Towards a Dynamic Future with Adaptable Computing and Network Convergence (ACNC)
arxiv(2024)
摘要
In the context of advancing 6G, a substantial paradigm shift is anticipated,
highlighting comprehensive everything-to-everything interactions characterized
by numerous connections and stringent adherence to Quality of
Service/Experience (QoS/E) prerequisites. The imminent challenge stems from
resource scarcity, prompting a deliberate transition to Computing-Network
Convergence (CNC) as an auspicious approach for joint resource orchestration.
While CNC-based mechanisms have garnered attention, their effectiveness in
realizing future services, particularly in use cases like the Metaverse, may
encounter limitations due to the continually changing nature of users,
services, and resources. Hence, this paper presents the concept of Adaptable
CNC (ACNC) as an autonomous Machine Learning (ML)-aided mechanism crafted for
the joint orchestration of computing and network resources, catering to dynamic
and voluminous user requests with stringent requirements. ACNC encompasses two
primary functionalities: state recognition and context detection. Given the
intricate nature of the user-service-computing-network space, the paper employs
dimension reduction to generate live, holistic, abstract system states in a
hierarchical structure. To address the challenges posed by dynamic changes,
Continual Learning (CL) is employed, classifying the system state into contexts
controlled by dedicated ML agents, enabling them to operate efficiently. These
two functionalities are intricately linked within a closed loop overseen by the
End-to-End (E2E) orchestrator to allocate resources. The paper introduces the
components of ACNC, proposes a Metaverse scenario to exemplify ACNC's role in
resource provisioning with Segment Routing v6 (SRv6), outlines ACNC's workflow,
details a numerical analysis for efficiency assessment, and concludes with
discussions on relevant challenges and potential avenues for future research.
更多查看译文
AI 理解论文
溯源树
样例
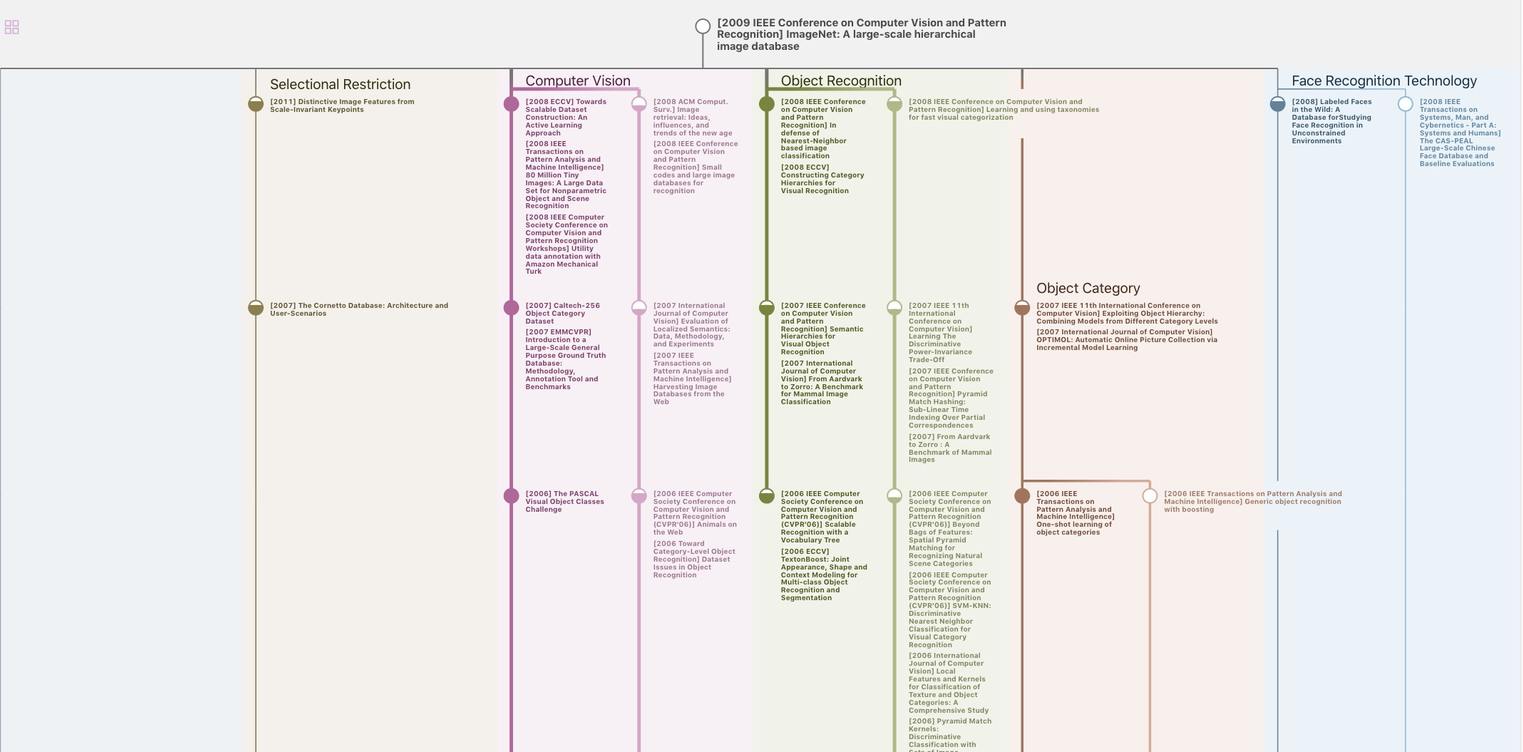
生成溯源树,研究论文发展脉络
Chat Paper
正在生成论文摘要