Measuring Item Fairness in Next Basket Recommendation: A Reproducibility Study
Lecture notes in computer science(2024)
摘要
Item fairness of recommender systems aims to evaluate whether items receive a fair share of exposure according to different definitions of fairness. Raj and Ekstrand [26] study multiple fairness metrics under a common evaluation framework and test their sensitivity with respect to various configurations. They find that fairness metrics show varying degrees of sensitivity towards position weighting models and parameter settings under different information access systems. Although their study considers various domains and datasets, their findings do not necessarily generalize to next basket recommendation (NBR) where users exhibit a more repeat-oriented behavior compared to other recommendation domains. This paper investigates fairness metrics in the NBR domain under a unified experimental setup. Specifically, we directly evaluate the item fairness of various NBR methods. These fairness metrics rank NBR methods in different orders, while most of the metrics agree that repeat-biased methods are fairer than explore-biased ones. Furthermore, we study the effect of unique characteristics of the NBR task on the sensitivity of the metrics, including the basket size, position weighting models, and user repeat behavior. Unlike the findings in [26], Inequity of Amortized Attention (IAA) is the most sensitive metric, as observed in multiple experiments. Our experiments lead to novel findings in the field of NBR and fairness. We find that Expected Exposure Loss (EEL) and Expected Exposure Disparity (EED) are the most robust and adaptable fairness metrics to be used in the NBR domain.
更多查看译文
关键词
Context-Aware Recommender Systems,Trust-Aware Recommender Systems
AI 理解论文
溯源树
样例
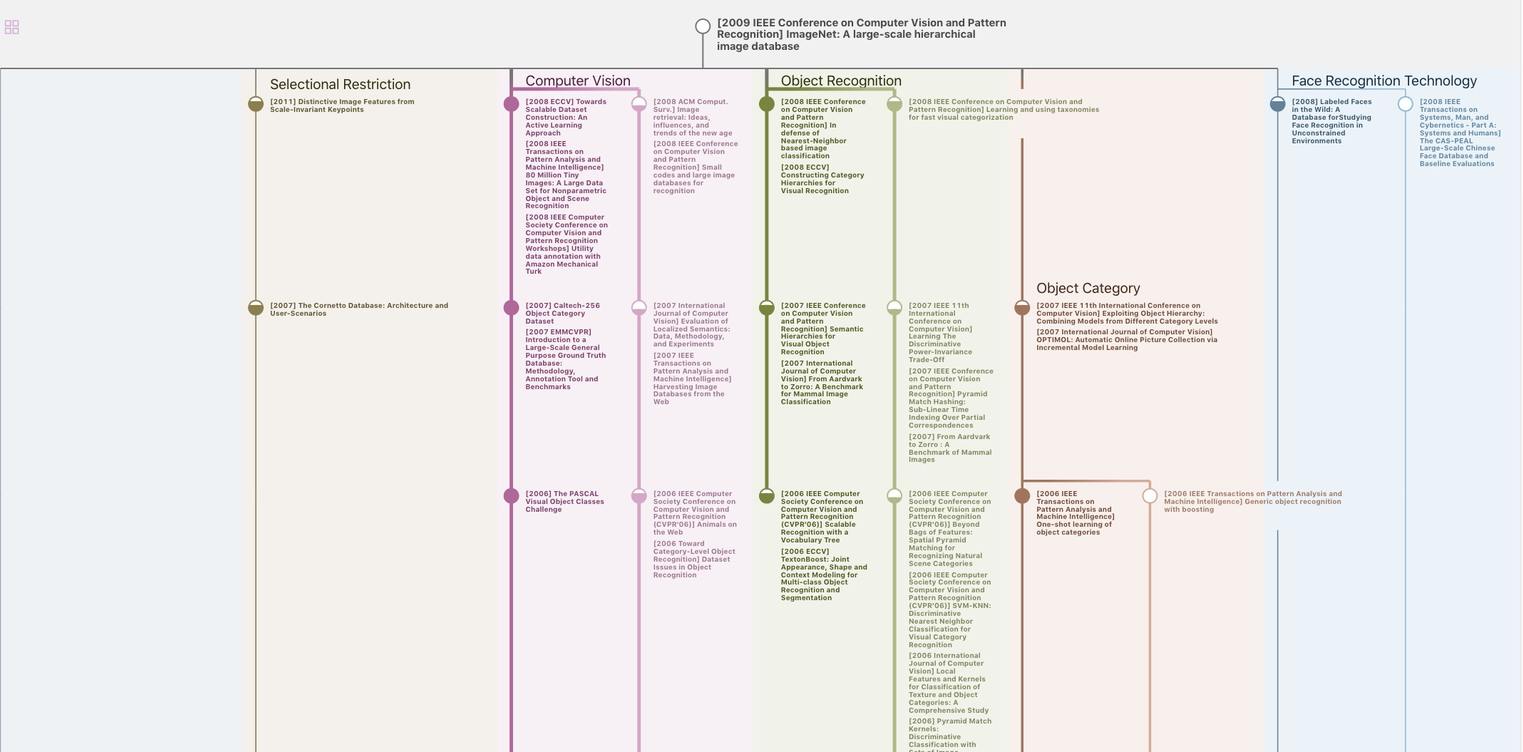
生成溯源树,研究论文发展脉络
Chat Paper
正在生成论文摘要