Predicting RUNX1::RUNX1T1 genetic abnormalities in acute myeloid leukemia from bone marrow smears: Can artificial intelligence do better?
crossref(2024)
摘要
Abstract Background: The presence of the RUNX1::RUNX1T1 fusion gene in patients diagnosed with acute myeloid leukemia (AML) subtype is often indicated by distinctive morphological features in myeloblasts from bone marrow (BM) smears. This study aims to evaluate the capacity of artificial intelligence (AI) to identify specific genetic abnormalities based solely on morphological characteristics. The intent is to investigate a non-invasive, cost-effective, and efficient preliminary screening method prior to the application of molecular biological assays. Methods: This multicenter trial included 205 patients diagnosed with AML, of which 75 were AML with RUNX1::RUNX1T1 fusion. A dataset of 65,039 myeloblasts images collected from the BM smears of these patients was compiled for model training, testing, and validation. The study also undertook a comparative analysis of the discrepancies between manual microscopy and AI-based identification. Results: The model demonstrated proficiency in adapting to varied clinical scenarios by applying two different threshold values. Under the threshold of 0.59, the testing and validation cohorts showed sensitivities of 92.86% and 95.65%, with corresponding accuracies of 87.04% and 71.88%. Conversely, by setting the threshold at 0.88, specificities of 92.31% and 92.68% were achieved, along with accuracies of 88.89% and 90.63%. Regardless of the threshold, the AI model consistently outperformed manual microscopy (average accuracy: 50.00%). Conclusion: The model demonstrates a significant capability to discern underlying RUNX1::RUNX1T1 genetic alterations from the morphological attributes of BM nucleated cells with a precision surpassing human observation. This providing a valuable tool highlights its potential for enhancing diagnostic efficiency in clinical practice.
更多查看译文
AI 理解论文
溯源树
样例
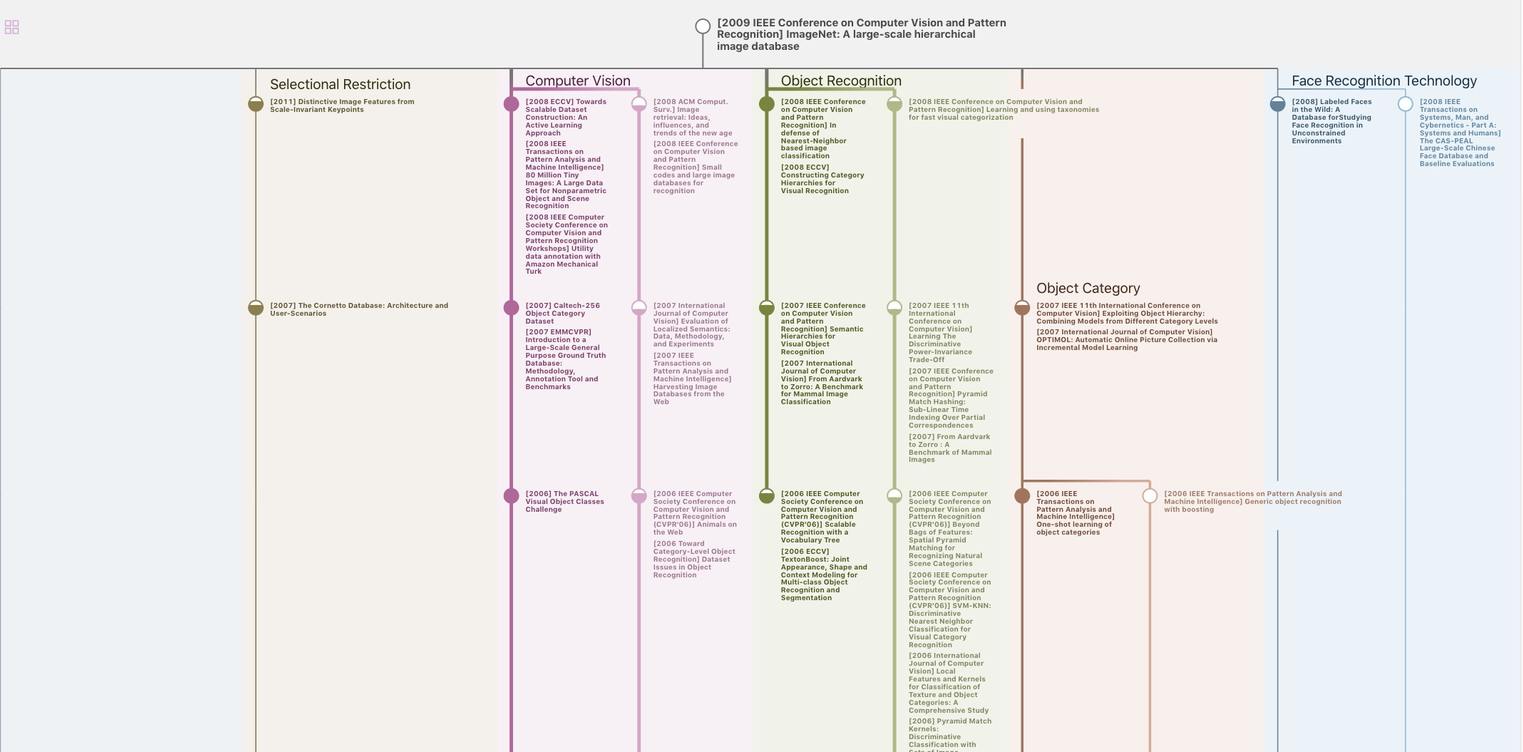
生成溯源树,研究论文发展脉络
Chat Paper
正在生成论文摘要