Model-Based Off-Policy Deep Reinforcement Learning With Model-Embedding
IEEE TRANSACTIONS ON EMERGING TOPICS IN COMPUTATIONAL INTELLIGENCE(2024)
摘要
Model-based reinforcement learning (MBRL) has shown its advantages in sample efficiency over model-free reinforcement learning (MFRL) by leveraging control-based domain knowledge. Despite the impressive results it achieves, MBRL is still outperformed by MFRL due to the lack of unlimited interactions with the environment. While imaginary data can be generated by imagining the trajectories of future states, a trade-off between the usage of data generation and the influence of model bias remains to be resolved. In this paper, we propose a simple and elegant off-policy model-based deep reinforcement learning algorithm with a model embedded in the framework of probabilistic reinforcement learning, called MEMB. To balance the sample-efficiency and model bias, we exploit both real and imaginary data in training. In particular, we embed the model in the policy update and learn value functions from the real data set. We also provide a theoretical analysis of MEMB with the Lipschitz continuity assumption on the model and policy, proving the reliability of the short-term imaginary rollout. Finally, we evaluate MEMB on several benchmarks and demonstrate that our algorithm can achieve state-of-the-art performance.
更多查看译文
关键词
Model-based,reinforcement learning,deep reinforcement learning,machine learning
AI 理解论文
溯源树
样例
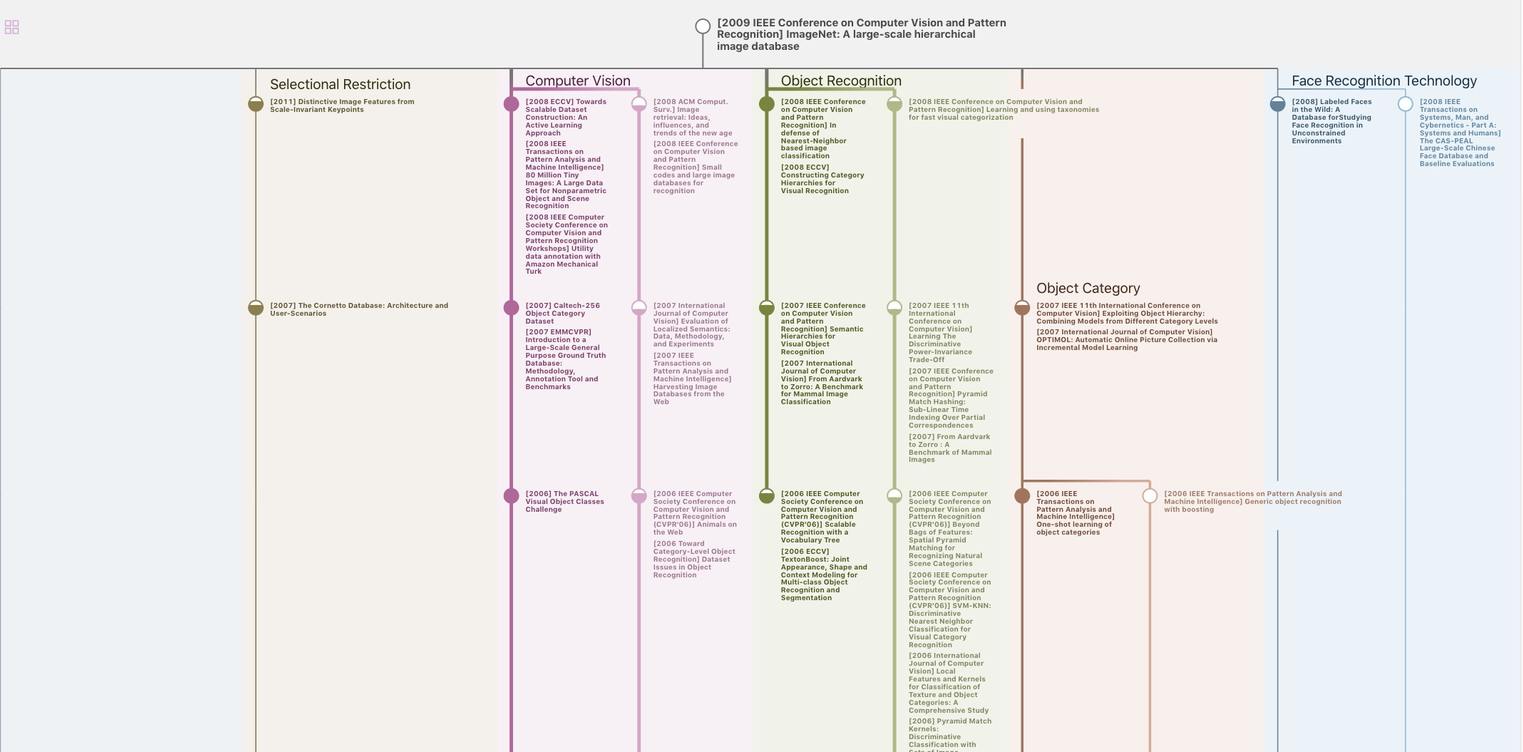
生成溯源树,研究论文发展脉络
Chat Paper
正在生成论文摘要