Enhancing deforestation monitoring in the Brazilian Amazon: A semi-automatic approach leveraging uncertainty estimation
ISPRS Journal of Photogrammetry and Remote Sensing(2024)
摘要
Official governmental monitoring of deforestation in the Brazilian Amazon relies on human experts conducting visual analyzes of remote sensing images, an approach that is very expensive and time-consuming due to the enormous geographic extent to be inspected. The main obstacle to the adoption of fully automatic methods is the requirement for the highest possible deforestation detection accuracy, which arises from the official nature of the deforestation reports, used as a basis for deforestation prevention policies and international treaties. This work addresses such a high accuracy constraint by introducing a semi-automatic method that aims at limiting visual analysis to areas where automatic classification is characterized by high uncertainty. We hypothesize that classification uncertainty is correlated with classification error. Therefore, by trusting automatic detections with low uncertainty and subjecting areas of high uncertainty to visual audit, the amount of human intervention in the whole process could be limited to a small fraction of the forest area, while very high accuracies would be achieved. In this work we investigate various uncertainty estimation strategies, including multiple and single inference approaches, and evaluate different uncertainty metrics. Additionally, we conducted a comprehensive analysis to identify the natural factors and deforestation practices that contribute to high uncertainty in automatic classification. We draw interesting conclusions from the analysis of selected regions of two distinct target sites, which exemplify common causes of uncertainty and indicate how uncertainty may be related with ongoing forest degradation processes. Through experimental evaluation at those sites, we demonstrate that the proposed semi-automatic methodology can achieve impressive accuracies in terms of F1-score values, exceeding 97%, while reducing the total human audit workload to just 3% of the target area.
更多查看译文
关键词
Uncertainty estimation,Deforestation detection,Fully convolutional networks,Brazilian Amazon,Deep learning,Remote sensing
AI 理解论文
溯源树
样例
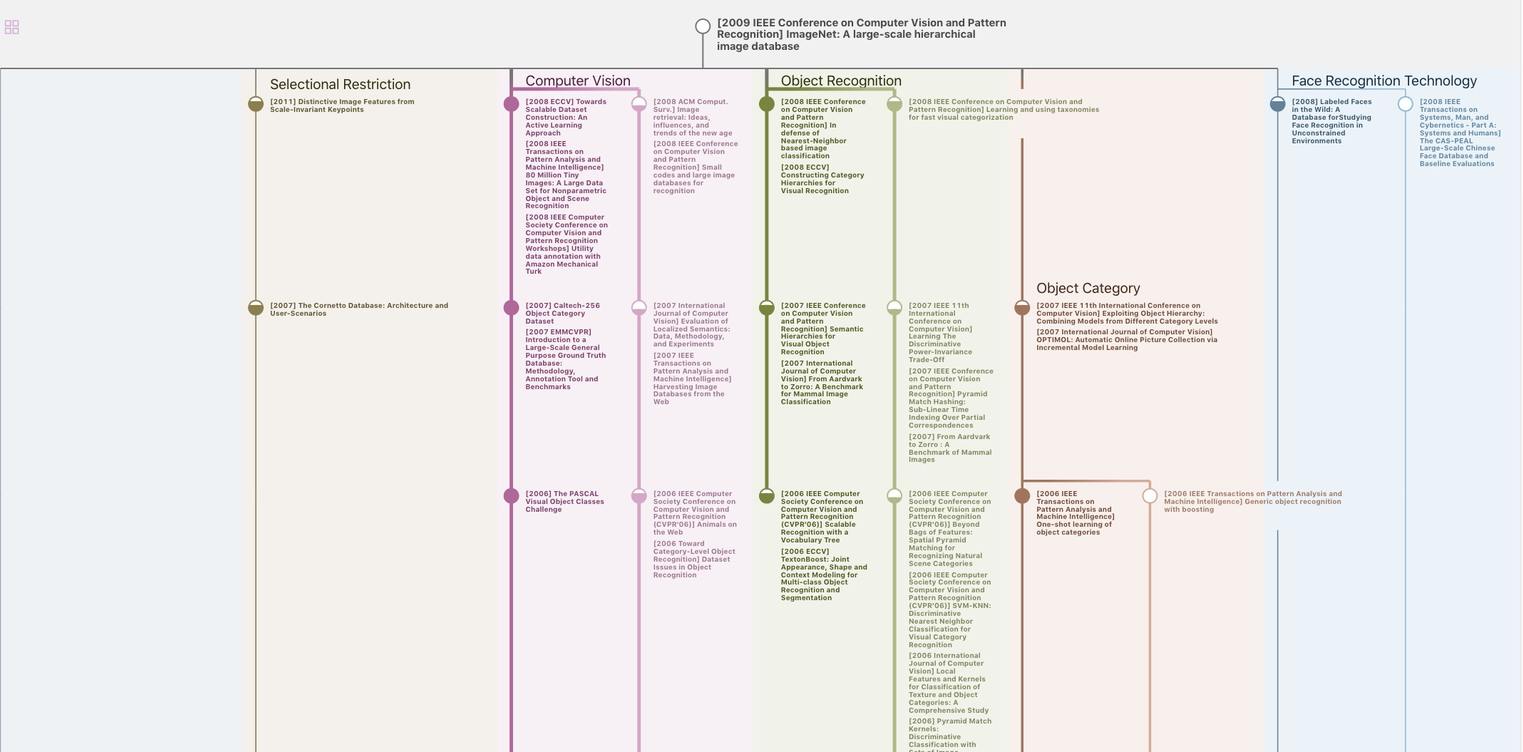
生成溯源树,研究论文发展脉络
Chat Paper
正在生成论文摘要