Deep Learning-based Bearing Fault Diagnosis Using a Trusted Multi-scale Quadratic Attention-embedded Convolutional Neural Network
IEEE Transactions on Instrumentation and Measurement(2024)
摘要
Bearing fault diagnosis is essential for ensuring the safety and reliability of industrial systems. Recently, deep learning approaches, especially the convolutional neural network, have demonstrated exceptional performance in bearing fault diagnosis. However, the limited availability of training samples has been a persistent issue, leading to a significant reduction in diagnostic accuracy. Additionally, noise interference or load variation during bearing operation pose significant challenges for fault diagnosis. To tackle the above issues, this paper explores the application of quadratic neuron with attention-embedded for fault diagnosis networks and introduces a trusted multi-scale learning strategy that fully considers the characteristics of bearing vibration signals. Building upon these concepts, a trusted multi-scale quadratic attention-embedded convolutional neural network is proposed for bearing faults diagnosis. Experimental results indicate that the proposed network outperforms six stateof-the-art networks under noise interference or load variation superimposed on small samples.
更多查看译文
关键词
Bearing fault diagnosis,Trusted multi-scale learning,Quadratic attention-embedded convolution neural network
AI 理解论文
溯源树
样例
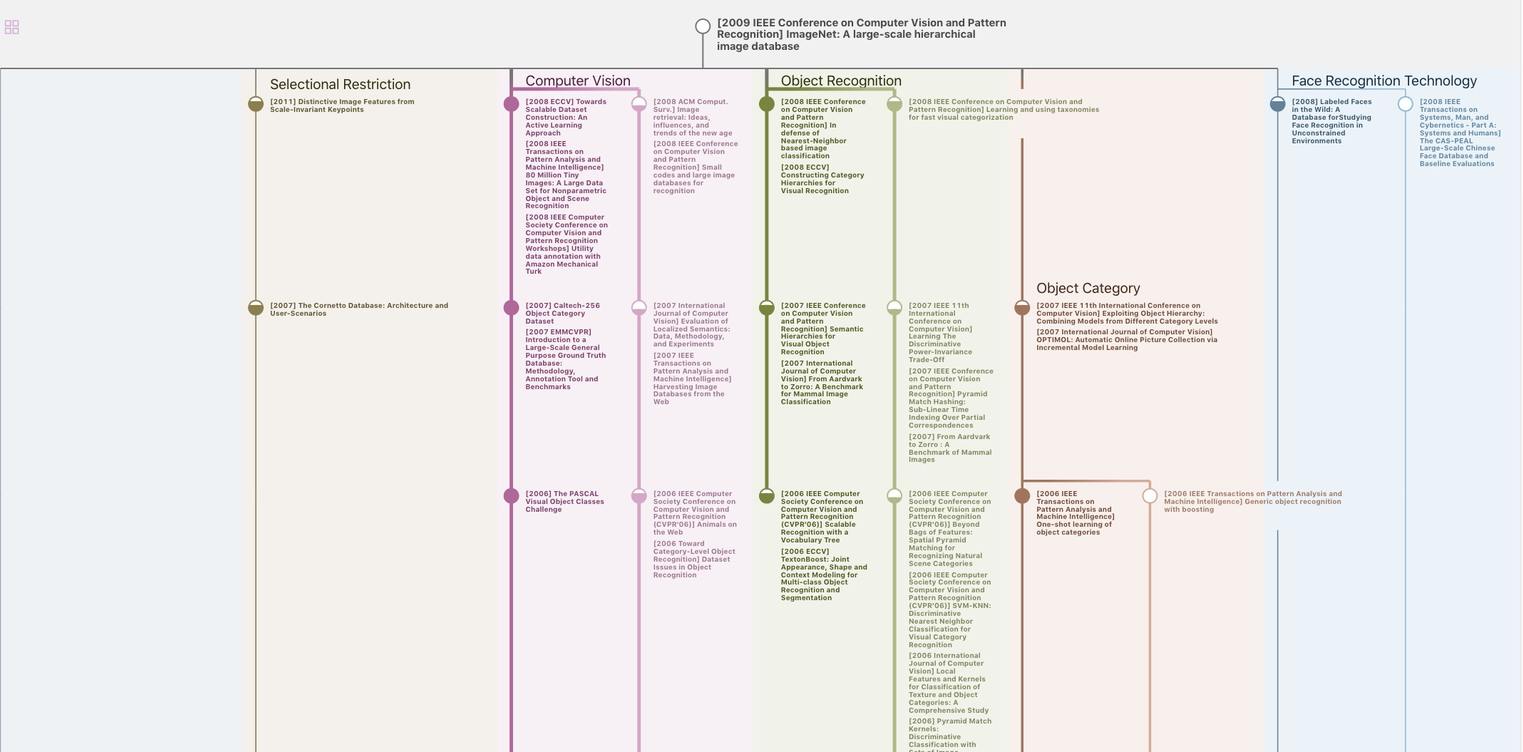
生成溯源树,研究论文发展脉络
Chat Paper
正在生成论文摘要