Exploring the Impact of Phase-Shifted Loading Conditions on Fatigue Life of S355J2 Mild Steel with Different Machine Learning Approaches
The International Journal of Advanced Manufacturing Technology(2024)
摘要
Predicting a component’s fatigue life requires information on not only the number of stress cycles the component will undergo but also the kind and frequency of those stress cycles, as well as information about the surrounding environment and the intended purpose of the component. Models that can forecast lifespan by utilizing available experimental data are preferred since fatigue investigations are costly and time-consuming. Therefore, this work focuses on fatigue testing of S355 mild steel specimens under multiaxial loading conditions involving bending and torsion. The impact of phase-shifted loading conditions on material behavior, considering in-phase and with angles 0°, 45°, and 90°, has been studied. Then, the data of fatigue tests involve variations in loading amplitudes, and configurations used in different ML algorithms such as lazy k-nearest neighbors (Lazy-KNN), linear regression (LR), and random forest (RF) were employed for predictive modeling. These models are evaluated based on their ability to predict nominal stresses, torsion, and bending moments under varying loading configurations. The predictive modeling results are visually presented, showcasing the effectiveness of Lazy-KNN in accurately predicting material responses. Quantitative analyses further confirm the robustness of Lazy-KNN in predicting bending, nominal stress, and torsion under different loading conditions. The study provides valuable insights into the fatigue behavior of S355 mild steel and highlights the significance of considering multiaxial loading configurations in material testing and design.
更多查看译文
关键词
Bending,Fatigue life,Machine learning,Multi axial loading,Torsion
AI 理解论文
溯源树
样例
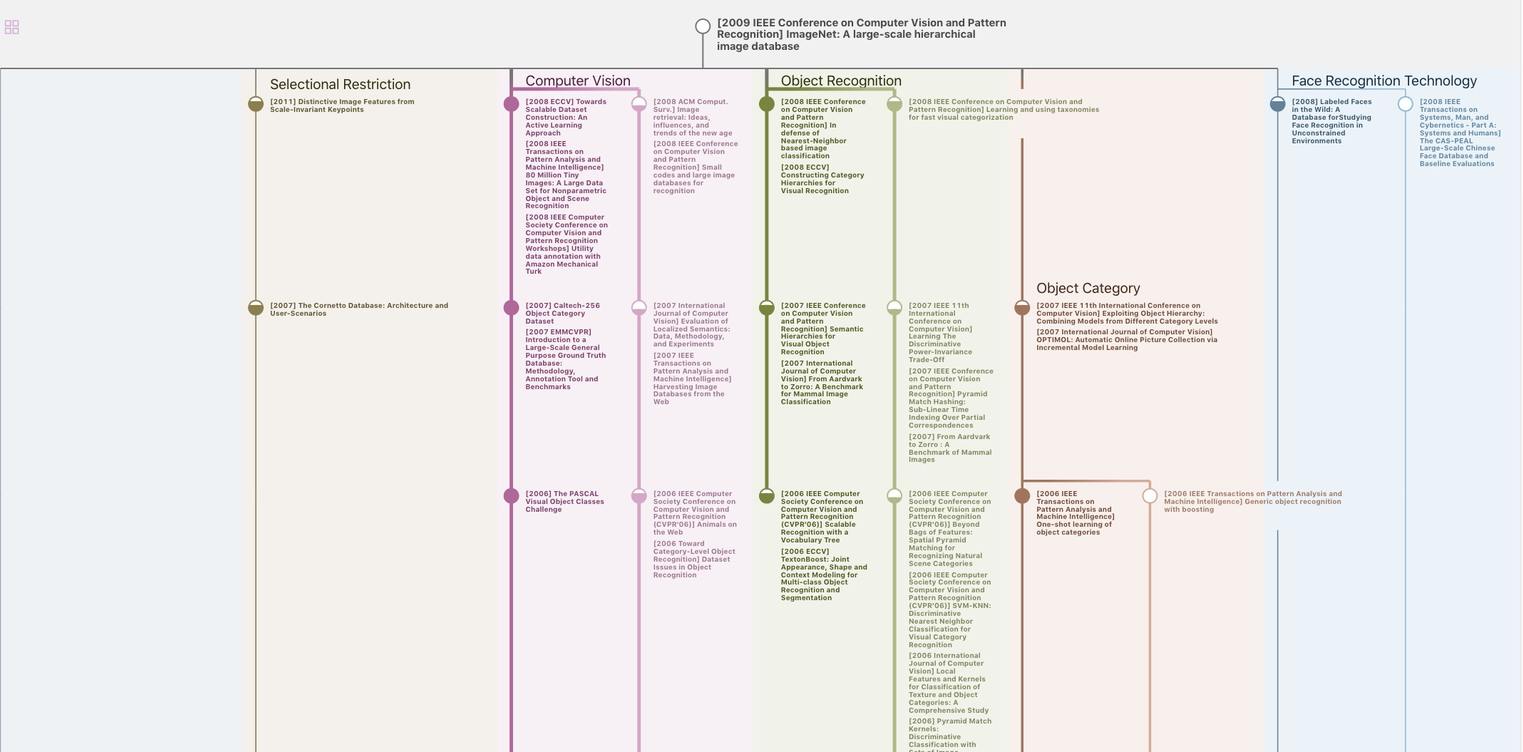
生成溯源树,研究论文发展脉络
Chat Paper
正在生成论文摘要