Optimal Experimental Design for Big Data: Applications in Brain Imaging
biorxiv(2019)
摘要
The cost of data collection and processing is becoming prohibitively expensive for many research groups across disciplines, a problem that is exacerbated by the dependence of ever larger sample sizes to obtain reliable inferences for increasingly subtle questions. And yet, as more data is available and open access, more researchers desire to analyze it for different questions, often including previously unforeseen questions. To further increase sample sizes, existing datasets are often amalgamated. These —datasets that serve to answer many disparate questions for different individuals—are increasingly common and important. efficiently and flexibly analyze on all the datasets. How can one optimally design these reference datasets and pipelines to yield derivative data that are simultaneously useful for many different tasks? We propose an approach to experimental design that leverages multiple measurements for each distinct item (for example, an individual). The key insight is that each measurement of the same item should be more similar to other measurements of that item, as compared to measurements of any other item. In other words, we seek to optimally one item from another. We formalize the notion of discriminability, and introduce both a non-parameteric and parametric statistic to quantify the discriminability of potentially multivariate or non-Euclidean datasets. With this notion, one can make optimal decisions—either with regard to acquisition or analysis of data—by maximizing discriminability. Crucially, this optimization can be performed in the absence of any task-specific (or supervised) information. We show that optimizing decisions with respect to discriminability yields improved performance on subsequent inference tasks. We apply this strategy to a brain imaging dataset built by the “Consortium for Reliability and Reproducability” which consists of 24 disparate magnetic resonance imaging datasets, each with up to hundreds of individuals that were imaged multiple times. We show that by optimizing pipelines with respect to discriminability, we improve performance on multiple subsequent inference tasks, even though discriminability does not consider the tasks whatsoever.
更多查看译文
AI 理解论文
溯源树
样例
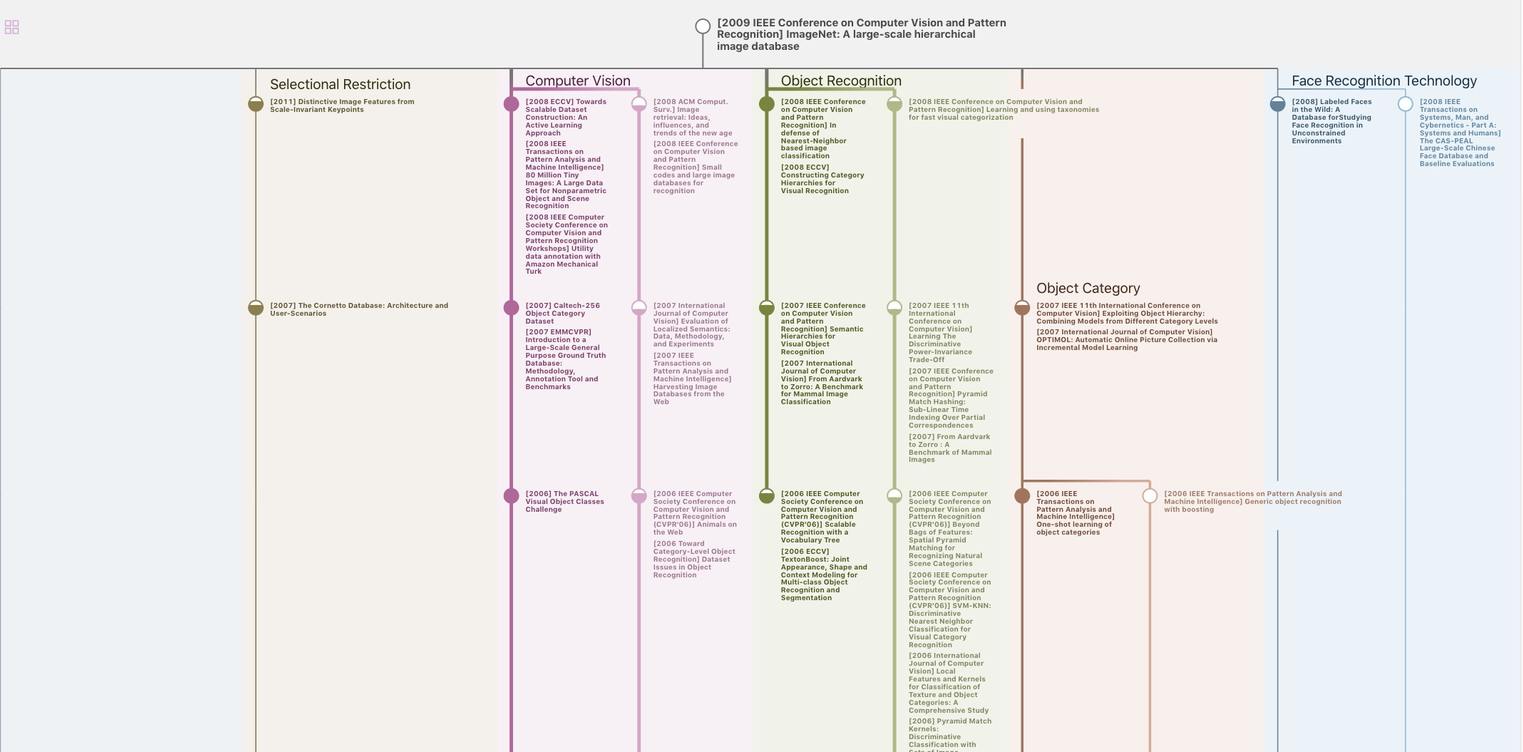
生成溯源树,研究论文发展脉络
Chat Paper
正在生成论文摘要