Controllability of continuous networks and a kernel-based learning approximation
arxiv(2024)
摘要
Residual deep neural networks are formulated as interacting particle systems
leading to a description through neural differential equations, and, in the
case of large input data, through mean-field neural networks. The mean-field
description allows also the recast of the training processes as a
controllability problem for the solution to the mean-field dynamics. We show
theoretical results on the controllability of the linear microscopic and
mean-field dynamics through the Hilbert Uniqueness Method and propose a
computational approach based on kernel learning methods to solve numerically,
and efficiently, the training problem. Further aspects of the structural
properties of the mean-field equation will be reviewed.
更多查看译文
AI 理解论文
溯源树
样例
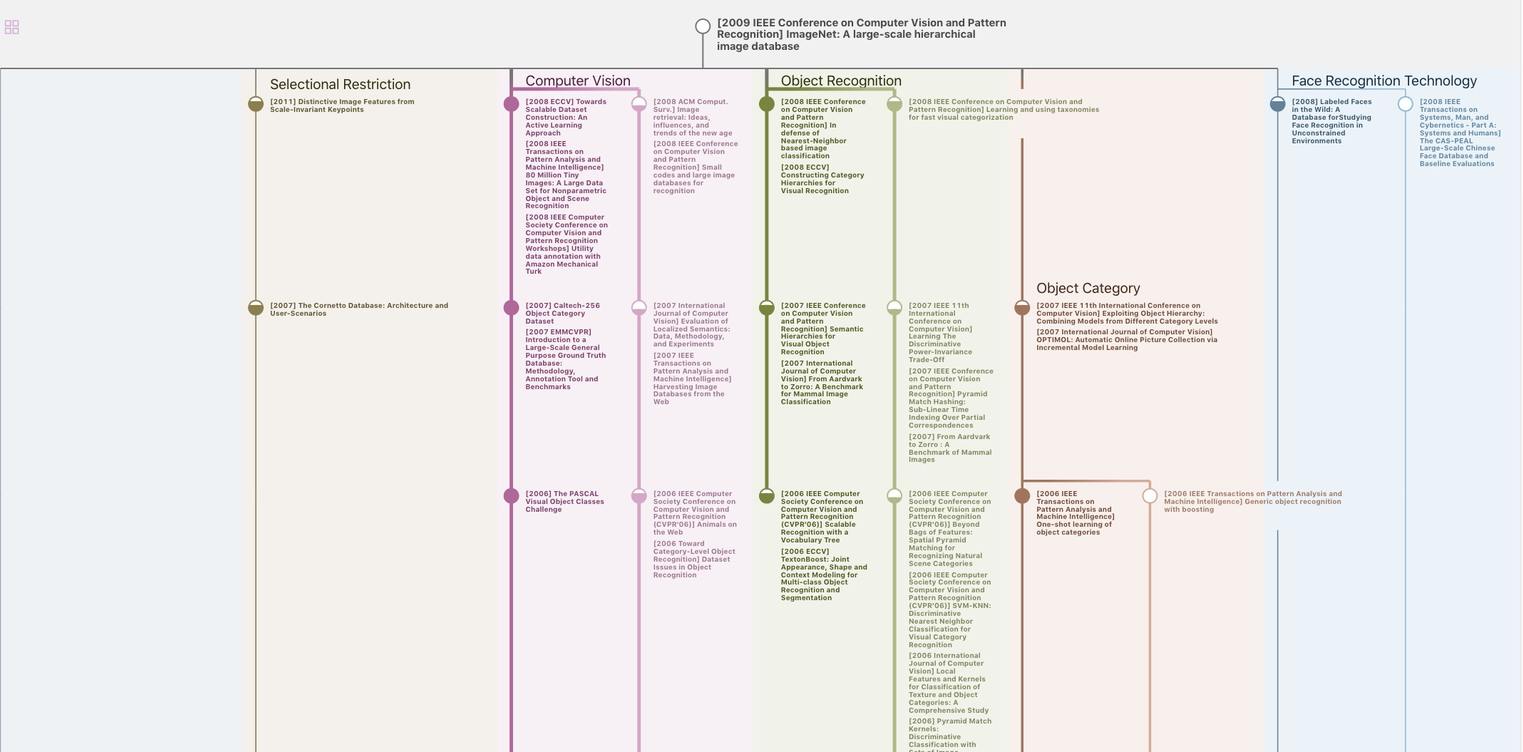
生成溯源树,研究论文发展脉络
Chat Paper
正在生成论文摘要