WLR-Net: An Improved YOLO-V7 With Edge Constraints and Attention Mechanism for Water Leakage Recognition in the Tunnel
IEEE TRANSACTIONS ON EMERGING TOPICS IN COMPUTATIONAL INTELLIGENCE(2024)
摘要
Water leakage recognition plays a significant role in ensuring the safety of shield tunnel lining. However, current models cannot meet the engineering requirements because the tunnel environment is complex. In this concern, a one-stage deep learning model is developed for water leakage recognition. First, we design an attention module to reduce background noise interference. Second, an edge refinement algorithm is proposed to refine the mask of water leakage region. Furthermore, a mixed data augmentation is developed to enhance the robustness of model. Experimental results indicate an average precision (AP) is up to 60%, and a recognition speed is 26 frames per second (FPS). This determines that our proposed network is lightweight and has advantages over peer methods.
更多查看译文
关键词
Tunnel lining,Water leakage region,Deep learning,Instance segmentation
AI 理解论文
溯源树
样例
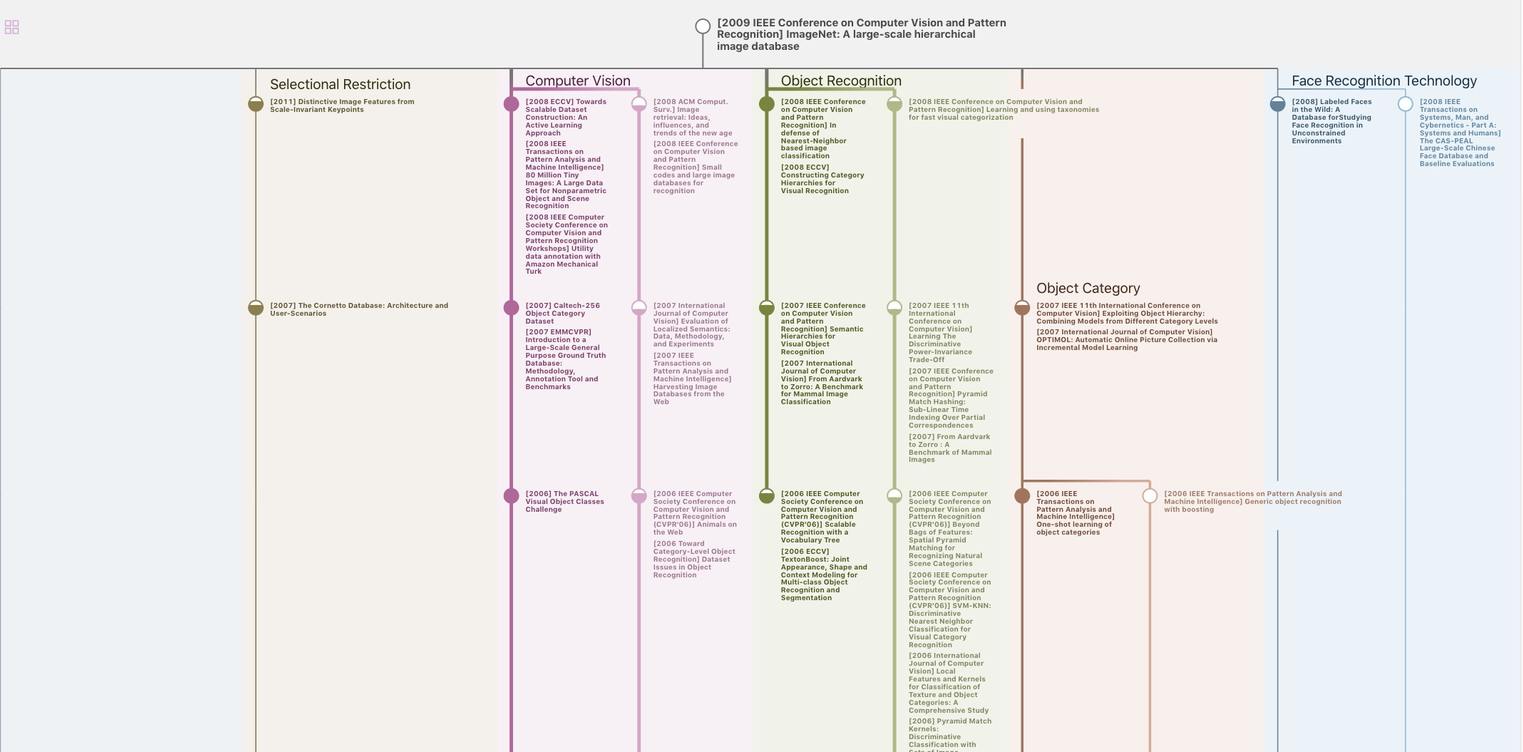
生成溯源树,研究论文发展脉络
Chat Paper
正在生成论文摘要