End-to-End High-Throughput Approach for Data-Driven Internal Donor Development in Heterogeneous Ziegler-Natta Propylene Polymerization
ACS catalysis(2024)
摘要
Internal donors (IDs) play a decisive role in shaping the structure and performance of Ziegler-Natta catalyst formulations for isotactic polypropylene production. Unfortunately, their diverse and intricate functions remain elusive, and rational ID discovery, therefore, is still problematic. Exploitation of artificial intelligence methods such as machine learning, in turn, has been hindered by the lack of training data sets with adequate quality and size. This study proposes an integrated high-throughput workflow encompassing catalyst synthesis, propylene polymerization, and polypropylene characterization. Its application to an ID library of 35 molecules generated a robust and consistent data set, which highlighted important and intriguing quantitative structure-property relations (QSPRs). Furthermore, by fingerprinting ID molecular structure in combination with feature selection, a black box QSPR model correlating ID molecular structure and catalytic performance was successfully implemented. This study demonstrates that the combination of high-throughput experimentation and machine learning is a promising asset for accelerating the research and development of Ziegler-Natta catalysts.
更多查看译文
关键词
heterogeneous Ziegler-Natta catalyst,propylenepolymerization,internal donor,high-throughputexperimentation,machine learning
AI 理解论文
溯源树
样例
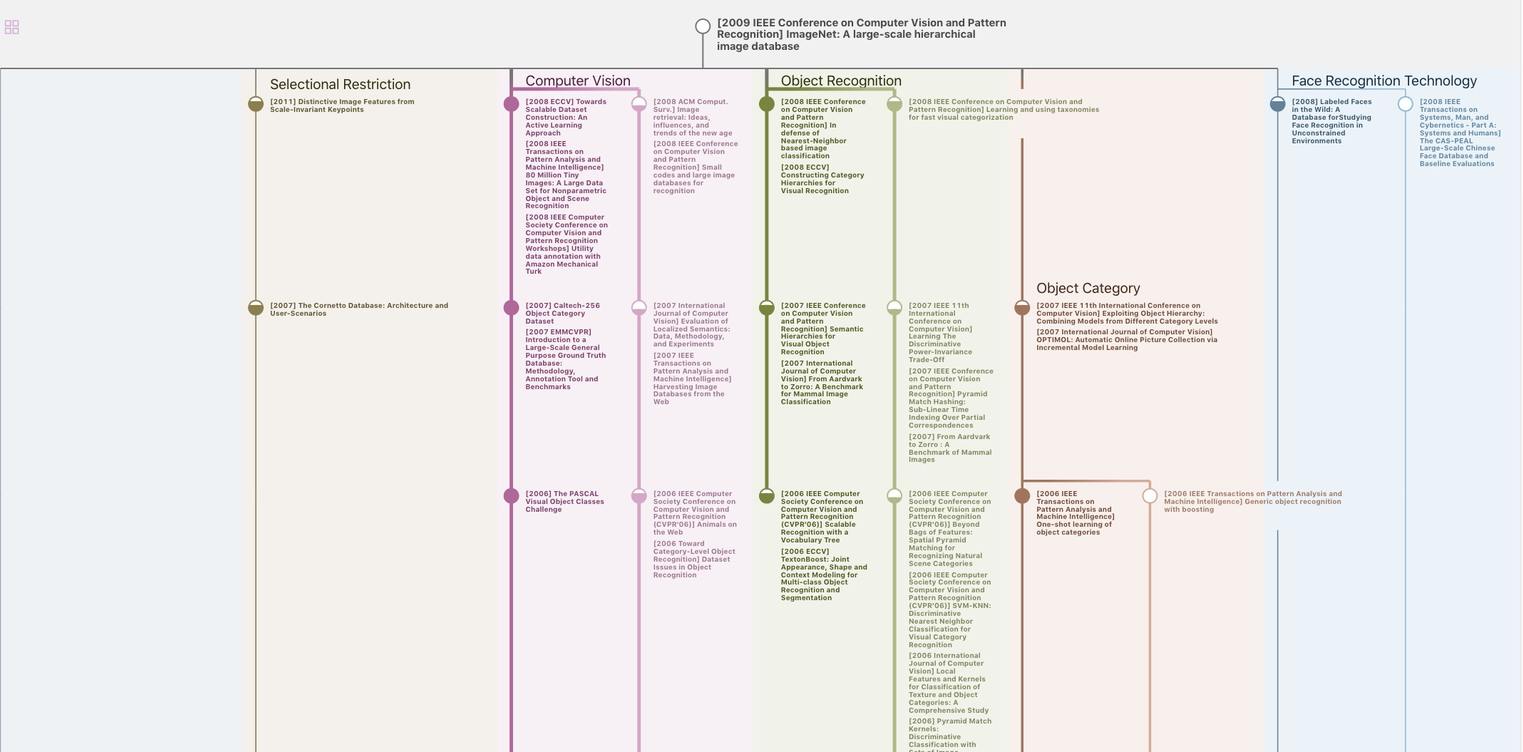
生成溯源树,研究论文发展脉络
Chat Paper
正在生成论文摘要