Multi-center solitary solid pulmonary nodule classification algorithm based on mutual information of graph federation
2023 5th International Conference on Frontiers Technology of Information and Computer (ICFTIC)(2023)
摘要
With the continuous growth of medical image data and the imbalance in distribution across multiple medical centers, accurate and reliable classification of Solitary solid pulmonary nodules has become increasingly important. Firstly, to address the issue of data silos, a federated learning framework is employed, which ensures data security while improving the diagnostic performance of the model. Secondly, to tackle the non-independent and identically distributed (non-IID) nature of multi-center data, a graph federated personalized strategy based on mutual information is proposed. Graph convolutional algorithms and mutual information are utilized to explore the topological structure and correlation information among the data for personalized modeling. Additionally, considering the variations in shape and size of pulmonary nodules in different CT images, this paper combines a Diverse Branch Block (DBB) convolutional structure with ResNet18, serving as the framework for local and global models, to enhance the model’s capability of extracting multi-scale features. The results demonstrate promising classification performance of the proposed algorithm across five pulmonary nodule data centers, with AUC values of 0.7572, 0.8378, 0.7616, 0.7813, and 0.9130 on the respective test sets.
更多查看译文
关键词
Personalized Federated Learning,Mutual Information,Diverse Branch Block,Graph Convolution,Solitary solid pulmonary Nodule Classification
AI 理解论文
溯源树
样例
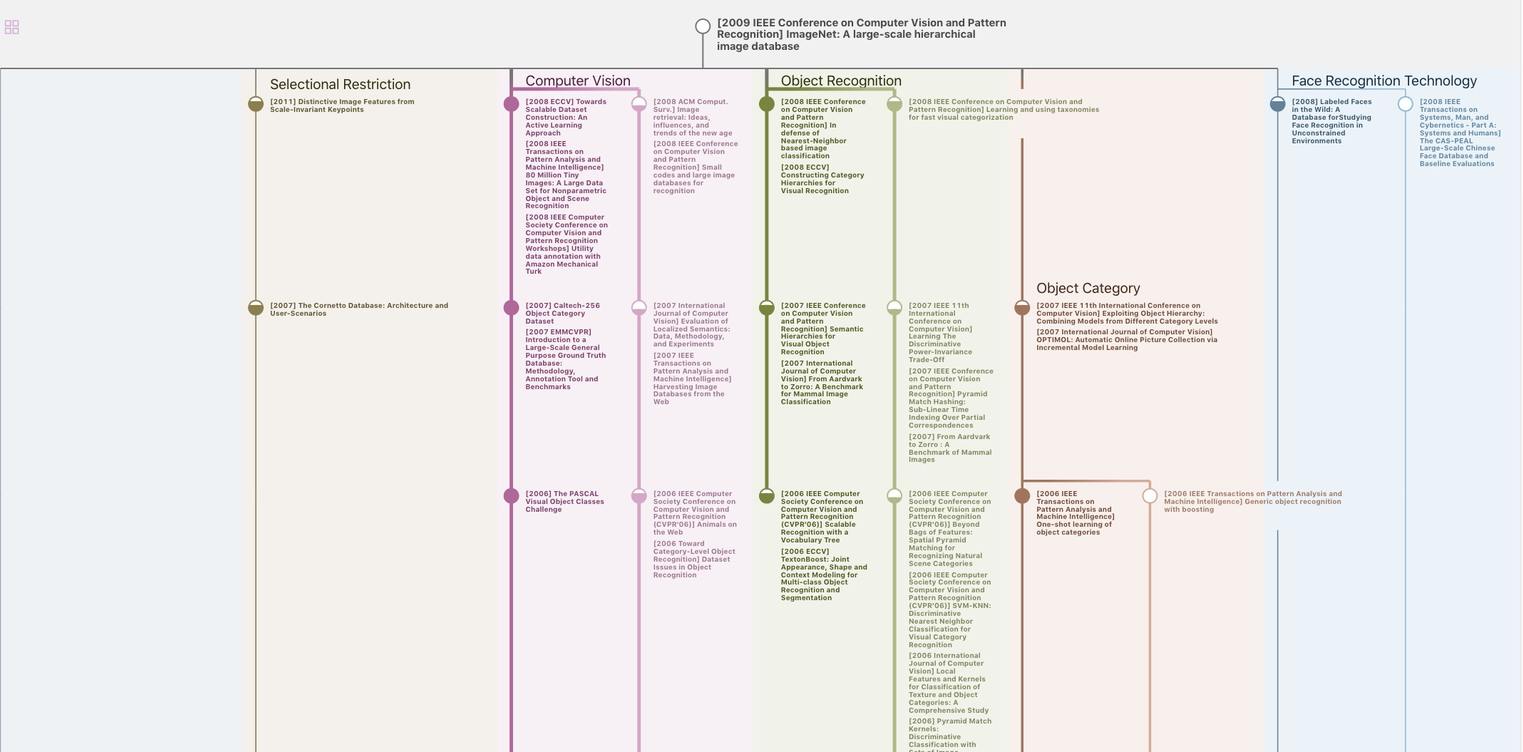
生成溯源树,研究论文发展脉络
Chat Paper
正在生成论文摘要