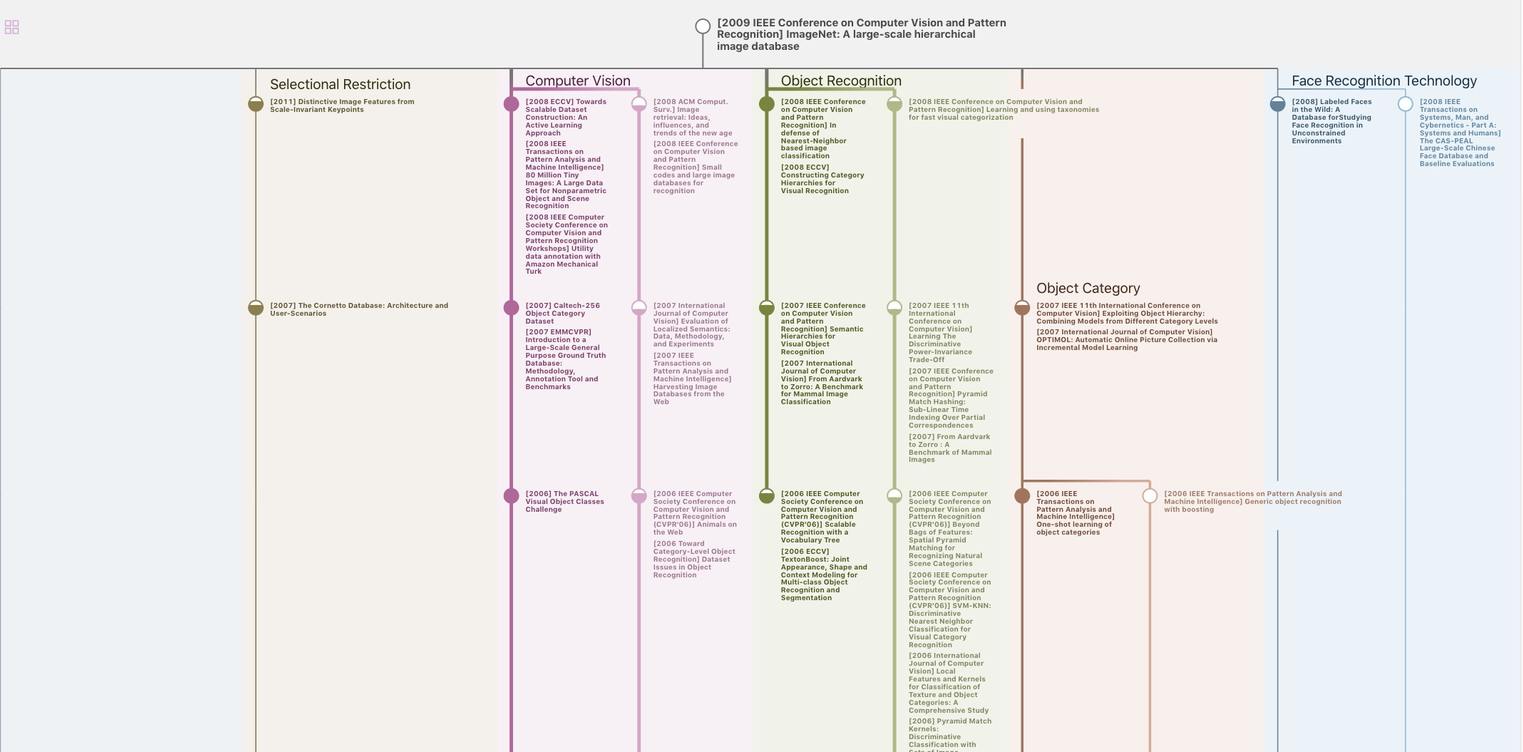
生成溯源树,研究论文发展脉络
Chat Paper
正在生成论文摘要