MadFormer: multi-attention-driven image super-resolution method based on Transformer
Multimedia Systems(2024)
摘要
While the Transformer-based method has demonstrated exceptional performance in low-level visual processing tasks, it has a strong modeling ability only locally, thereby neglecting the importance of spatial feature information and high-frequency details within the channel for super-resolution. To enhance feature information and improve the visual experience, we propose a multi-attention-driven image super-resolution method based on a Transformer network, called MadFormer. Initially, the low-resolution image undergoes an initial convolution operation to extract shallow features while being fed into a residual multi-attention block incorporating channel attention, spatial attention, and self-attention mechanisms. By employing multi-head self-attention, the proposed method aims to capture global–local feature information; channel attention and spatial attention are utilized to effectively capture high-frequency features in both the channel and spatial domains. Subsequently, deep feature information is inputted into a dynamic fusion block that dynamically fuses multi-attention extracted features, facilitating the aggregation of cross-window information. Ultimately, the shallow and deep feature information is fused via convolution operations, yielding high-resolution images through high-quality reconstruction. Comprehensive quantitative and qualitative comparisons with other advanced algorithms demonstrate the substantial advantages of the proposed approach in terms of peak signal-to-noise ratio (PSNR) and structural similarity (SSIM) for image super-resolution.
更多查看译文
关键词
Image super-resolution,Transformer,Multi-attention-driven,Dynamic fusion
AI 理解论文
溯源树
样例
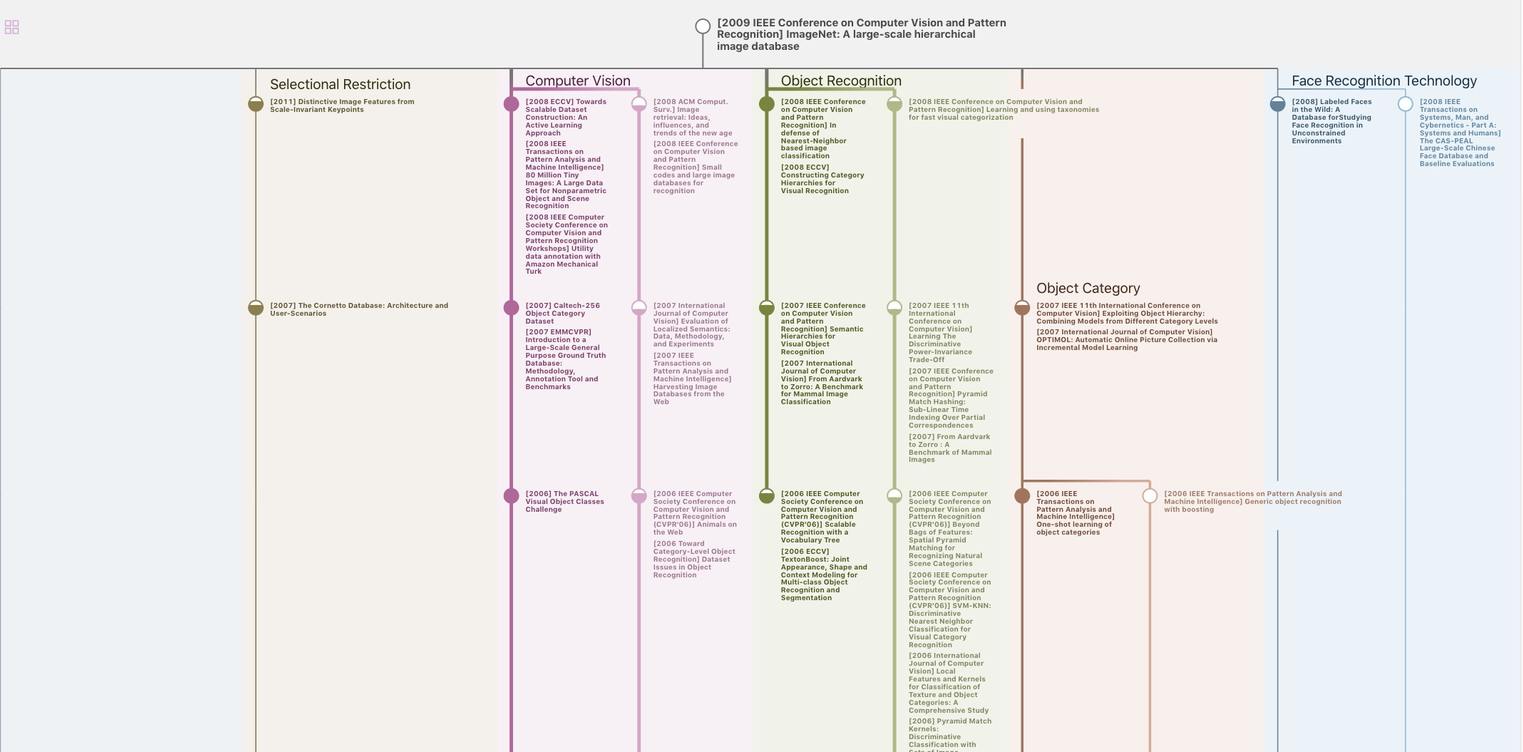
生成溯源树,研究论文发展脉络
Chat Paper
正在生成论文摘要