Impact of AI-Based Post-Processing on Image Quality of Non-Contrast Computed Tomography of the Chest and Abdomen
DIAGNOSTICS(2024)
摘要
Non-contrast computed tomography (CT) is commonly used for the evaluation of various pathologies including pulmonary infections or urolithiasis but, especially in low-dose protocols, image quality is reduced. To improve this, deep learning-based post-processing approaches are being developed. Therefore, we aimed to compare the objective and subjective image quality of different reconstruction techniques and a deep learning-based software on non-contrast chest and low-dose abdominal CTs. In this retrospective study, non-contrast chest CTs of patients suspected of COVID-19 pneumonia and low-dose abdominal CTs suspected of urolithiasis were analysed. All images were reconstructed using filtered back-projection (FBP) and were post-processed using an artificial intelligence (AI)-based commercial software (PixelShine (PS)). Additional iterative reconstruction (IR) was performed for abdominal CTs. Objective and subjective image quality were evaluated. AI-based post-processing led to an overall significant noise reduction independent of the protocol (chest or abdomen) while maintaining image information (max. difference in SNR 2.59 +/- 2.9 and CNR 15.92 +/- 8.9, p < 0.001). Post-processing of FBP-reconstructed abdominal images was even superior to IR alone (max. difference in SNR 0.76 +/- 0.5, p <= 0.001). Subjective assessments verified these results, partly suggesting benefits, especially in soft-tissue imaging (p < 0.001). All in all, the deep learning-based denoising-which was non-inferior to IR-offers an opportunity for image quality improvement especially in institutions using older scanners without IR availability. Further studies are necessary to evaluate potential effects on dose reduction benefits.
更多查看译文
关键词
computed tomography,image reconstruction,image quality,chest,abdomen,urolithiasis,COVID-19,deep learning
AI 理解论文
溯源树
样例
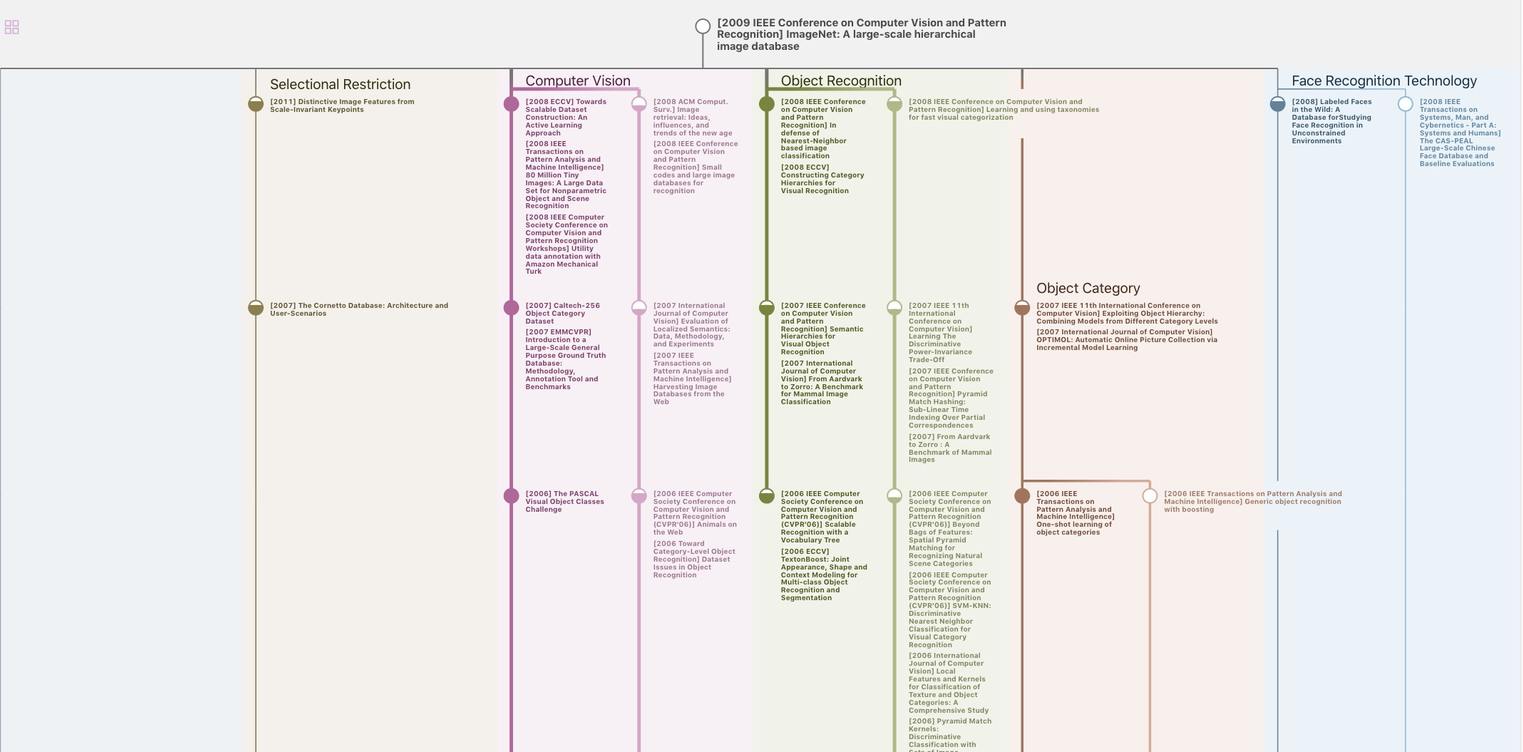
生成溯源树,研究论文发展脉络
Chat Paper
正在生成论文摘要