KnowCoder: Coding Structured Knowledge into LLMs for Universal Information Extraction
Annual Meeting of the Association for Computational Linguistics(2024)
摘要
In this paper, we propose KnowCoder, a Large Language Model (LLM) to conductUniversal Information Extraction (UIE) via code generation. KnowCoder aims todevelop a kind of unified schema representation that LLMs can easily understandand an effective learning framework that encourages LLMs to follow schemas andextract structured knowledge accurately. To achieve these, KnowCoder introducesa code-style schema representation method to uniformly transform differentschemas into Python classes, with which complex schema information, such asconstraints among tasks in UIE, can be captured in an LLM-friendly manner. Wefurther construct a code-style schema library covering over 30,000types of knowledge, which is the largest one for UIE, to the best of ourknowledge. To ease the learning process of LLMs, KnowCoder contains a two-phaselearning framework that enhances its schema understanding ability via codepretraining and its schema following ability via instruction tuning. After codepretraining on around 1.5B automatically constructed data, KnowCoder alreadyattains remarkable generalization ability and achieves relative improvements by49.8% F1, compared to LLaMA2, under the few-shot setting. Afterinstruction tuning, KnowCoder further exhibits strong generalization ability onunseen schemas and achieves up to 12.5% and 21.9%,compared to sota baselines, under the zero-shot setting and the low resourcesetting, respectively. Additionally, based on our unified schemarepresentations, various human-annotated datasets can simultaneously beutilized to refine KnowCoder, which achieves significant improvements up to7.5% under the supervised setting.
更多查看译文
AI 理解论文
溯源树
样例
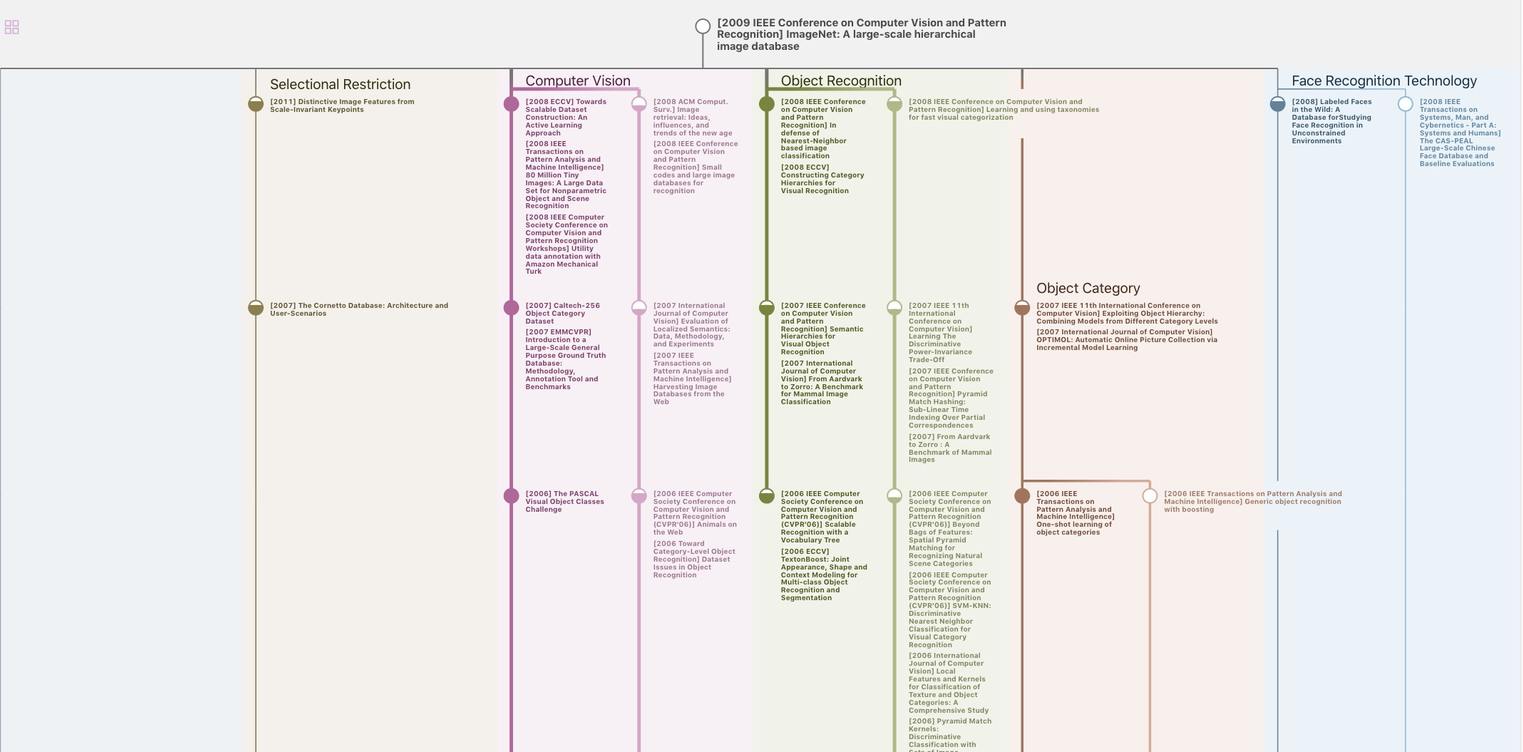
生成溯源树,研究论文发展脉络
Chat Paper
正在生成论文摘要