Multi Self-Supervised Pre-Finetuned Transformer Fusion for Better Vehicle Detection
IEEE transactions on automation science and engineering(2024)
摘要
Vehicle detection is an important task in intelligent transportation, which can provides services for road condition monitoring and highway toll collection systems. However existing detection methods are limited by two aspects. First, there is a difference between the model knowledge pre-trained on large-scale datasets and the knowledge required for target task. Second, most detection models follow the pattern of single-source learning, which limits the learning ability. To address these problems, we propose a Multi Self-supervised Pre-finetuned Transformer Fusion (MSPTF) network, consisting of two steps: self-supervised pre-finetuning domain knowledge learning and multi-model fusion target task learning. In the first step, we introduced self-supervised learning methods into transformer pre-finetuning to reduce data costs and alleviate knowledge gap. In the second step, we take feature information differences between different model architectures and pre-finetuning tasks into account and propose Multi-model Semantic Consistency Cross-attention Fusion (MSCCF) network to combine different transformer features by considering channel semantic consistency and feature vector semantic consistency, which obtain more complete and proper fusion features for detection task. We experimented the proposed method on two vehicle detection datasets and achieved 1.1%, 5.5% improvement compared with baseline and 0.7%, 1.8% compared with sota, which proved the effectiveness of our method. Note to Practitioners-Vehicle detection can provide basic services for many intelligent transportation scenarios, but the results of training and adjusting existing models in vehicle detection scenarios with limited data are often not ideal. This article proposes MSPTF to improve model performance from two aspects. First, we randomly collect additional vehicle pictures, and train the model without the need for annotation through annotation-free methods such as image feature extraction and reconstruction, so that the model can be trained on more vehicle-related pictures with low data cost. In addition, we trained multiple models in the above way and proposed a fusion method to combine the knowledge of multiple models for vehicle detection. The results show that our Our model is able to learn from additional unlabeled vehicle images as well as multiple trained models information to achieve better vehicle detection.
更多查看译文
关键词
Pre-finetuning,broad learning,multi-model fusion,intelligent transportation system,object detection
AI 理解论文
溯源树
样例
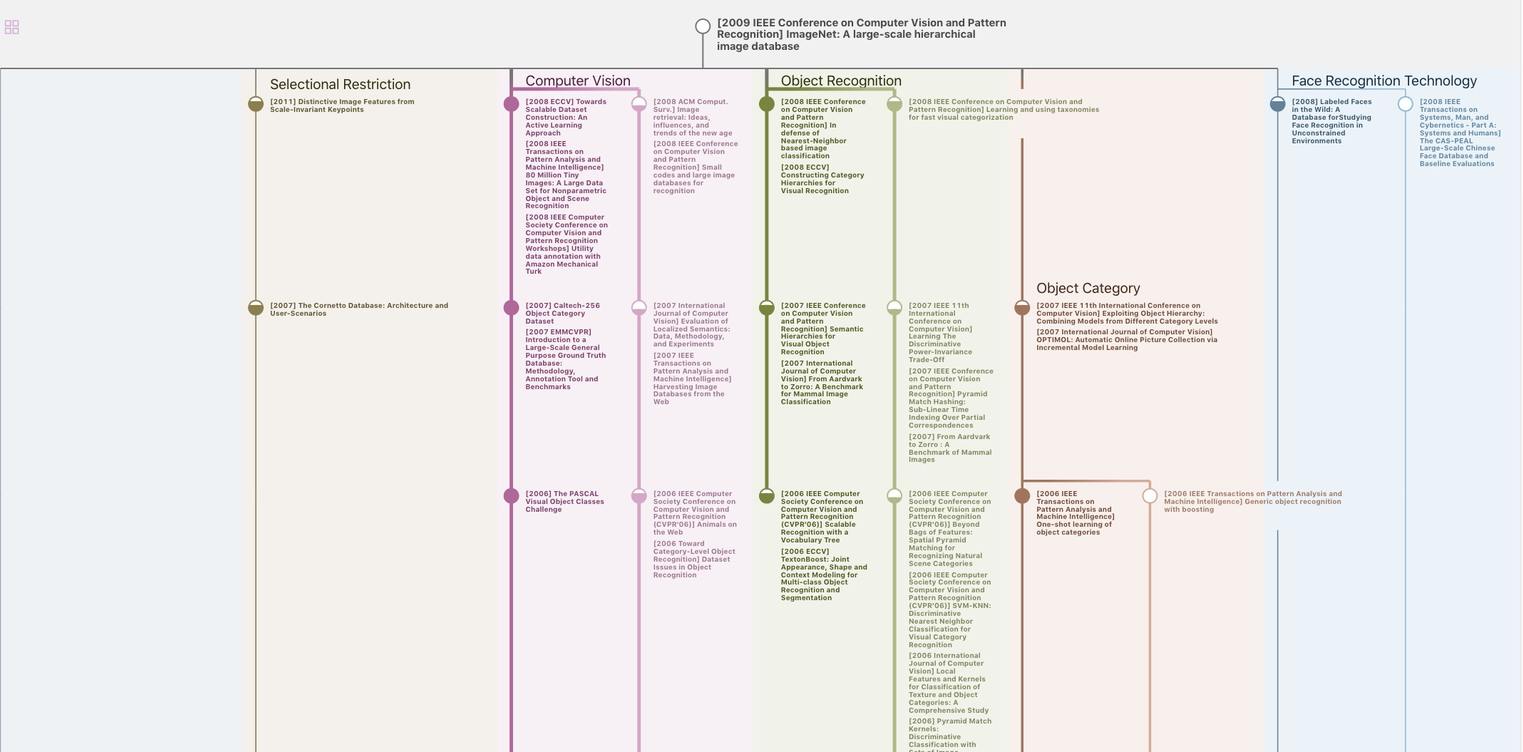
生成溯源树,研究论文发展脉络
Chat Paper
正在生成论文摘要