A Physics-Based Learning Approach for ROADM-Induced Anomaly Localization and Estimation
Journal of Lightwave Technology(2024)
摘要
Reconfigurable optical add-drop multiplexers (ROADMs) based on wavelength selective switches (WSSs) are crucial devices in optical networks, facilitating reconfigurable optical routing. In practical systems, soft failures in WSSs may occur, leading to connection disruptions if not addressed promptly. For rapid network diagnosis and maintenance, it is essential to quickly localize the failed WSS and estimate the failure magnitude. However, current approaches rely on scarce real-system failure data or require the installation of additional monitors. To address these limitations, in this paper, we propose a physics-based learning approach (PBLA) to localize the failed WSS and estimate the failure magnitude with two types of failure: filter shift (FS) and filter tightening (FT), without using historical failure data and additional monitors at the repeater nodes. The method leverages the power spectrum (PS) extracted from the chromatic dispersion compensation (CDC) module in receiver digital signal processing (DSP) as input. It formulates an optimization problem to minimize the discrepancy between the extracted PS and the PS calculated through a theoretical model. By solving the optimization problem, the method can effectively localize the failed WSS and estimate the failure magnitude in WSSs. The method is validated through extensive simulations, exhibiting a high localization accuracy and a small estimation error. Furthermore, we thoroughly explore the influence of various factors, including the measurement of PS, failure location, magnitude, the increasing number of WSSs, different symbol rates, and model inaccuracy, on the performance of our method, demonstrating its adaptability across diverse scenarios. Finally, an experimental demonstration further substantiates our method's performance in localizing and estimating WSS-related anomalies in optical networks.
更多查看译文
关键词
Physics-based learning,soft failure localization,soft failure estimation,optical communication
AI 理解论文
溯源树
样例
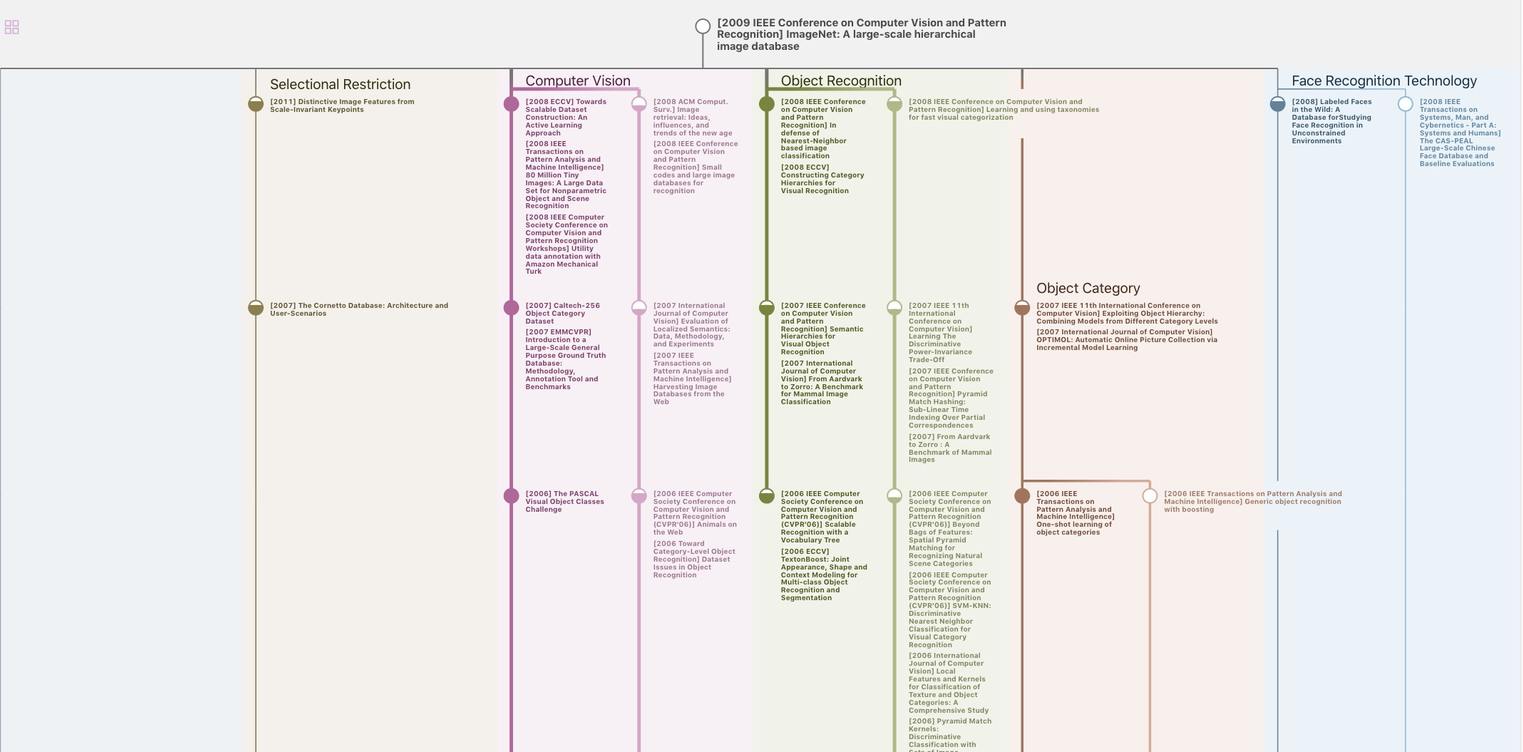
生成溯源树,研究论文发展脉络
Chat Paper
正在生成论文摘要