Comparison of Drone-Assisted Maize Seedling Detection Models Based on Deep Learning
crossref(2024)
摘要
Effective agricultural management in maize production operations starts with the early quantification of seedlings. Accurately determining plant presence allows growers to optimize planting density, allocate resources, and detect potential growth issues early on. This study presents an in-depth analysis of multiple object detection models used by drones to count maize seedlings, while concurrently investigating the influence of planting density, flight altitude, and plant growth stage. The findings of this study demonstrate that one-stage models are able to more accurately detect maize seedlings than two-stage models. In particular, YOLOv8n exhibits outstanding performance, achieving an F1-score of over 0.92 under various density conditions and maintaining a stable performance, especially under densities below 105,000 plants/ha. Additionally, planting density and growth stage were observed to significantly affect detection accuracy, with performance declining as density and growth stage increased. Image resolution and detection were impacted by flight altitude, with lower flights producing higher-quality results. Ultimately, YOLOv8n displays optimal performance under various conditions, providing crucial data to support intelligent decision-making. This research offers valuable insights into the application of object detection technology and provides a basis for the future development of precision agriculture.
更多查看译文
AI 理解论文
溯源树
样例
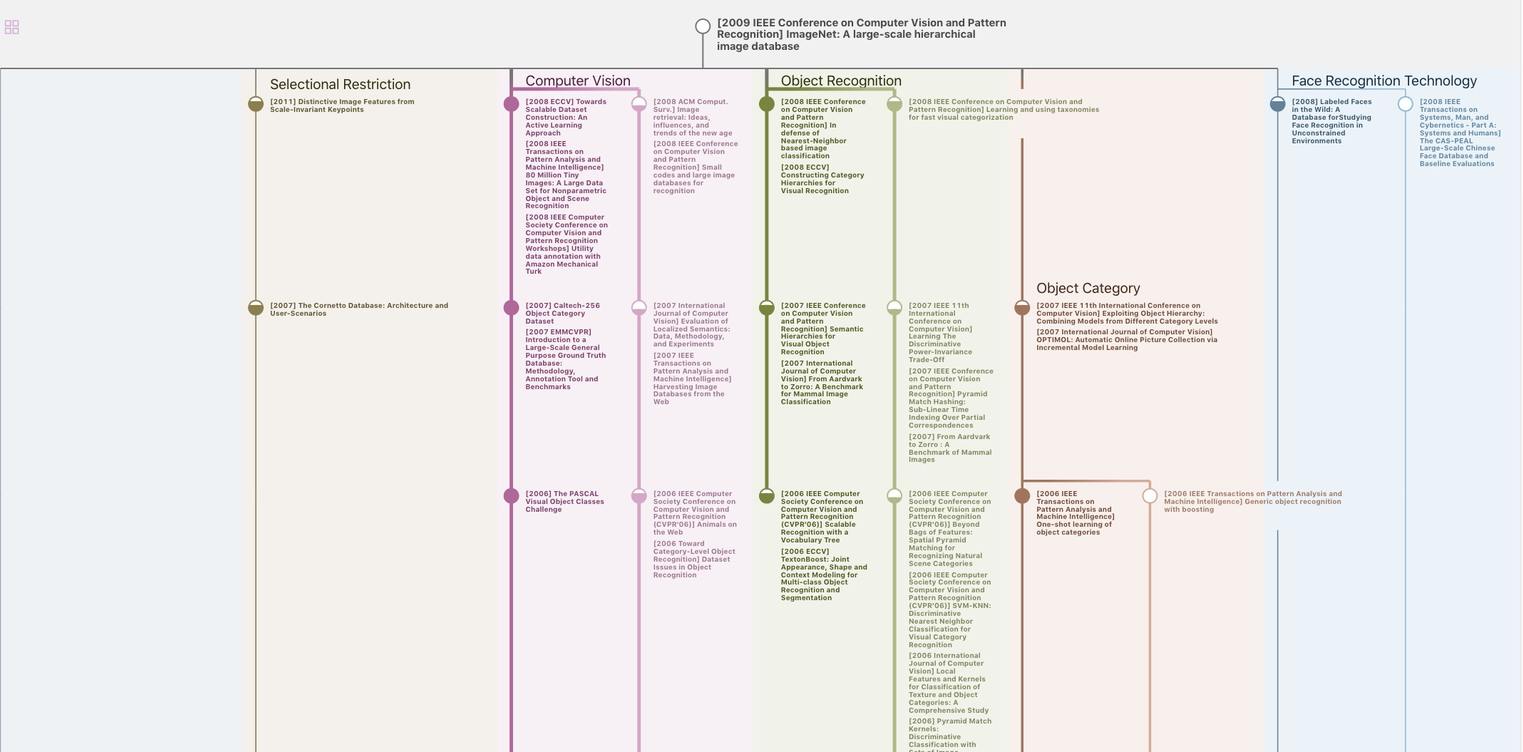
生成溯源树,研究论文发展脉络
Chat Paper
正在生成论文摘要