Urban Traffic Flow Prediction Based on Bayesian Deep Learning Considering Optimal Aggregation Time Interval
SUSTAINABILITY(2024)
摘要
Predicting short-term urban traffic flow is a fundamental and cost-effective strategy in traffic signal control systems. However, due to the interrupted, periodic, and stochastic characteristics of urban traffic flow influenced by signal control, there are still unresolved issues related to the selection of the optimal aggregation time interval and the quantifiable uncertainties in prediction. To tackle these challenges, this research introduces a method for predicting urban interrupted traffic flow, which is based on Bayesian deep learning and considers the optimal aggregation time interval. Specifically, this method utilizes the cross-validation mean square error (CVMSE) method to obtain the optimal aggregation time interval and to establish the relationship between the optimal aggregation time interval and the signal cycle. A Bayesian LSTM-CNN prediction model, which extends the LSTM-CNN model under the Bayesian framework to a probabilistic model to better capture the stochasticity and variation in the data, is proposed. Experimental results derived from real-world data demonstrate gathering traffic flow data based on the optimal aggregation time interval significantly enhances the prediction accuracy of the urban interrupted traffic flow model. The optimal aggregation time interval for urban interrupted traffic flow data corresponds to a multiple of the traffic signal control cycle. Comparative experiments indicate that the Bayesian LSTM-CNN prediction model outperforms the state-of-the-art prediction models.
更多查看译文
关键词
urban interrupted flow,optimal aggregation time interval,signal control cycle,short term traffic flow prediction,Bayesian deep learning
AI 理解论文
溯源树
样例
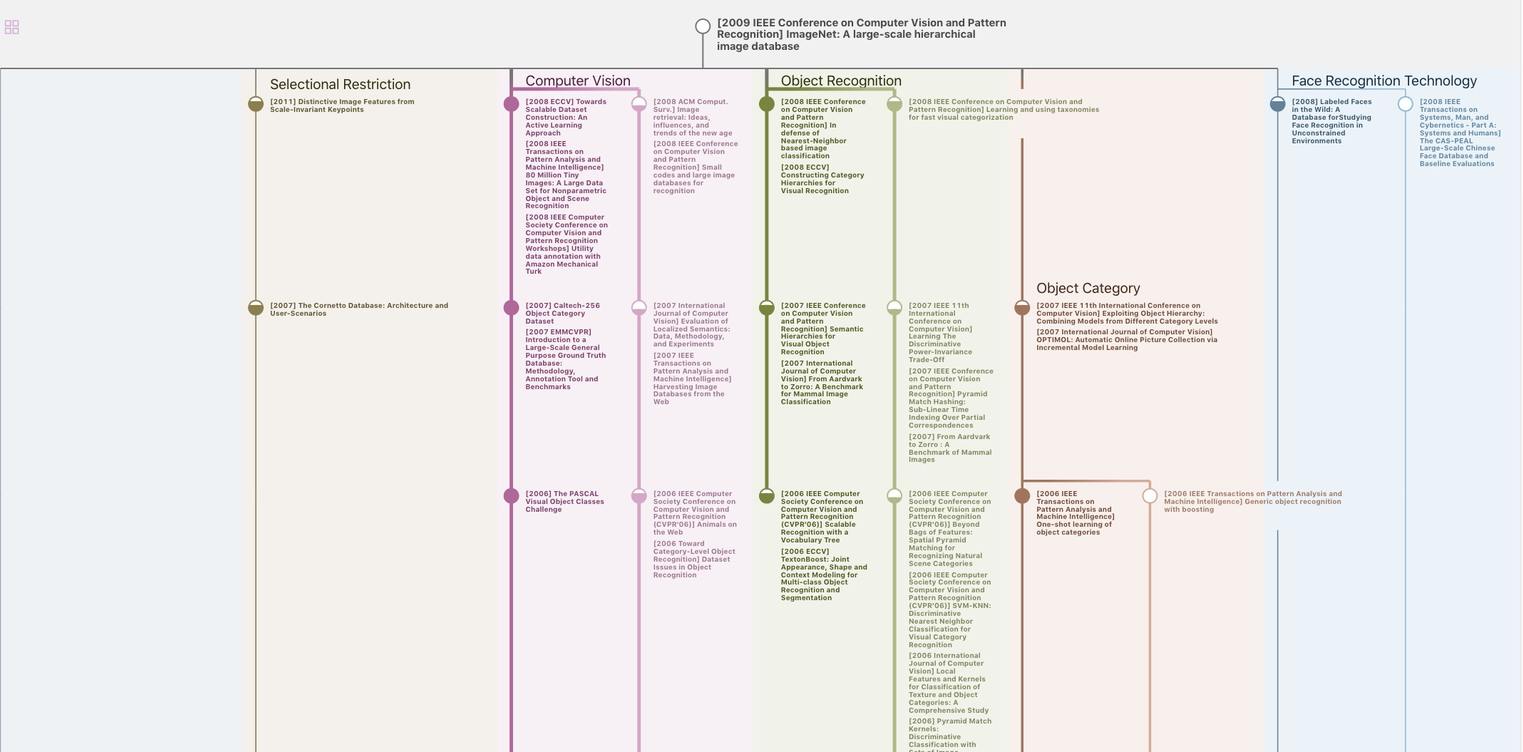
生成溯源树,研究论文发展脉络
Chat Paper
正在生成论文摘要