Nestability: A Deep Learning Oracle for Nesting Scrap Prediction in Manufacturing Industry
Resources, conservation and recycling(2024)
摘要
In the quest for sustainable manufacturing, a critical yet often overlooked aspect is predicting the scrap in nesting-based manufacturing. This aspect is pivotal for its eco-friendly implications and potential to enhance cost-efficiency and competitiveness in the manufacturing industry. This paper introduces a method to predict scrap generation in nesting-based manufacturing solely based on part geometries without prior knowledge of the resulting arrangement. Our method employs three deep learning modules: one for encoding geometries, another to condense these into an information vector reflecting interdependence for scrap prediction, and a third for the actual prediction using the prior modules. Evaluated on a private real-world sheet metal dataset, the technique achieves a Mean Absolute Percentage Error (MAPE) of 24.8%, showcasing its precision in scrap prediction. Such predictions can bolster sustainable manufacturing by curtailing material waste, improving production efficiency, and enhancing eco-sustainability. The paper underscores scrap prediction's role in advancing sustainable manufacturing in nesting-based industries.
更多查看译文
关键词
Scrap prediction,Sustainable production,Artificial intelligence,Nesting-based manufacturing,Neural networks,Autoencoder
AI 理解论文
溯源树
样例
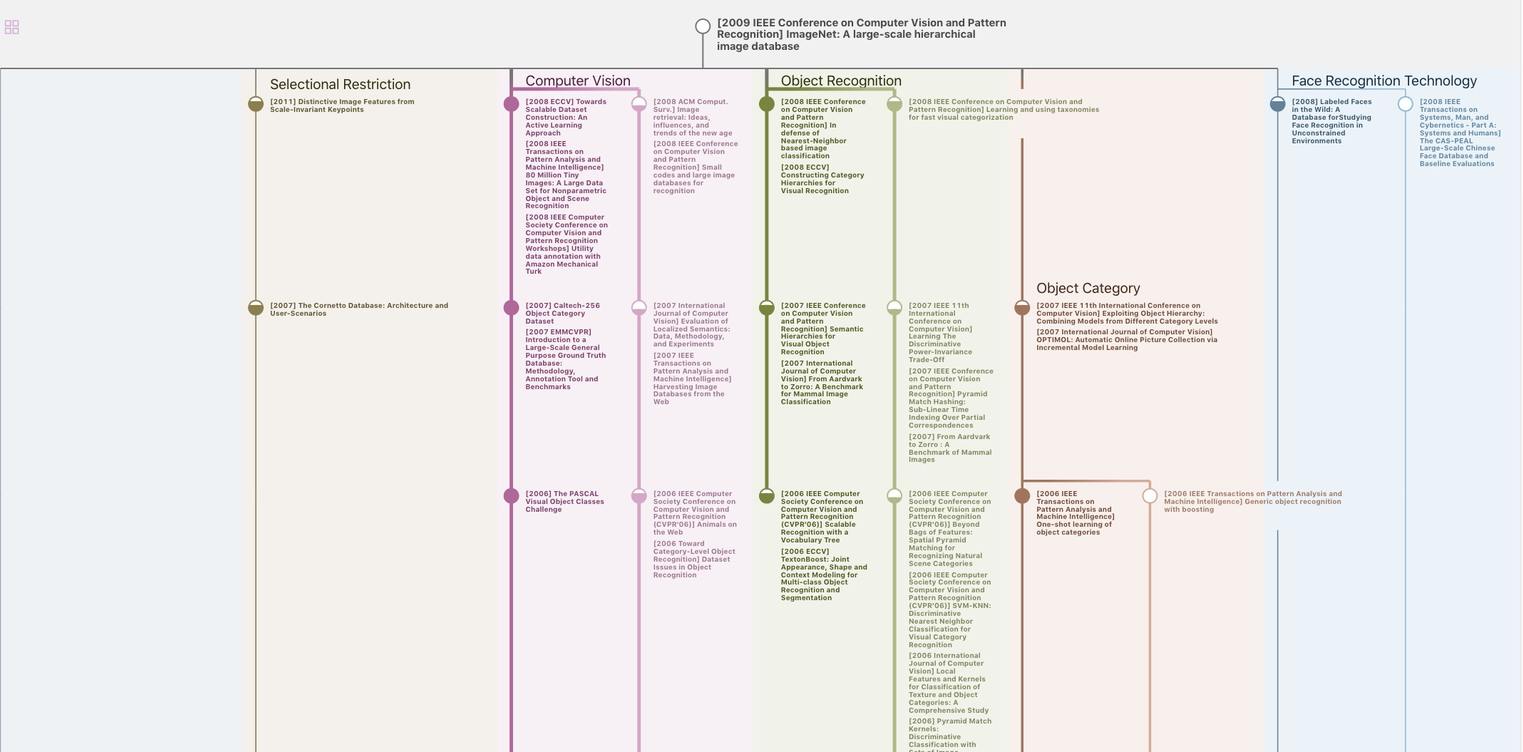
生成溯源树,研究论文发展脉络
Chat Paper
正在生成论文摘要