A separable privacy-preserving technique based on reversible medical data hiding in plaintext encrypted images using neural network
Multimedia Tools and Applications(2024)
摘要
The Internet of Medical Things (IoMT) and cloud storage play pivotal roles in supporting diagnosis, treatment processes, and healthcare. However, they also present significant threats to patient privacy and security. Safeguarding patient privacy has become an urgent practical concern. This paper introduces a privacy-preserving technique based on reversible medical data hiding in plaintext encrypted images using a neural network. Our approach starts by constructing a unified neural network model that integrates U-Net and AlexNet for precise classification and segmentation tasks. Subsequently, we employ plaintext encryption to conceal the region of interest (ROI), preventing visual disclosure of patient privacy while avoiding unwarranted attention from ciphertext encryption. Through a skipping hiding method, we encrypt and embed privacy information into the region of non-interest (RONI). Notably, the proposed scheme is separable, enhancing flexibility and security. For content owners, a joint neural network model divides the medical image into ROI and RONI, with ROI encrypted using an improved plaintext encryption method. Data hiders embed secret bits into RONI based on skipping hiding without accessing any original image information. Receivers can obtain desired information based on specific authority. Importantly, our scheme effectively addresses the challenge of separately storing medical images and diagnostic information, thereby reducing the risk of loss or mismatch. It mitigates the risk of privacy disclosure in diagnostic information without compromising ROI image quality. Experimental results demonstrate the superior accuracy of our scheme (accuracy, precision, recall, and F1-score all exceeded 0.98, with a remarkable recall rate of 0.9922), along with enhanced visual quality, robust plaintext encryption (plaintext-encrypted stego-image: average PSNR: 43dB, SSIM: 0.99, NC: 0.99), high efficiency and low complexity (overall processing time of 3.19 s) and complete reversibility (restored image: PSNR: +∞dB, SSIM: 1, NC: 1). Additionally, our scheme proves effective in addressing current privacy protection challenges.
更多查看译文
关键词
Plaintext encryption,Reversible medical data hiding,Joint neural network,Skipping hiding
AI 理解论文
溯源树
样例
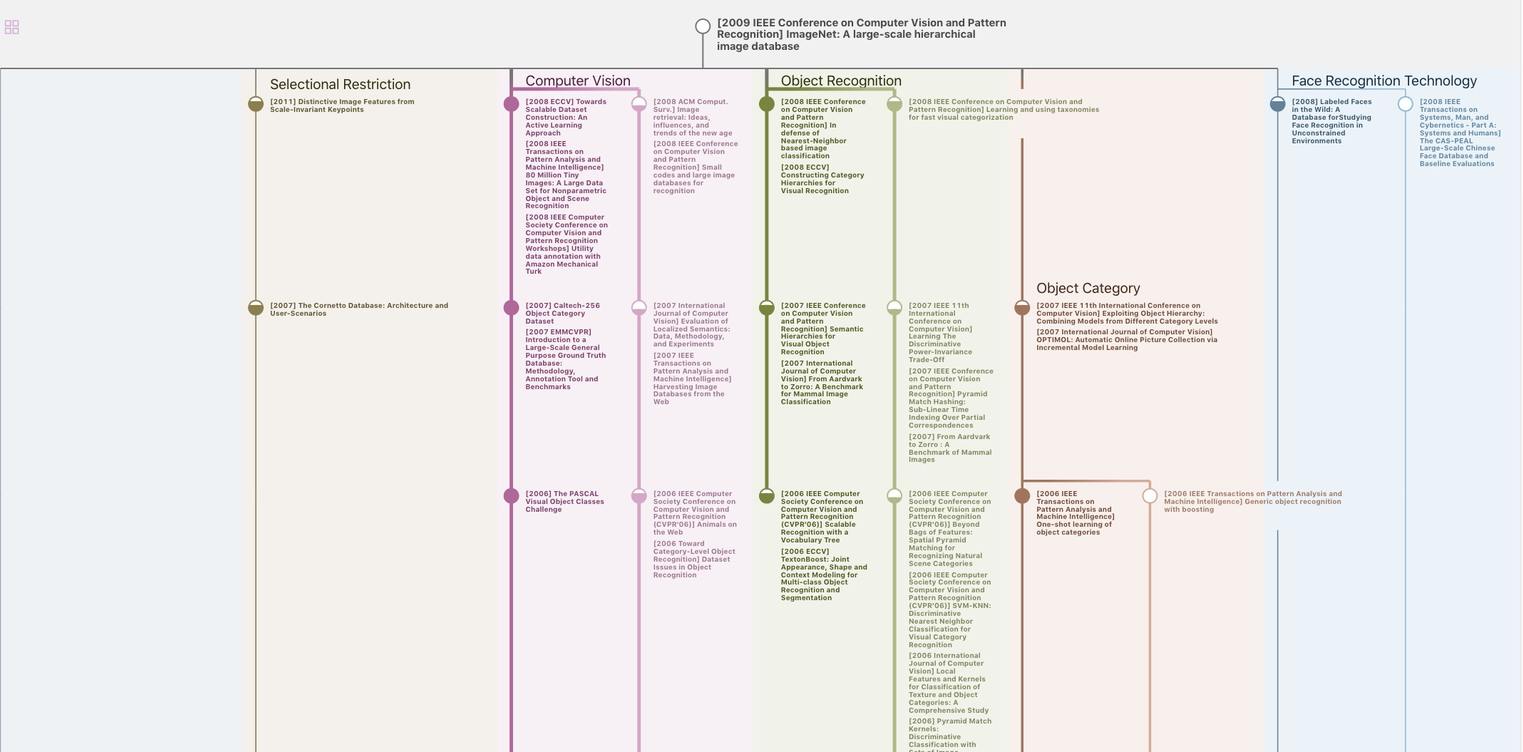
生成溯源树,研究论文发展脉络
Chat Paper
正在生成论文摘要