Synth^2: Boosting Visual-Language Models with Synthetic Captions and Image Embeddings
arxiv(2024)
摘要
The creation of high-quality human-labeled image-caption datasets presents a
significant bottleneck in the development of Visual-Language Models (VLMs). We
propose a novel approach that leverages the strengths of Large Language Models
(LLMs) and image generation models to create synthetic image-text pairs for
efficient and effective VLM training. Our method employs pretraining a
text-to-image model to synthesize image embeddings starting from captions
generated by an LLM. These synthetic pairs are then used to train a VLM.
Extensive experiments demonstrate that the VLM trained with synthetic data
exhibits comparable performance on image captioning, while requiring a fraction
of the data used by models trained solely on human-annotated data. In
particular, we outperform the baseline by 17
synthetic dataset. Furthermore, we show that synthesizing in the image
embedding space is 25
a promising technique for generating large-scale, customizable image datasets,
leading to enhanced VLM performance and wider applicability across various
domains, all with improved data efficiency and resource utilization.
更多查看译文
AI 理解论文
溯源树
样例
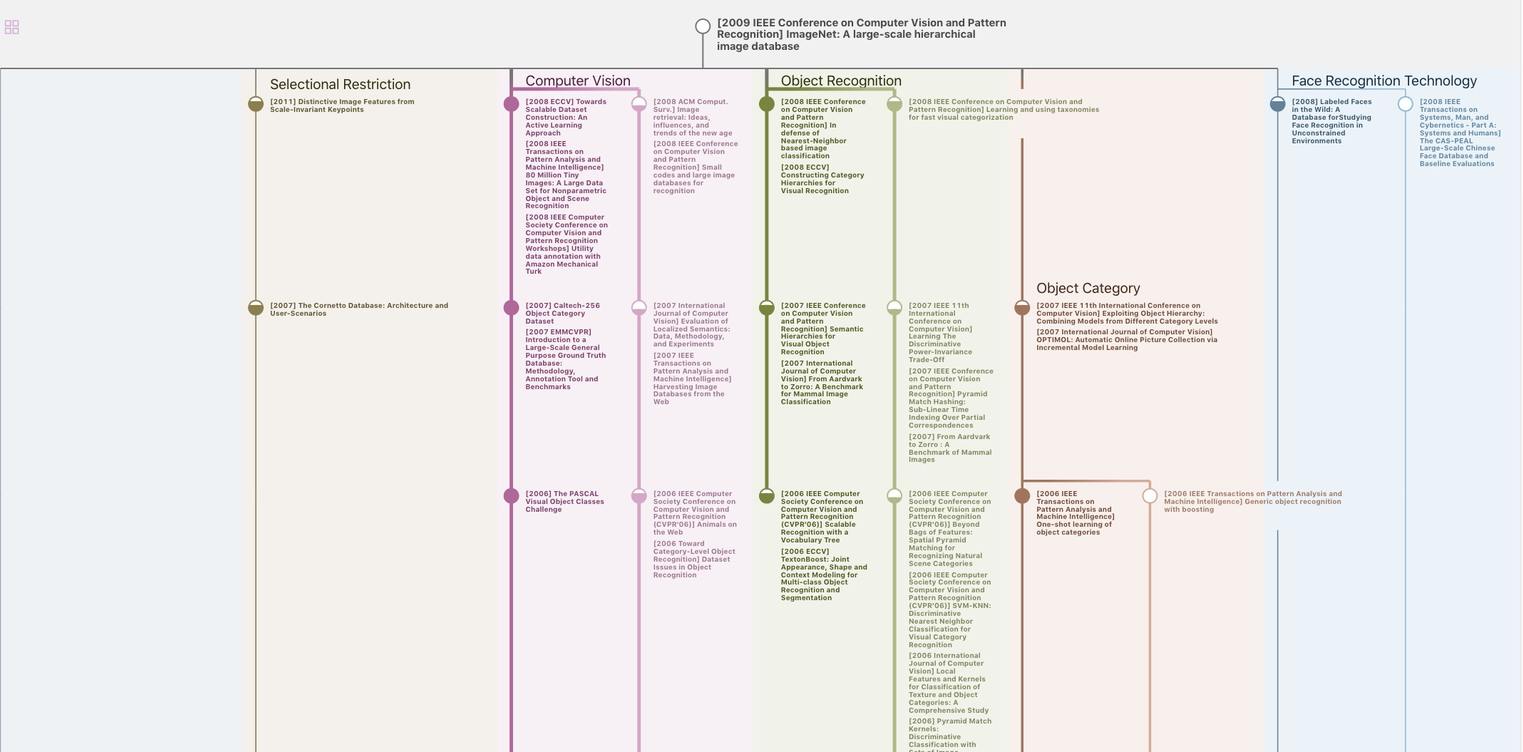
生成溯源树,研究论文发展脉络
Chat Paper
正在生成论文摘要