CSI-GLSTN: A Location-Independent CSI Human Activity Recognition Method Based on Spatio-Temporal and Channel Feature Fusion
IEEE Transactions on Instrumentation and Measurement(2024)
摘要
In the field of Human Activity Recognition (HAR) based on Wi-Fi signals, channel characteristics are susceptible to environmental influences, leading to significant feature variations among samples from different locations. Consequently, when existing model-based HAR systems attempt to recognize activities across different locations, the accuracy of pre-trained models often deteriorates. To address this challenge, a location-independent HAR system based Channel State Information(CSI) named Graph structure Learning based graph Saptio-temporal and Transformer Network(CSI-GLSTN) is proposed, which treats the sub-channels of Wi-Fi signals as nodes in a graph and constructs an initial graph structure through a graph structure learning layer. It employs a graph spatio-temporal network to fuse temporal and channel features of activity samples and map the fused features back to the initial graph structure. Through optimization and updates with activity samples from different locations, the final graph structure integrates both spatio-temporal and channel features of activities, characterizing different activity types. This achieves the decoupling of activity features from their respective locations, ultimately serving the goal of location-independent HAR. After training on samples from ten different locations, the system achieves an average recognition accuracy of 95.2% for six different types of human activities. Experimental results validate the system’s generalization performance in HAR across different locations.
更多查看译文
AI 理解论文
溯源树
样例
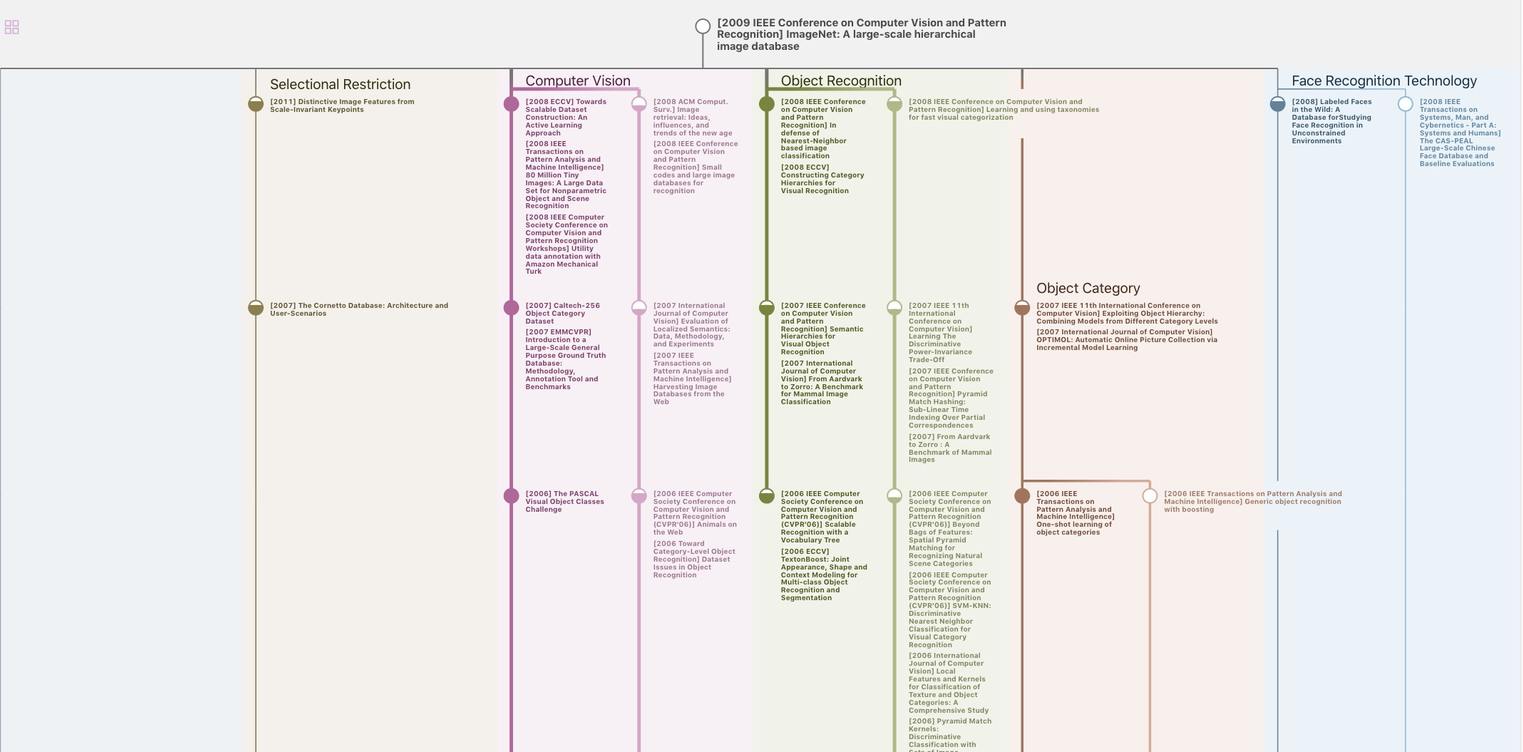
生成溯源树,研究论文发展脉络
Chat Paper
正在生成论文摘要