34.5 A 818-4094TOPS/W Capacitor-Reconfigured CIM Macro for Unified Acceleration of CNNs and Transformers
2024 IEEE International Solid-State Circuits Conference (ISSCC)(2024)
摘要
In the rapidly evolving landscape of machine learning, workloads using diverse neural-network architectures must be covered: including CNNs for image processing, transformers for natural language processing (NLP), and hybrid architectures that blend CNNs and transformers for audio processing. As illustrated in Fig. 34.5.1, these varied architectures have unique computational precision requirements. While CNNs achieve satisfactory accuracy even with low-computational precision, compute SNR or CSNR[1], transformers require higher CSNR to reach their full potential. This diversity amplifies the need for versatile hardware accelerators that can efficiently handle both CNNs and transformers, while meeting the multifaceted demands of modern machine-learning applications.
更多查看译文
关键词
Multilayer Perceptron,Majority Voting,Power Efficiency,Production Inputs,Demand For Applications,Inductive Load,Computational Precision,Hardware Accelerators,Top-1 Accuracy,Hybrid Architecture,Analog Computing,Area Overhead,Vision Transformer,Multilayer Perceptron Layer,Average Noise Level,65-nm CMOS,Power Overhead
AI 理解论文
溯源树
样例
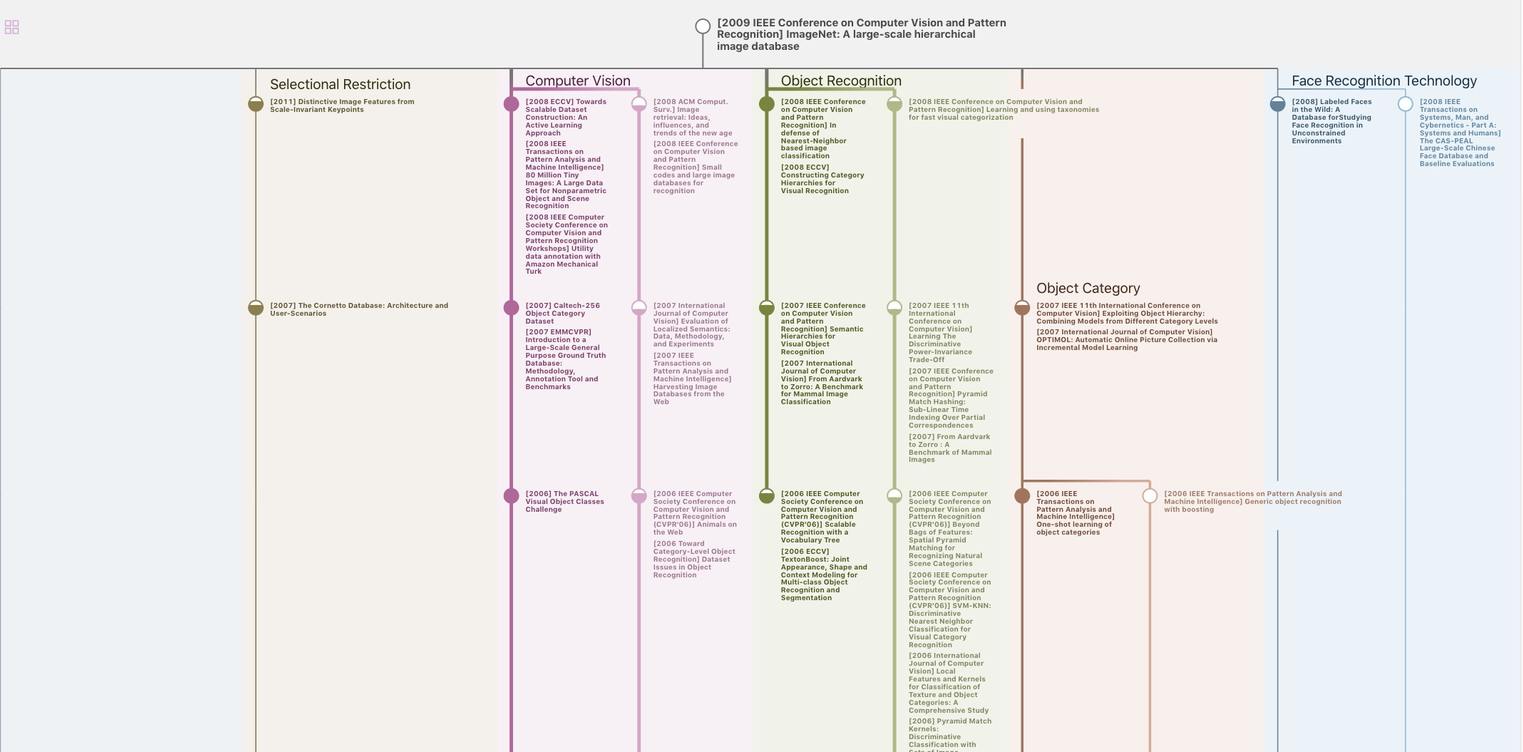
生成溯源树,研究论文发展脉络
Chat Paper
正在生成论文摘要