LapEfficientDepth: lightweight model for monocular depth estimation based on small samples
crossref(2024)
摘要
Abstract This study proposes an improved LapDepth model, named LapEfficientDepth, for the monocular depth estimation field. The objective is to reduce the error in model-predicted relative depths and enhance depth estimation accuracy, addressing the issues of substantial resource consumption and large parameter count inherent in the original model. By incorporating lightweight modules, the LapEfficientDepth model significantly reduces model the complexity and resource requirements of the model while maintaining high accuracy estimation. Specifically, the parameter count of the LapEfficientDepth model has been reduced to 6M, constituting only 8.2% of the parameter volume found in the original LapDepth model, while achieving an approximate 1% improvement in accuracy compared to the Lite-Mono-8M model, which has a similar number of parameters. In addition, the LapEfficientDepth model exhibits exceptional transfer learning capabilities. After pre-training on the KITTI dataset and further training on the ETH3D-S dataset, the model achieved a1, a2, and a3 metrics of 0.706, 0.997, and 0.999, respectively, proving its rapid adaptability and learning ability on small sample datasets. This offers an effective solution for high-performance, lightweight monocular depth estimation network models.
更多查看译文
AI 理解论文
溯源树
样例
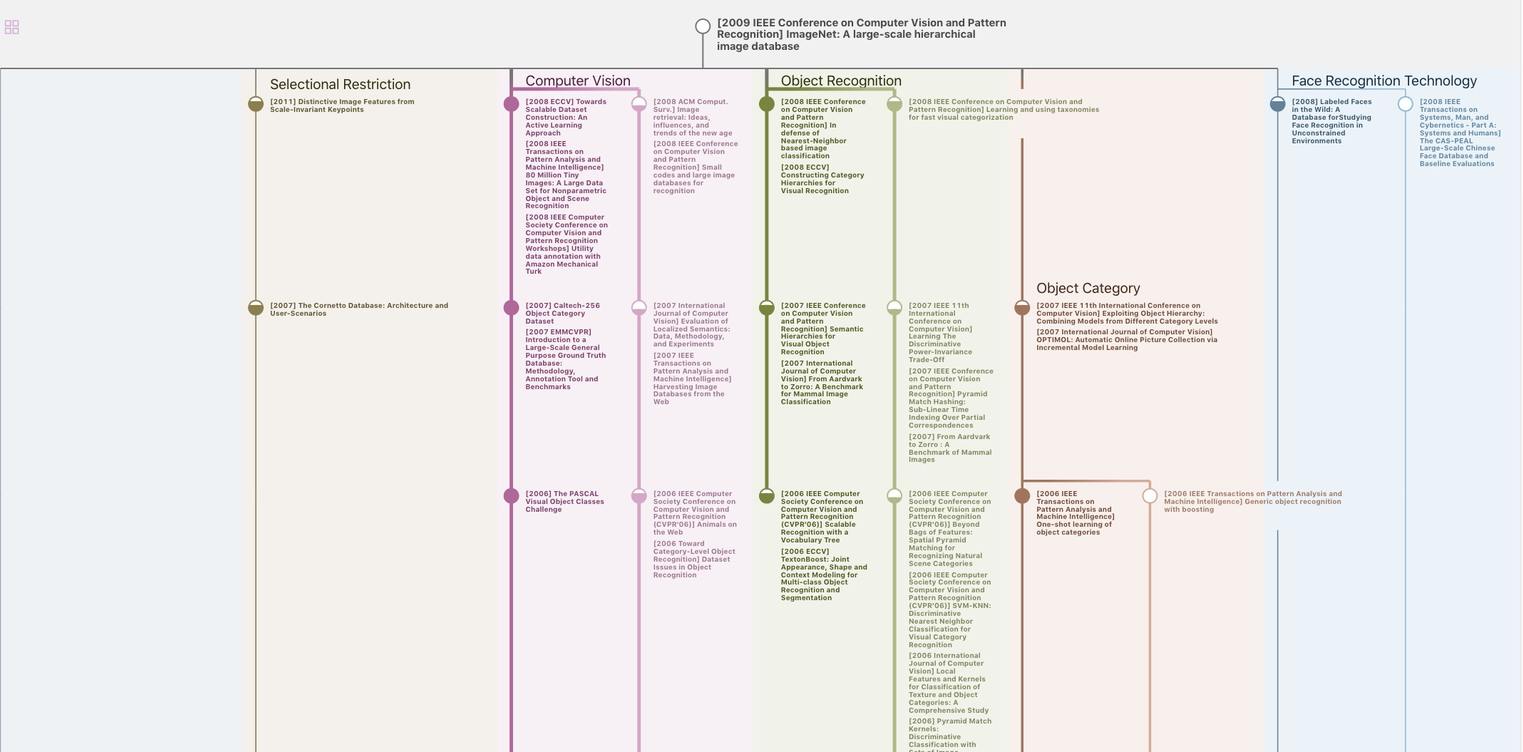
生成溯源树,研究论文发展脉络
Chat Paper
正在生成论文摘要