Deep-Learning-Guided Evaluation Method for the High-Volume Preparation of Flexible Sensors Based on Inkjet Printing
ACS APPLIED MATERIALS & INTERFACES(2024)
摘要
Flexible sensors for application in various industries, including biomedicine and wearable electronics, are frequently made using silver nanoparticle (AgNP) inks and inkjet printing (IJP) technology. Inkjet-printed flexible electronic devices are made up of many printed lines that run parallel to each other, and the surface morphology of the printed lines and the interline state directly impact the electrical conductivity of the electronic devices. This paper describes the experimental setup for IJP, the definition of print line characteristics, and common unavoidable defects. Conductivity and physical defects are considered in defining the print line quality assessment. In addition, two prediction models of flexible sensors before batch printing and a model for detecting defects after printing are provided. The predictive models can guide actions, leading to a print success rate of over 80%. We build the defect detection model using a neural architecture search because manually fine-tuning neural networks for reference is challenging. Finally, a target detection model with a mAP@0.5 of 81.2% is built in just 0.77 graphics processing unit (GPU) days. The model takes only 4.6 ms to detect an image, satisfying the real-time monitoring needs. At the same time, an accuracy of 95.5% can be achieved in the test data set. This work provides a new idea for the high-volume preparation of flexible sensors.
更多查看译文
关键词
flexible sensor,inkjet printing,defect detection,neural architecture search,convolutional neural networks,printing quality optimization
AI 理解论文
溯源树
样例
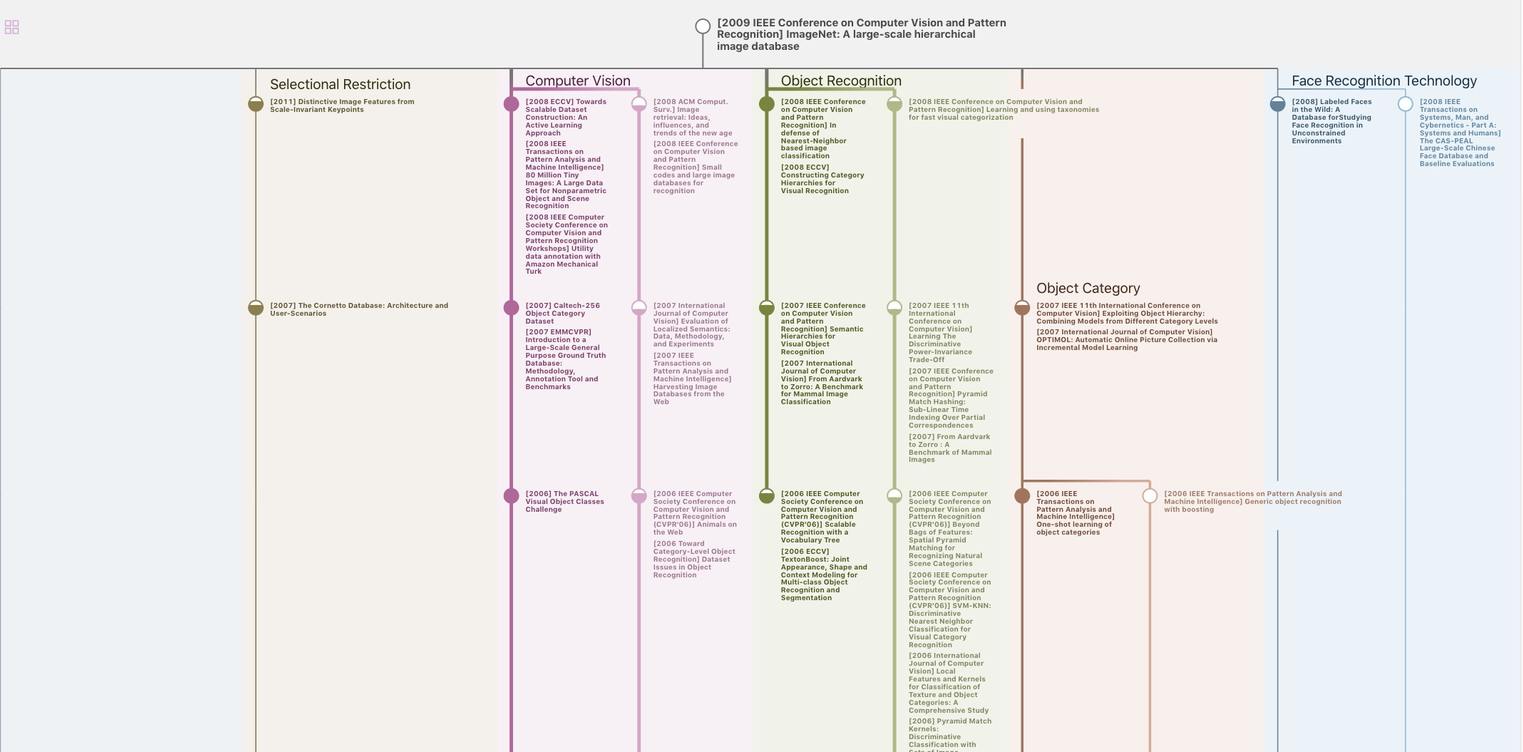
生成溯源树,研究论文发展脉络
Chat Paper
正在生成论文摘要