Pseudo Label Fusion with Uncertainty Estimation for Semi-Supervised Cropping Box Regression
IEEE Transactions on Multimedia(2024)
摘要
Cropping box regression algorithms re-frame the images with predicted cropping boxes for better composition quality, which can save considerable manpower and time for massive image retouching work. Yet, recent learning-based cropping box regression algorithms require expert annotations, which makes the scale of training limited. This consequently incurs a performance bottleneck. To address this issue, previous works seek the help from auxiliary datasets of related tasks,
e.g.
, the composition classification. However, the domain gap between related tasks and the likewise restricted scale of auxiliary datasets are still limiting factors. Hence, our work provides a novel semi-supervised framework that can learn better re-framing knowledge with unlimited unlabeled data. We make use of the unlabeled data via pseudo-labeling, where the model learns from the pseudo labels generated from a temporal ensemble version of itself. To prevent the model learns from its own mistakes,
a.k.a
. the problem of confirmation bias, we propose to rectify the mistakes by fusing multiple candidate pseudo labels into the better ones. The fusion procedure is based on the uncertainty estimation for each boundary of the candidate cropping boxes. The multiple candidates are from the proposed aesthetic region proposal network. Extensive experimental results explain how the uncertainty-based pseudo label fusion procedure overcomes the confirmation bias and demonstrate the superiority of our semi-supervised cropping box regression framework.
更多查看译文
关键词
Image cropping,cropping box regression,semisupervised learning,uncertainty estimation
AI 理解论文
溯源树
样例
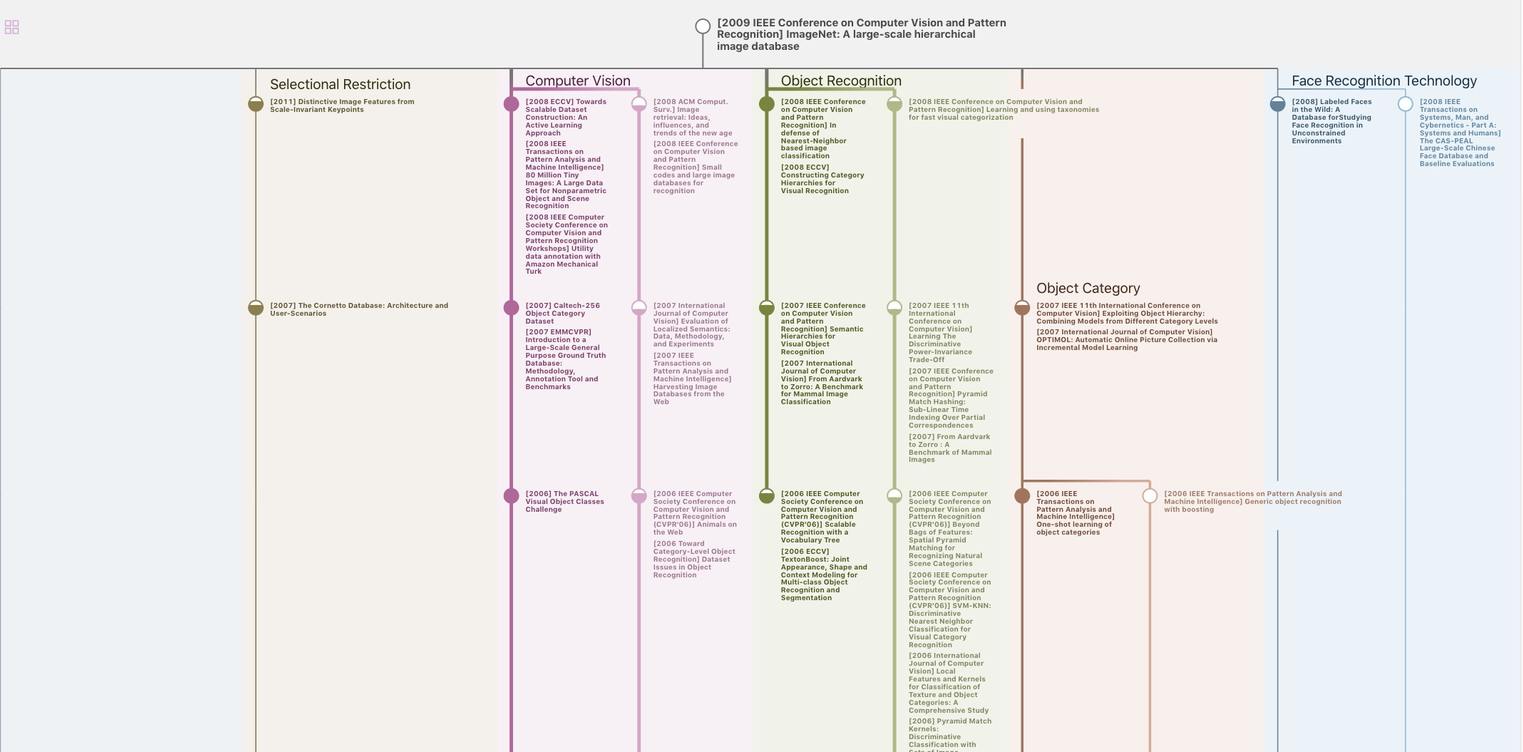
生成溯源树,研究论文发展脉络
Chat Paper
正在生成论文摘要