An iDCA with Sieving Strategy for PDE-Constrained Optimization Problems with L^1-2 -Control Cost
Journal of Scientific Computing(2024)
摘要
In this paper, sparse elliptic PDE-constrained optimization problems with L^1-2 -control cost ( L^1-2 -EOCP) are considered. To induce control sparsity, traditional finite element models usually use L^1 -control cost to induce sparsity, and in practical problems, many non-convex regularization terms are more capable of inducing sparsity than convex regularization terms, for example, in finite-dimensional problems, the sparsity of l_1-2 -norm induced solutions is stronger than l_1 -norm. Inspired by the finite-dimensional problems, we extend the L^1-2 -regularization technique to infinite-dimensional elliptic PDE-constrained optimization problems. Unlike finite-dimensional problems where the l_1-2 -norm is greater than or equal to 0, the conclusion does not hold for the L^1-2 -control cost in the infinite-dimensional sense. To overcome these difficulties, an inexact difference of convex functions algorithm with sieving strategy (s-iDCA) is proposed for solving L^1-2 -EOCP where the corresponding subproblems are solved by an inexact heterogeneous alternating direction method of multipliers (ihADMM) algorithm. Furthermore, by using the particular structure of the L^1-2 -EOCP and constructing a new energy function and using its KŁ property, the global convergence results of the DCA algorithm are given. Numerical experiments show that our proposed s-iDCA algorithm is effective and that the model with the L^1-2 -regularization term is stronger than the L^1 -regularization term in terms of the sparsity of the induced solutions.
更多查看译文
关键词
PDE-constrained optimization problems,-norm,Sparsity,S-iDCA,KŁ property,49J20,49N05,65G99,68W15
AI 理解论文
溯源树
样例
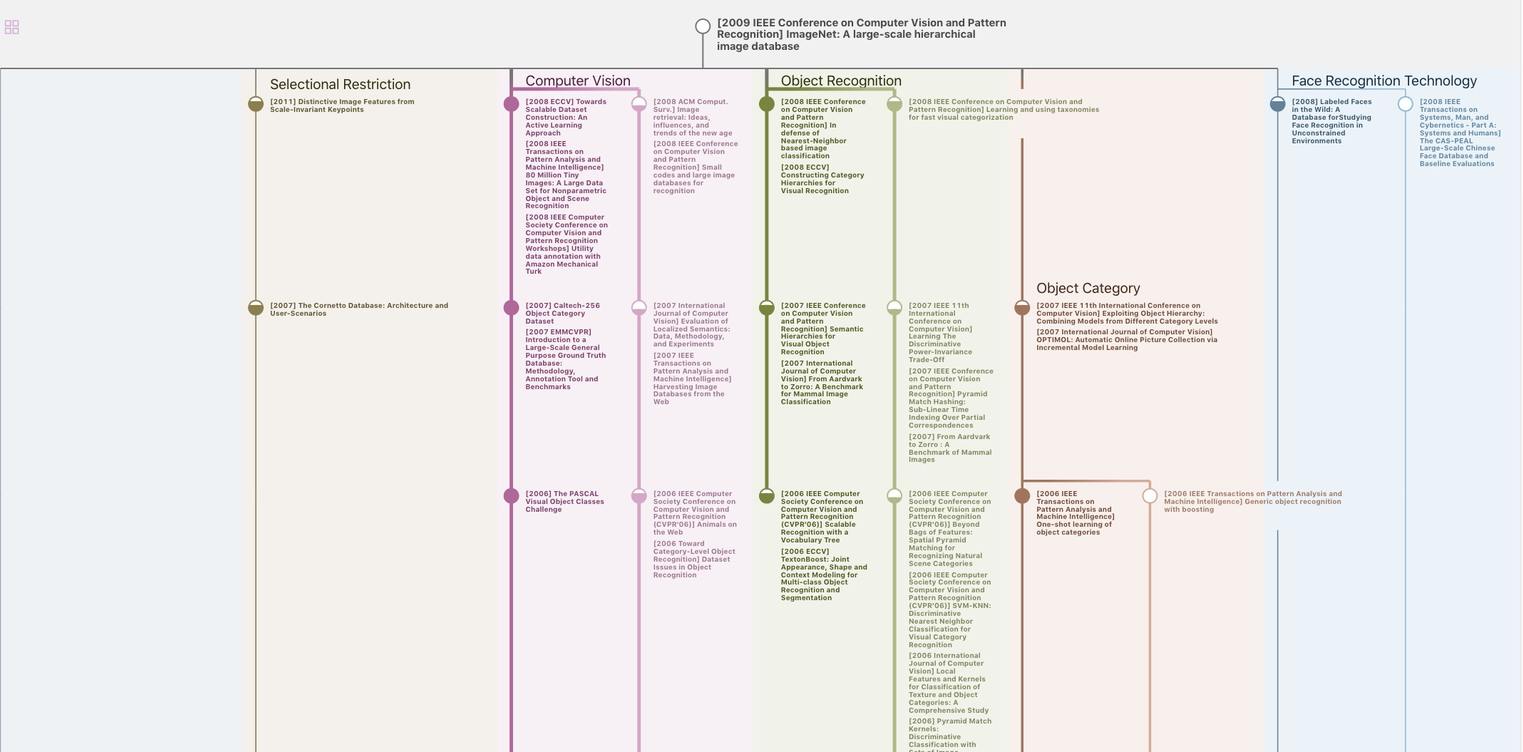
生成溯源树,研究论文发展脉络
Chat Paper
正在生成论文摘要