34.6 A 28nm 72.12TFLOPS/W Hybrid-Domain Outer-Product Based Floating-Point SRAM Computing-in-Memory Macro with Logarithm Bit-Width Residual ADC
2024 IEEE International Solid-State Circuits Conference (ISSCC)(2024)
摘要
SRAM-based computing-in-memory (CIM) is considered crucial to achieving high-energy efficiency (EF) for artificial-intelligence (AI) applications on edge devices. Researchers are currently exploring floating-point (FP) CIM [1], [2], as integer (INT) precision CIMs [3] –[6] are no longer sufficient for new AI applications, which demand increased accuracy, complexity, and on-chip training. However, both analog and digital FP-CIMs face several significant challenges in realizing FP calculations, due to difficulties associated with handling high-bit precision: including (1) effectively combining the advantages of analog and digital CIMs while mitigating their respective drawbacks for high-bit-precision processing; (2) achieving optimal design trade-off for an analog-digital converter (ADC) necessitates the simultaneous consideration of bit precision, throughput, and overhead; (3) addressing the need for large fan-in multi-level adder trees in inner-based CIMs to sum high-bit-precision partial products, which can adversely impact overall EF, as shown in Fig. 34.6.1.
更多查看译文
关键词
Throughput,Image Stacks,Partial Products,Artificial Intelligence Applications,Control Circuit,Input Current,Partial Sums,Edge Devices,Simultaneous Consideration,Analog Domain,CIFAR-100 Dataset,Word Line,Current Mirror
AI 理解论文
溯源树
样例
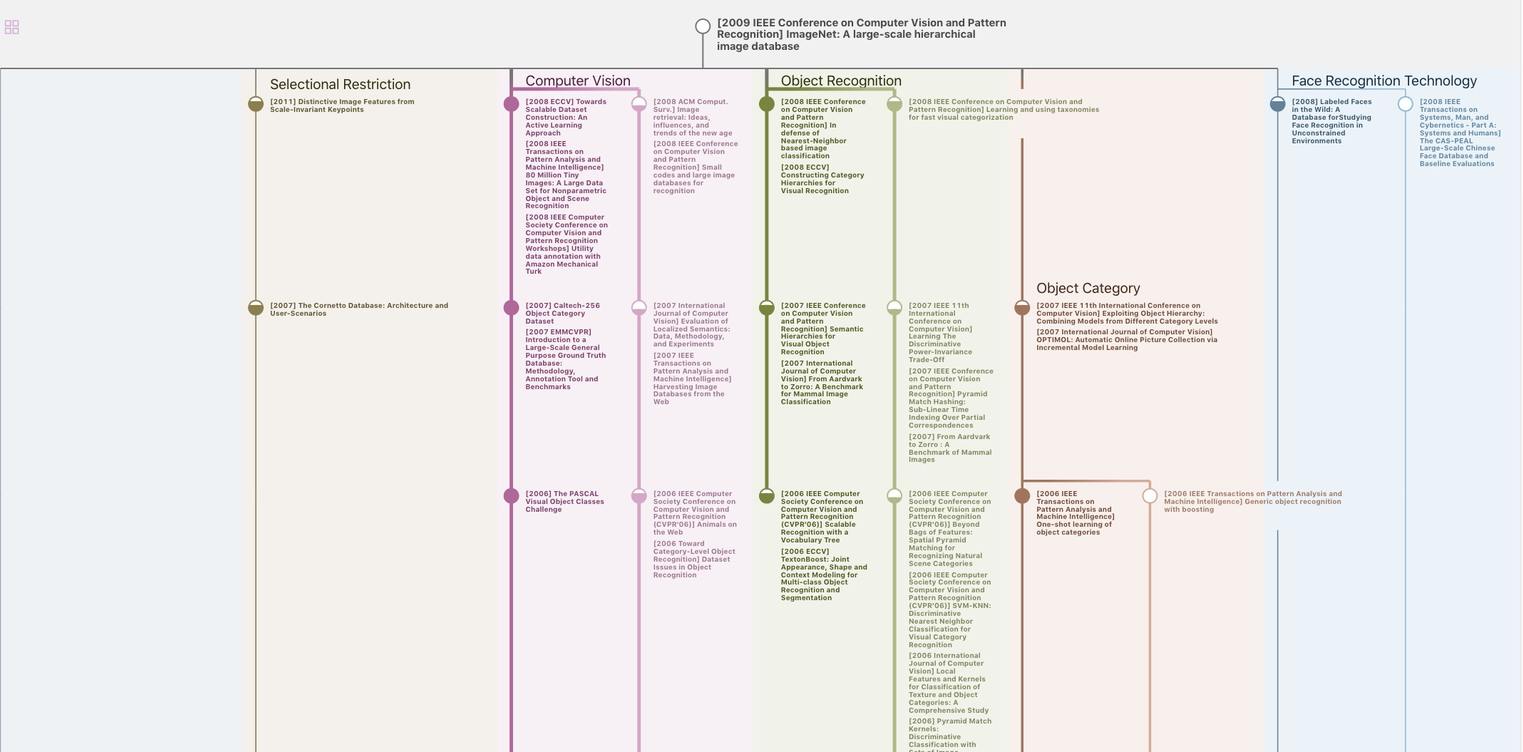
生成溯源树,研究论文发展脉络
Chat Paper
正在生成论文摘要