34.8 A 22nm 16Mb Floating-Point ReRAM Compute-in-Memory Macro with 31.2TFLOPS/W for AI Edge Devices
2024 IEEE International Solid-State Circuits Conference (ISSCC)(2024)
摘要
AI-edge devices demand high-precision computation (e.g. FP16 and BF16) for accurate inference in practical applications, while maintaining high energy efficiency (EF) and low standby power to prolong battery life. Thus, advanced non-volatile AI-edge processors [1, 2] require non-volatile compute-in-memory (nvCIM) [3–5] with a large non-volatile on-chip memory, to store all of the neural network’s parameters (weight data) during power-off, and high-precision high-EF multiply-and-accumulate (MAC) operations during compute, to maximize battery life. Among nvCIMs, ReRAM-nvCIM stands out as a promising candidate due to its lowest cost-per-bit (vs. MRAM, PCM, and eFlash), large on-off ratio, and resilience to magnetic-field interference. However, existing nvCIM macros [3–5] do not support floating-point (FP) computation. Implementing a FP-MAC for nvCIM faces challenges, as shown in Fig. 34.8.1, in (1) balancing the bit width tradeoff for weight pre-alignment between accuracy and storage, (2) addressing long latency and energy consumption in MAC operations due to the high input bit width in FP format, and (3) managing high array current consumption when accessing numerous memory cells (MCs) for FP operations, particularly in the low-resistance-state (LRS) ReRAM cells.
更多查看译文
关键词
Exponent,Weight Data,Non-volatile Memory,Lossless Compression,Line Current,Bit-width,Input Bits,CIFAR-100 Dataset,Network-on-chip,Sign Bit
AI 理解论文
溯源树
样例
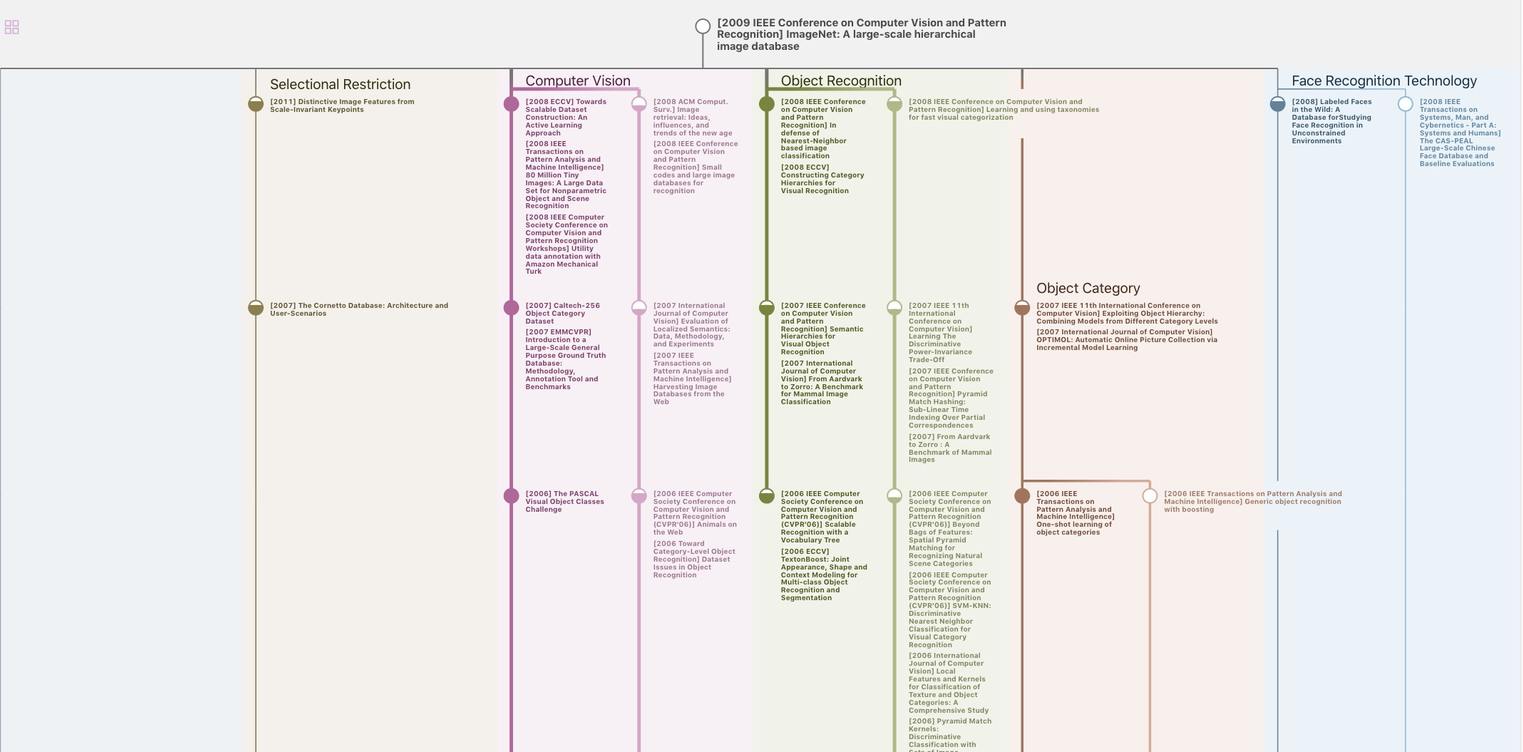
生成溯源树,研究论文发展脉络
Chat Paper
正在生成论文摘要