BASS: A Blockchain-Based Asynchronous SignSGD Architecture for Efficient and Secure Federated Learning
IEEE Transactions on Dependable and Secure Computing(2024)
摘要
Federated learning (FL) is a distributed framework for machine learning that enables collaborative training of a shared model across data silos while preserving data privacy. However, the FL aggregation server faces a challenge in waiting for a large volume of model parameters from selected nodes before generating a global model, which leads to inefficient communication and aggregation. Although transmitting only the signs of stochastic gradient descent (SignSGD) reduces the transmission load, it decreases model accuracy, and the time waiting for local model collection remains substantial. Moreover, the security of FL is severely compromised by prevalent poisoning, backdoor, and DDoS attacks, causing ineffective and inaccurate model training. To overcome these challenges, this paper proposes a
B
lockchain-based
A
synchronous
S
ign
S
GD (BASS) architecture for efficient and secure federated learning. By integrating a blockchain-based semi-asynchronous aggregation scheme with sign-based gradient compression, BASS considerably improves communication and aggregation efficiency, while providing resistance against attacks. Besides, a novel node-summarized sign aggregation algorithm is developed for the blockchain leaders to ensure the convergence and accuracy of the global model. An open-source prototype is developed, on top of which extensive experiments are conducted. The results validate the superiority of BASS in terms of efficiency, model accuracy, and security.
更多查看译文
关键词
Federated Learning,Blockchain,SignSGD,Efficiency,Security
AI 理解论文
溯源树
样例
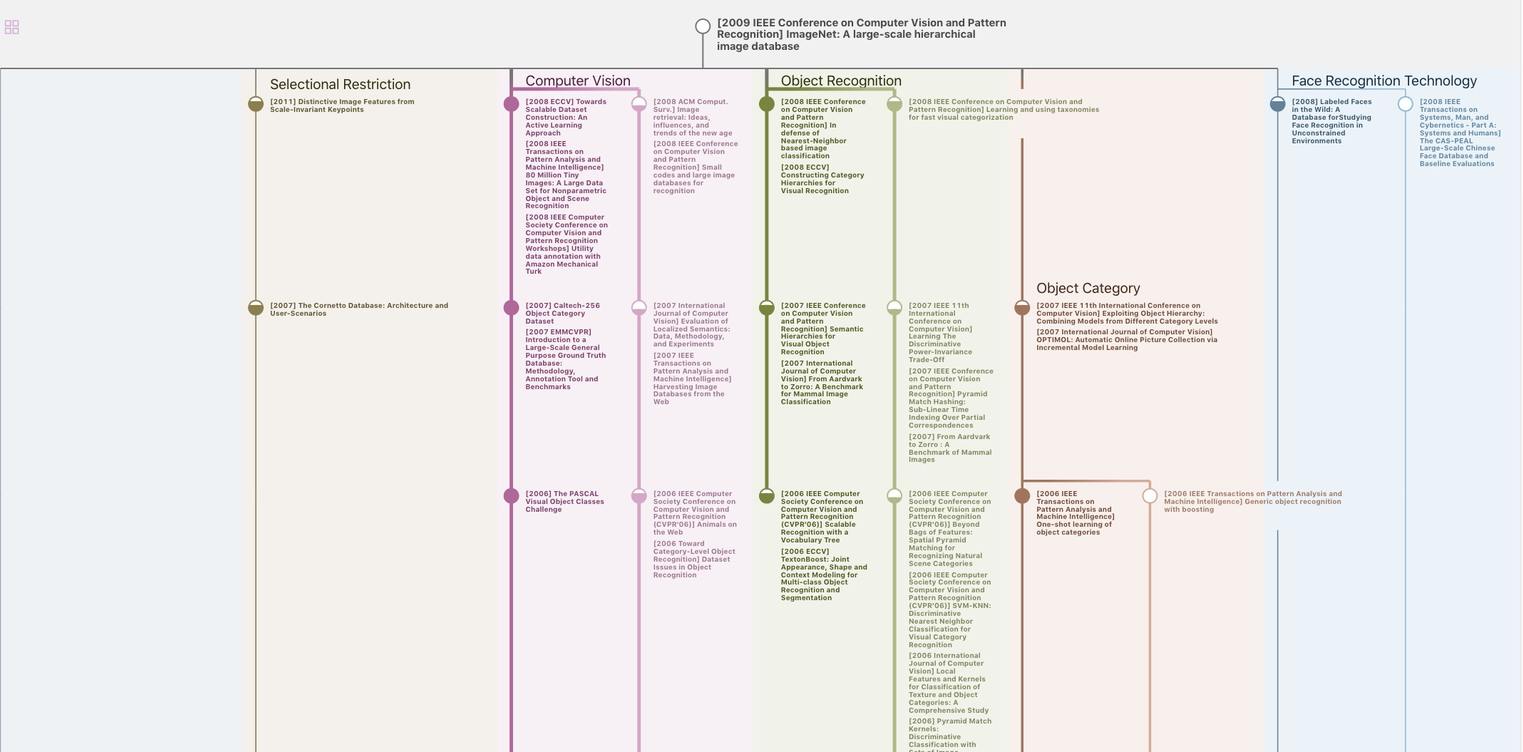
生成溯源树,研究论文发展脉络
Chat Paper
正在生成论文摘要